User Polarization Aware Matrix Factorization for Recommendation Systems
OHARS@RecSys(2021)
摘要
User feedback results in different rating patterns due to the users’ preferences, cognitive differences, and biases. However, little research has taken into account cognitive biases when building recommender systems. In this paper, we propose novel methods to take into account user polarization into matrix factorization-based recommendation systems, with the hope to produce algorithmic recommendations that are less biased by extreme polarization. Polarization is an emerging social phenomenon with serious consequences in the era of social media communication. Our experimental results show that our proposed methods outperform the widely-used methods while considering both rank-based and value-based evaluation metrics, as well as polarization-aware metrics.
更多查看译文
AI 理解论文
溯源树
样例
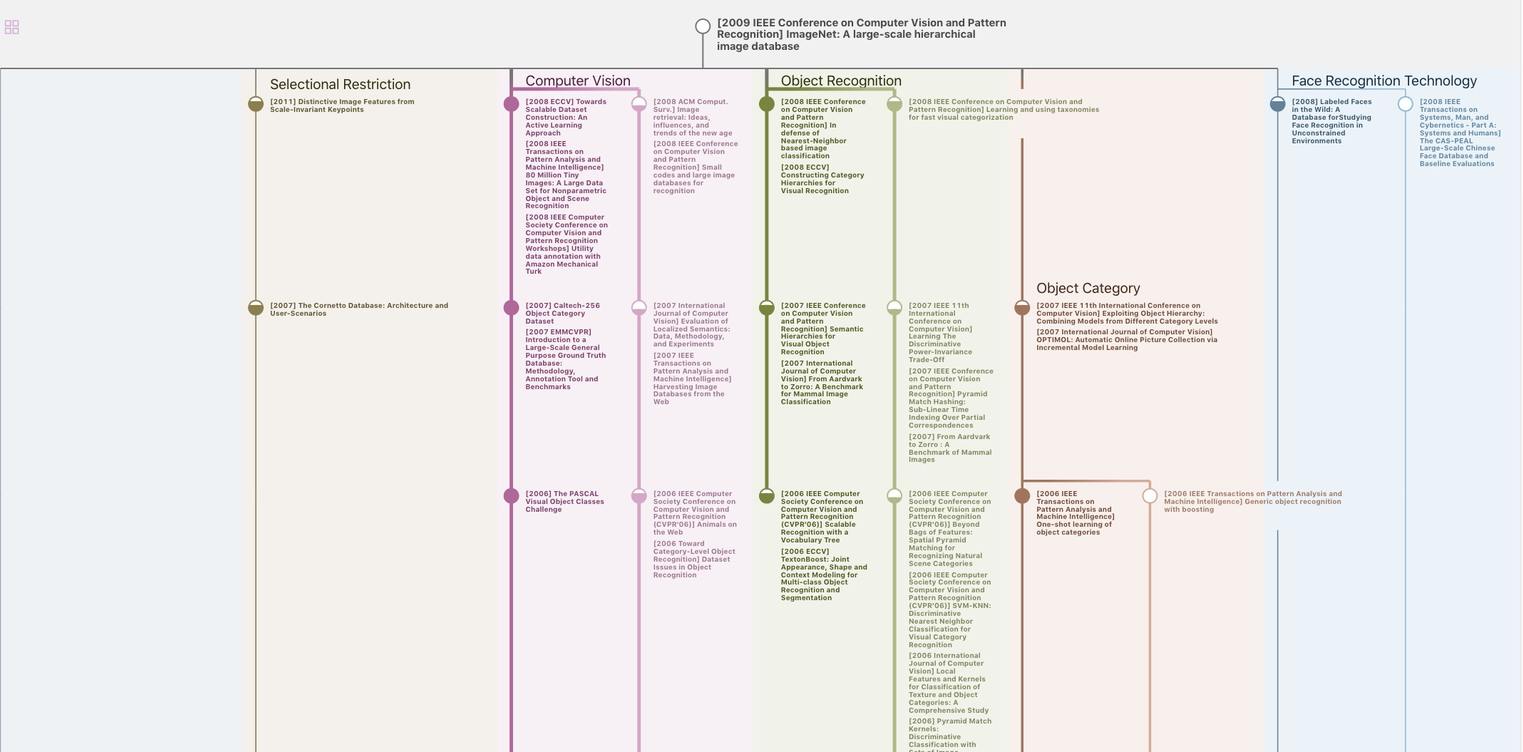
生成溯源树,研究论文发展脉络
Chat Paper
正在生成论文摘要