HowReinforcement Learning Systems Fail andWhat to do About It
semanticscholar(2022)
摘要
Recent research has turned to Reinforcement Learning (RL) to solve challenging decision problems, as an alternative to hand-tuned heuristics. RL can learn good policies without the need for modeling the environment’s dynamics. Despite this promise, RL remains an impractical solution for many realworld systems problems. A particularly challenging case occurs when the environment changes over time, i.e. it exhibits non-stationarity. In this work, we characterize the challenges introduced by non-stationarity and develop a framework for addressing themtotrainRLagents in livesystems.Suchagents must explore and learn new environments, without hurting the system’s performance, and remember them over time. To this end, our framework (1) identifies different environments encountered by the live system, (2) explores and trains a separate expert policy for each environment, and (3) employs safeguards to protect the system’s performance.We apply our framework to straggler mitigation, and evaluate it against a variety of alternative approaches using real-world. We show that each component of our framework is necessary to cope with non-stationarity. CCS Concepts: •Networks→Network algorithms; •Computingmethodologies→Reinforcement learning.
更多查看译文
AI 理解论文
溯源树
样例
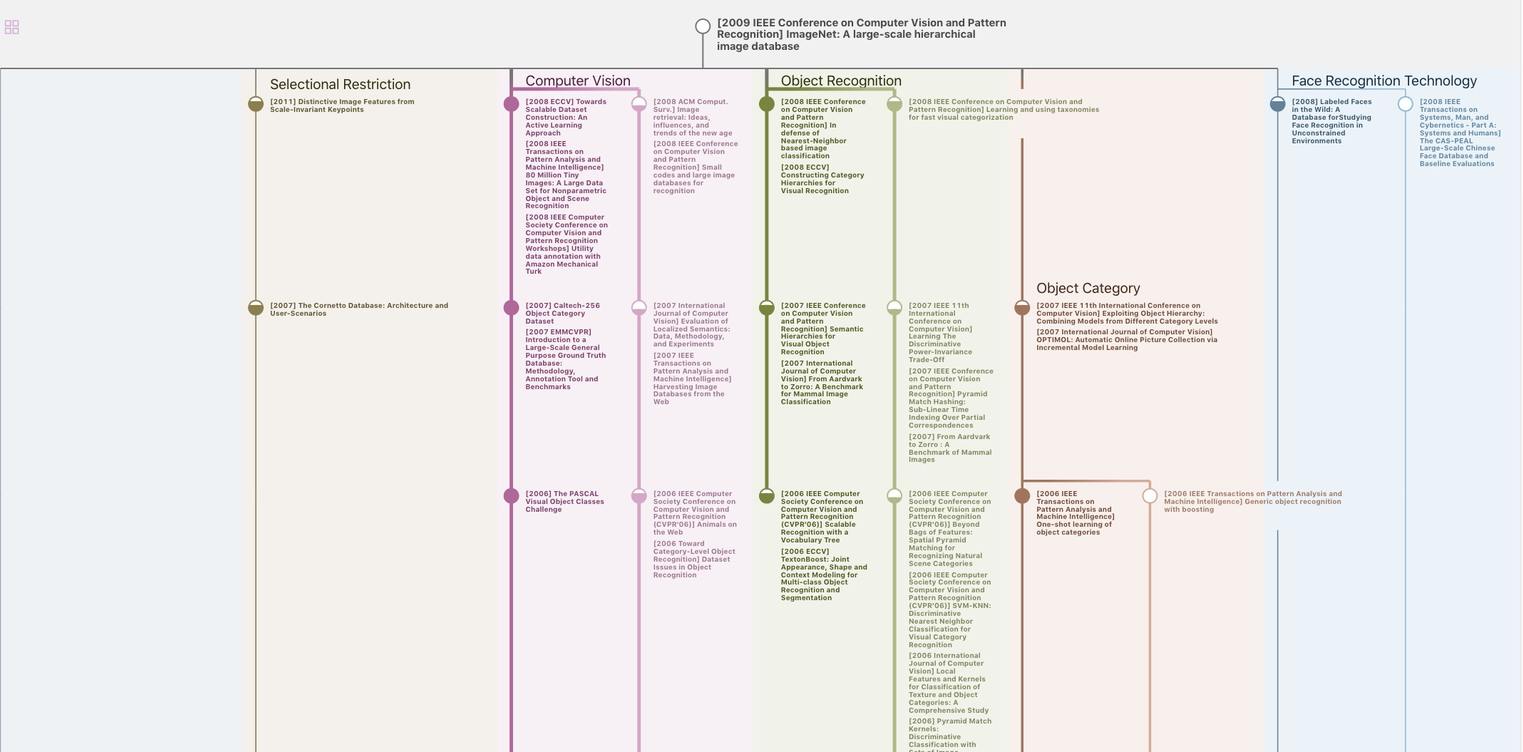
生成溯源树,研究论文发展脉络
Chat Paper
正在生成论文摘要