perCLTV: A General System for Personalized Customer Lifetime Value Prediction in Online Games
ACM Transactions on Information Systems (TOIS)(2022)
摘要
Online games make up the largest segment of the booming global game market, in terms of revenue as well as players. Unlike games that sell games at one time for profit, online games make money from in-game purchases by a large number of engaged players. Therefore, Customer Lifetime Value (CLTV) is particularly vital for game companies to improve marketing decisions and increase game revenues. Nowadays, as virtual game worlds are becoming increasingly innovative, complex, and diverse, the CLTV of massive players is highly personalized. That is, different players may have very different patterns of CLTV, especially on churn and payment. However, current solutions are inadequate in terms of personalization and thus limit predictive performance. First, most methods just attempt to address either task of CLTV, i.e., churn or payment, and only consider the personalization from one of them. Second, the correlation between churn and payment has not received enough attention and its personalization has not been fully explored yet. Last but not least, most solutions around this line are conducted based on historical data where the evaluation is not convincing enough without real-world tests. To tackle these problems, we propose a general system to predict personalized customer lifetime value in online games, named perCLTV. To be specific, we revisit the personalized CLTV prediction problem from the two sub-tasks of churn prediction and payment prediction in a sequential gated multi-task learning fashion. On this basis, we develop a generalized framework to model CLTV across games in distinct genres by heterogeneous player behavior data, including individual behavior sequential data and social behavior graph data. Comprehensive experiments on three real-world datasets validate the effectiveness and rationality of perCLTV, which significantly outperforms other baseline methods. Our work has been implemented and deployed in many online games released from NetEase Games. Online A/B testing in production shows that perCLTV achieves a prominent improvement in two precision marketing applications of popup recommendation and churn intervention.
更多查看译文
关键词
Personalized customer lifetime value,multi-task learning,churn prediction,payment prediction,online games
AI 理解论文
溯源树
样例
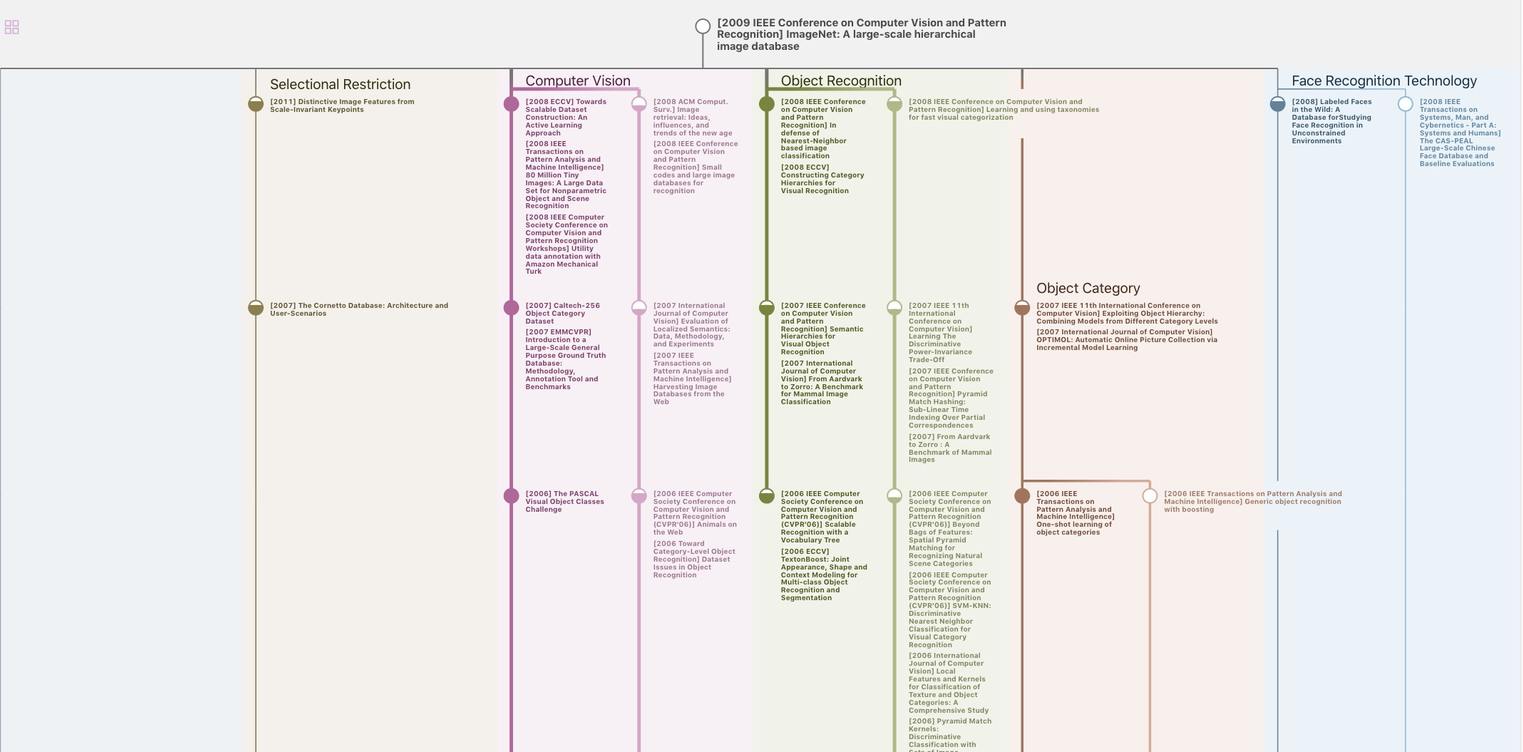
生成溯源树,研究论文发展脉络
Chat Paper
正在生成论文摘要