Symphony: Learning Realistic and Diverse Agents for Autonomous Driving Simulation
IEEE International Conference on Robotics and Automation(2022)
摘要
Simulation is a crucial tool for accelerating the development of autonomous vehicles. Making simulation realistic requires models of the human road users who interact with such cars. Such models can be obtained by applying learning from demonstration (LfD) to trajectories observed by cars already on the road. However, existing LfD methods are typically insufficient, yielding policies that frequently collide or drive off the road. To address this problem, we propose Symphony, which greatly improves realism by combining conventional policies with a parallel beam search. The beam search refines these policies on the fly by pruning branches that are unfavourably evaluated by a discriminator. However, it can also harm diversity, i.e., how well the agents cover the entire distribution of realistic behaviour, as pruning can encourage mode collapse. Symphony addresses this issue with a hierarchical approach, factoring agent behaviour into goal generation and goal conditioning. The use of such goals ensures that agent diversity neither disappears during adversarial training nor is pruned away by the beam search. Experiments on both proprietary and open Waymo datasets confirm that Symphony agents learn more realistic and diverse behaviour than several baselines.
更多查看译文
关键词
autonomous driving
AI 理解论文
溯源树
样例
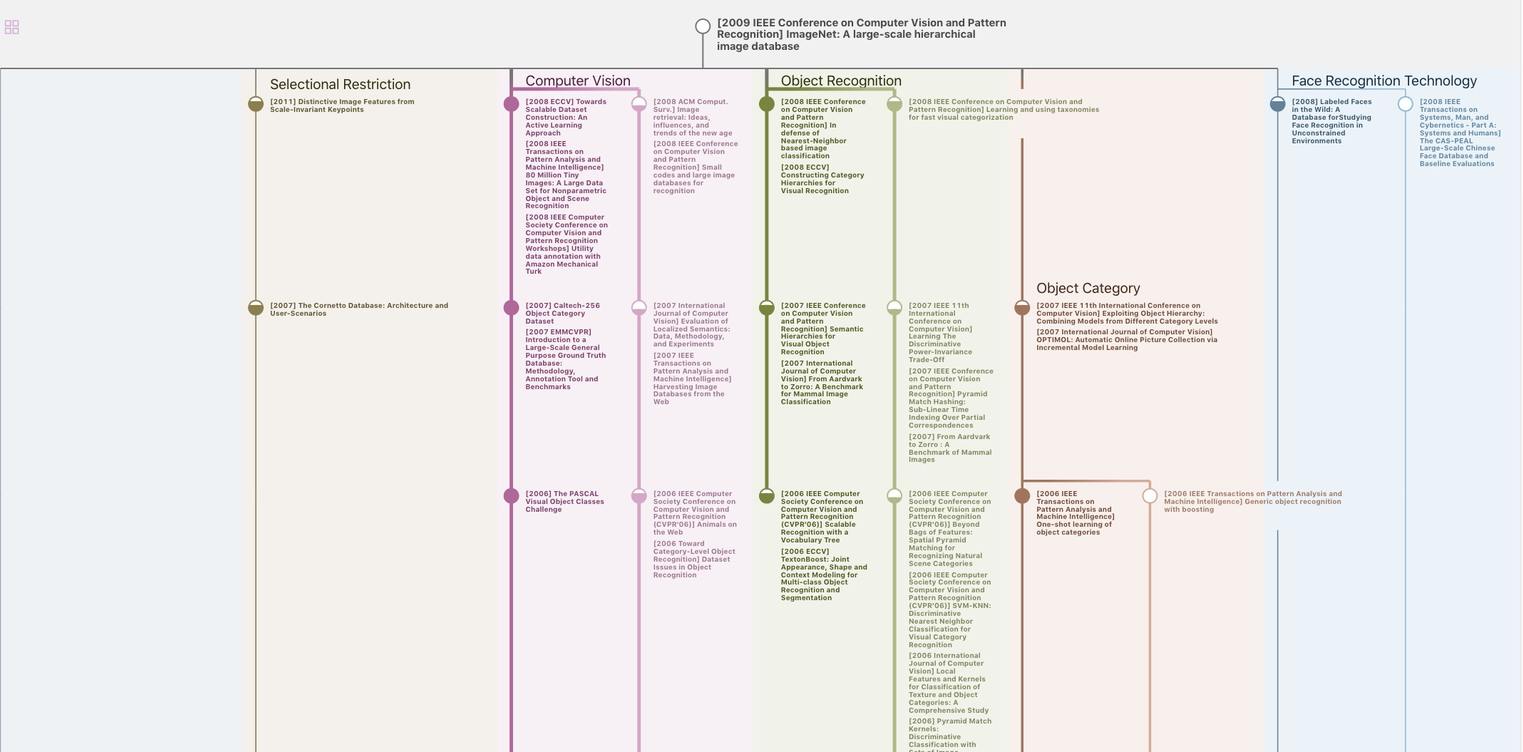
生成溯源树,研究论文发展脉络
Chat Paper
正在生成论文摘要