Extrinsic Self-Calibration of the Surround-View System: A Weakly Supervised Approach
IEEE Transactions on Multimedia(2023)
摘要
An SVS usually consists of four wide-angle fisheye cameras mounted around the vehicle to sense the surrounding environment. From the images synchronously captured by cameras, a top-down surround-view can be synthesized, on the premise that both intrinsics and extrinsics of the cameras have been calibrated. At present, the intrinsic calibration approach is relatively complete and can be pipelined, while the extrinsic calibration is still immature. To fill such a research gap, we propose a novel extrinsic self-calibration scheme which follows a weakly supervised framework, namely WESNet (Weakly-supervised Extrinsic Self-calibration Network). The training of WESNet consists of two stages. First, we utilize the corners in a few calibration site images as the weak supervision to roughly optimize the network by minimizing the geometric loss. Then, after the convergence in the first stage, we additionally introduce a self-supervised photometric loss term that can be constructed by the photometric information from natural images for further fine-tuning. Besides, to support training, we totally collected 19,078 groups of synchronously captured fisheye images under various environmental conditions. To our knowledge, thus far this is the largest surround-view dataset containing original fisheye images. By means of learning prior knowledge from the training data, WESNet takes the original fisheye images synchronously collected as the input, and directly yields extrinsics end-to-end with little labor cost. Its efficiency and efficacy have been corroborated by extensive experiments conducted on our collected dataset. To make our results reproducible, source code and the collected dataset have been released.(1)
更多查看译文
关键词
Extrinsic calibration, photometric loss, surround-view dataset, surround-view system, weakly supervised learning
AI 理解论文
溯源树
样例
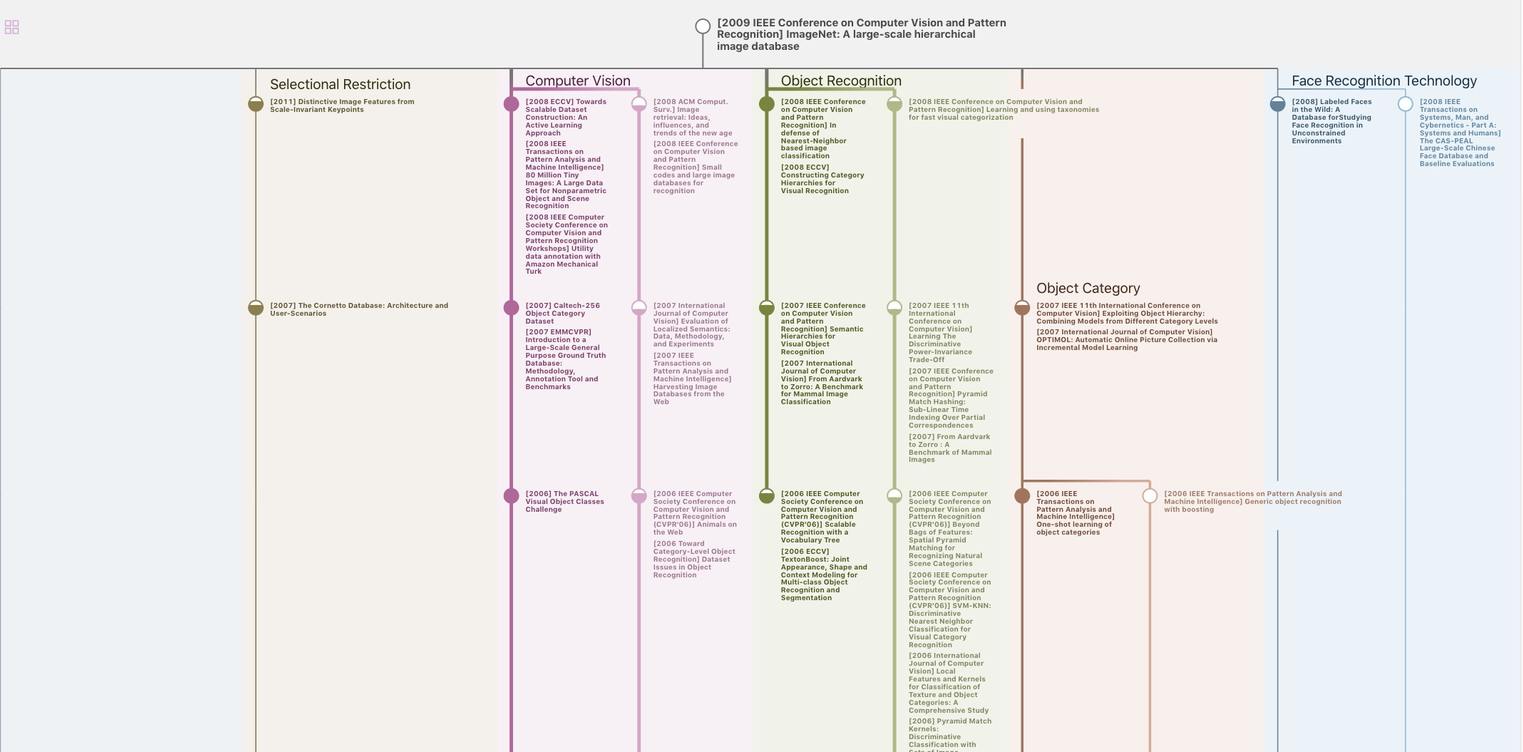
生成溯源树,研究论文发展脉络
Chat Paper
正在生成论文摘要