A framework for unbiased explainable pairwise ranking for recommendation
Software Impacts(2022)
摘要
Recent research in recommender systems has demonstrated the advantages of pairwise ranking in recommendation. In this work, we focus on the state-of-the-art pairwise ranking loss function, Bayesian Personalized Ranking (BPR), and aim to address two of its limitations, namely: (1) the lack of explainability and (2) exposure bias. We propose a recommendation framework that encompasses various loss functions that are based on BPR and which aim to mitigate the aforementioned limitations. Our open-source framework includes code to train and tune state-of-the-art pairwise ranking recommender systems on benchmark datasets and evaluate them based on the three criteria of ranking accuracy, explainability, and popularity debiasing.
更多查看译文
关键词
Recommender systems,Fairness in AI,Debiased machine learning,Pairwise ranking,Explainability,Exposure bias
AI 理解论文
溯源树
样例
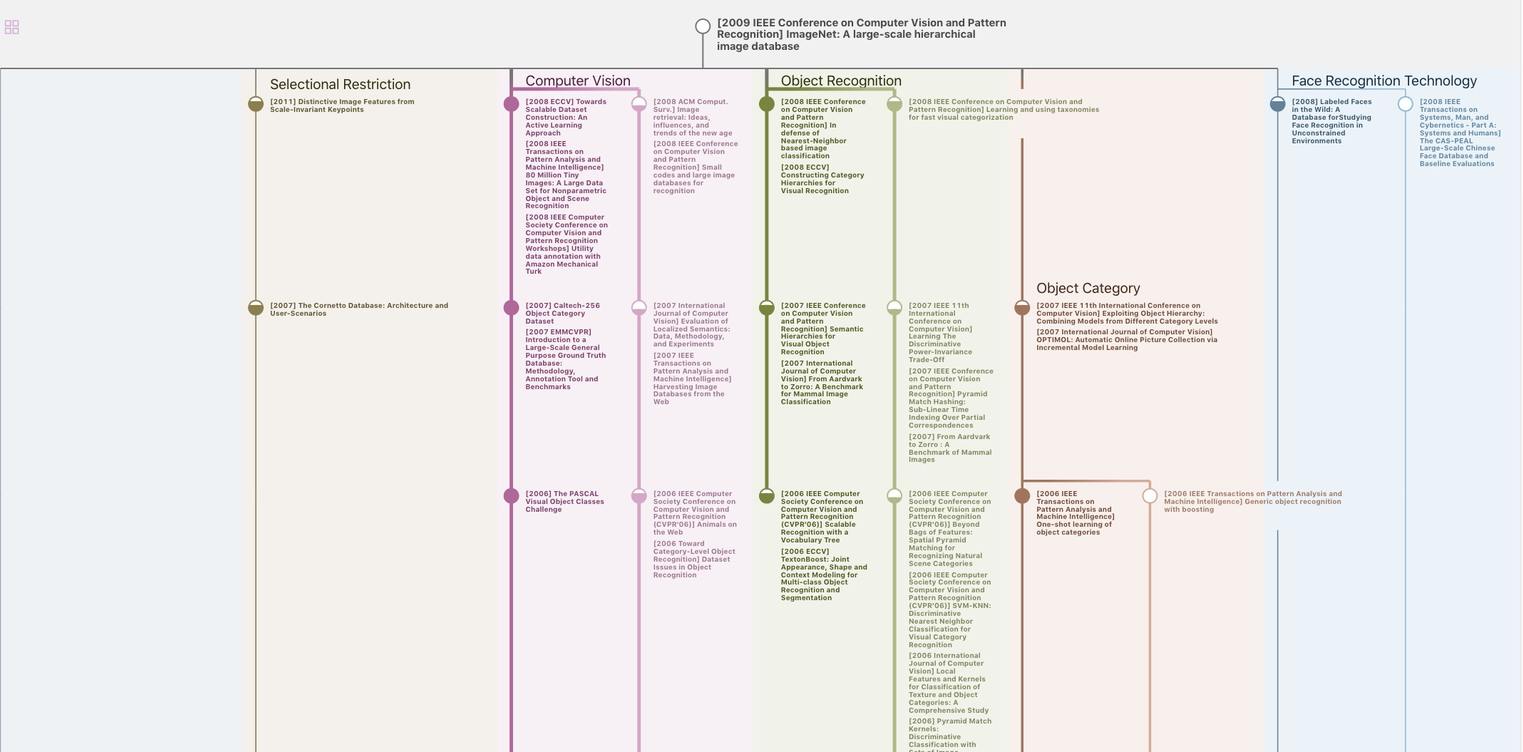
生成溯源树,研究论文发展脉络
Chat Paper
正在生成论文摘要