Efficient dynamic filter for robust and low computational feature extraction
arxiv(2022)
摘要
Unseen noise signal which is not considered in a model training process is difficult to anticipate and would lead to performance degradation. Various methods have been investigated to mitigate unseen noise. In our previous work, an Instance-level Dynamic Filter (IDF) and a Pixel Dynamic Filter (PDF) were proposed to extract noise-robust features. However, the performance of the dynamic filter might be degraded since simple feature pooling is used to reduce the computational resource in the IDF part. In this paper, we propose an efficient dynamic filter to enhance the performance of the dynamic filter. Instead of utilizing the simple feature mean, we separate Time-Frequency (T-F) features as non-overlapping chunks, and separable convolutions are carried out for each feature direction (inter chunks and intra chunks). Additionally, we propose Dynamic Attention Pooling that maps high dimensional features as low dimensional feature embeddings. These methods are applied to the IDF for keyword spotting and speaker verification tasks. We confirm that our proposed method performs better in unseen environments (unseen noise and unseen speakers) than state-of-the-art models.
更多查看译文
关键词
dynamic filter,keyword spotting,speaker verification
AI 理解论文
溯源树
样例
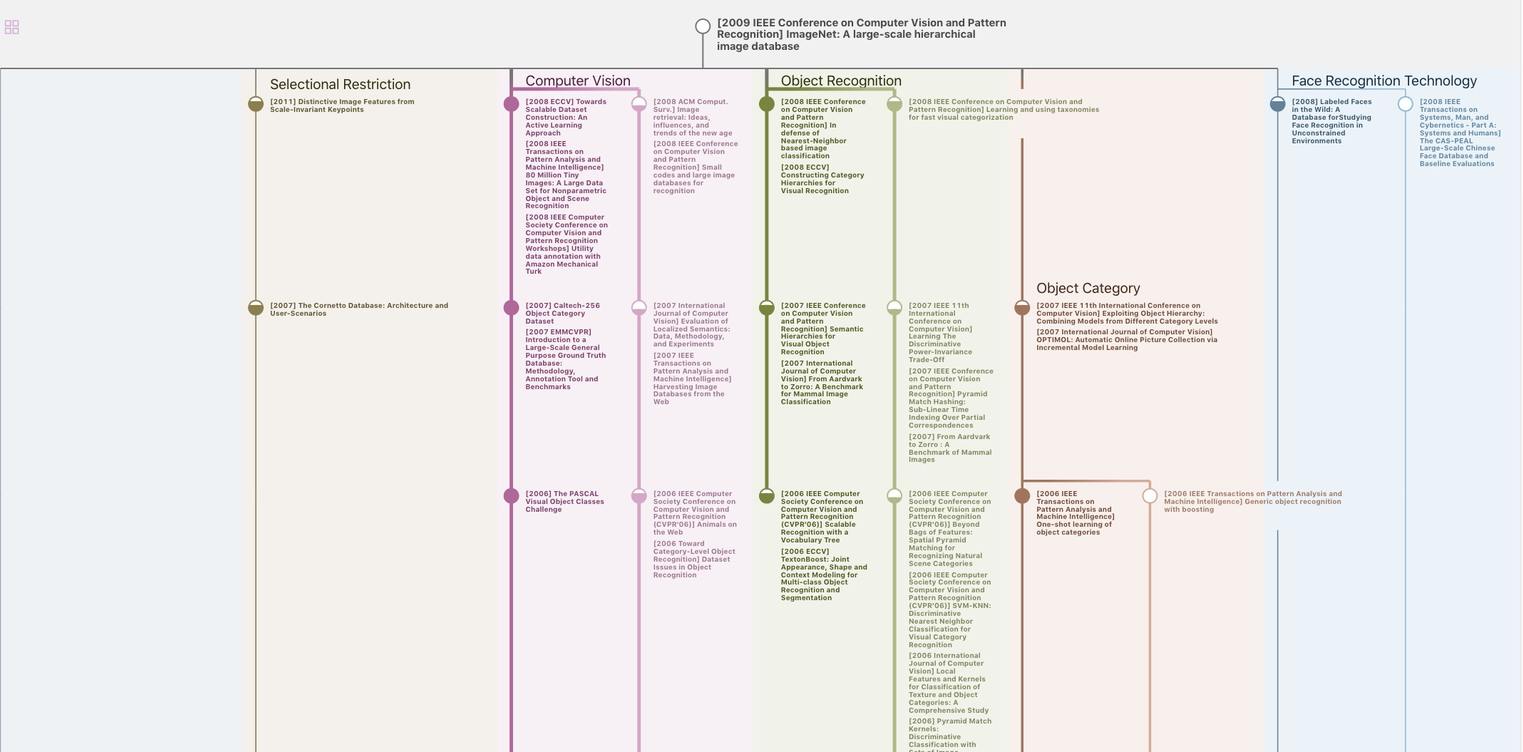
生成溯源树,研究论文发展脉络
Chat Paper
正在生成论文摘要