Identification of novel organic ferroelectrics: A study combining importance sampling with machine learning
arXiv (Cornell University)(2021)
摘要
Recent advances in the synthesis of polar molecular materials have produced practical alternatives to ferroelectric ceramics, opening up exciting new avenues for their incorporation into modern electronic devices. However, in order to realize the full potential of polar polymer and molecular crystals for modern technological applications, it is paramount to assemble and evaluate all the available data for such compounds, identifying descriptors that could be associated with an emergence of ferroelectricity. In this work, we utilized data-driven approaches to judiciously shortlist candidate materials from a wide chemical space that could possess ferroelectric functionalities. An importance-sampling based method was utilized to address the challenge of having a limited amount of available data on already known organic ferroelectrics. Sets of molecular- and crystal-level descriptors were combined with a Random Forest Regression algorithm in order to predict spontaneous polarization of the shortlisted compounds. First-principles simulations were performed to further validate the predictions obtained from the machine learning model.
更多查看译文
关键词
novel organic ferroelectrics,machine learning
AI 理解论文
溯源树
样例
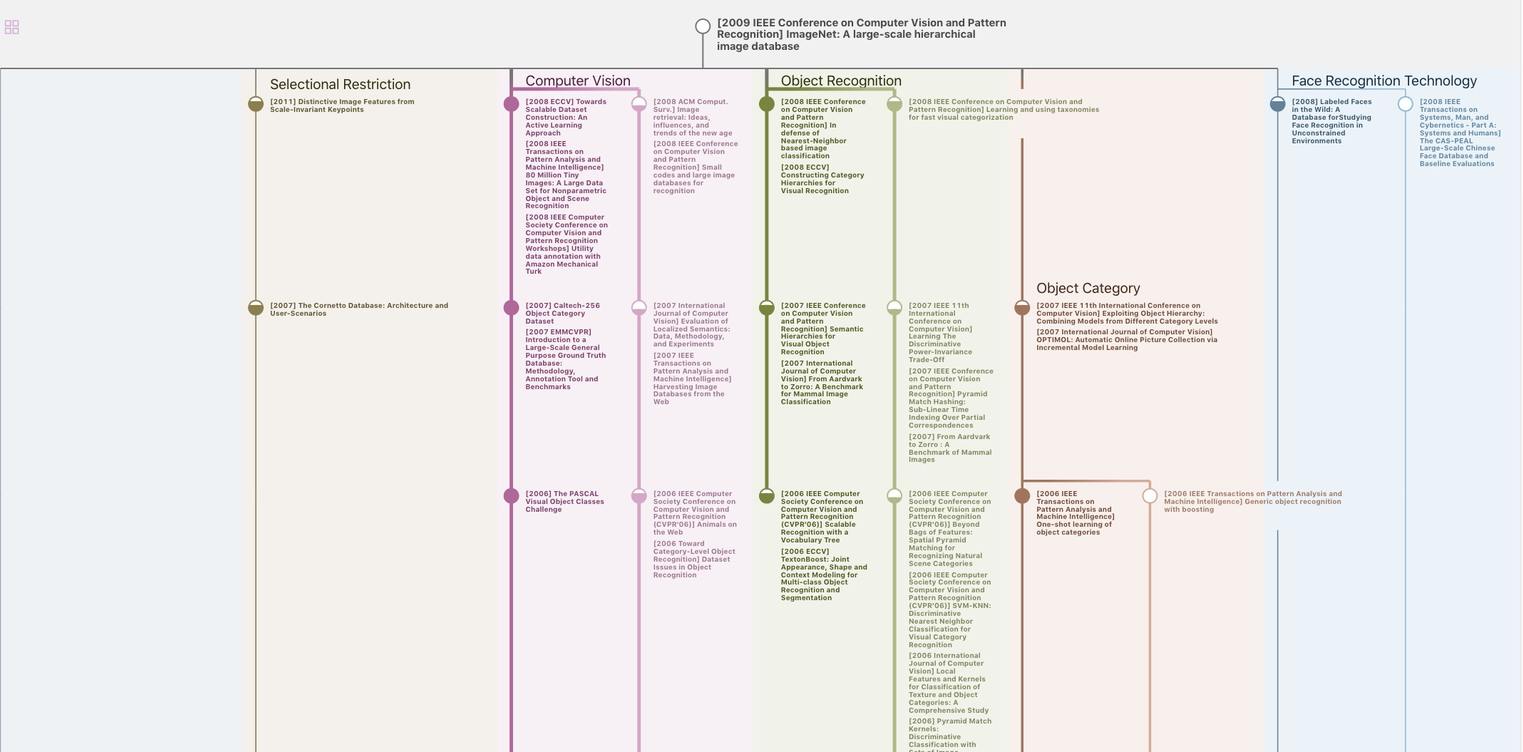
生成溯源树,研究论文发展脉络
Chat Paper
正在生成论文摘要