Model-Parallel Fourier Neural Operators as Learned Surrogates for Large-Scale Parametric PDEs
arxiv(2023)
摘要
Fourier neural operators (FNOs) are a recently introduced neural network architecture for learning solution operators of partial differential equations (PDEs), which have been shown to perform significantly better than comparable deep learning approaches. Once trained, FNOs can achieve speed-ups of multiple orders of magnitude over conventional numerical PDE solvers. However, due to the high dimensionality of their input data and network weights, FNOs have so far only been applied to two-dimensional or small three-dimensional problems. To remove this limited problem-size barrier, we propose a model-parallel version of FNOs based on domain-decomposition of both the input data and network weights. We demonstrate that our model-parallel FNO is able to predict time-varying PDE solutions of over 2.6 billion variables on Perlmutter using up to 512 A100 GPUs and show an example of training a distributed FNO on the Azure cloud for simulating multiphase CO$_2$ dynamics in the Earth's subsurface.
更多查看译文
关键词
Operator learning, Deep learning, Learned surrogates, Model parallelism, Multiphase flow, Carbon capture sequestration
AI 理解论文
溯源树
样例
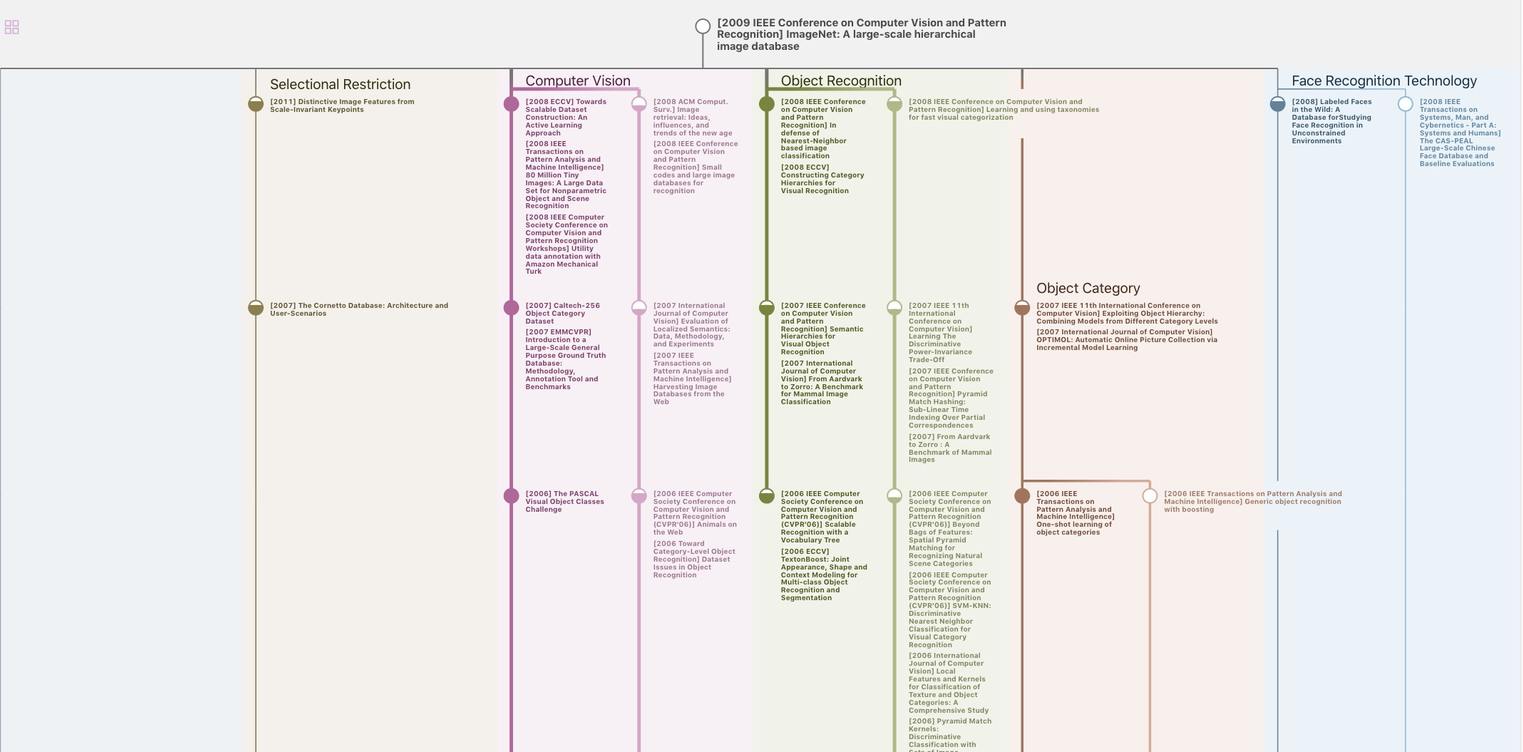
生成溯源树,研究论文发展脉络
Chat Paper
正在生成论文摘要