Generating targeted e-mail at scale using neural machine translation
2019 Workshop on DYnamic and Novel Advances in Machine learning and Intelligent Cyber Security(2019)
摘要
Advances in deep learning, specifically in Neural Machine Translation (NMT) [1, 20, 28], have opened up new application opportunities for generating human-readable text by machines. In this paper, we discuss one such application – the generation of spearphishing e-mail at scale – that has ramifications for cybersecurity. To this end, the ability to characterize text generated by people and by machines is important, and specific characteristics of text could be used as differentiating features. For example, we could evaluate whether the generated text is grammatically well-formed or semantically relevant to some topic. However, existing metrics for evaluating NMT models do not adequately address these needs. As a result, we propose new metrics that capture both well-formedness and topical relevance to evaluate the quality of text. We show that the proposed metrics corroborate the findings of existing metrics and provide additional insights. Although the metrics consistently indicate that text generated by state-of-the-art NMT models possess grammatical structure, the machine-generated text differ in performance with respect to the metrics when compared to human-generated text. By using these new metrics, we assessed whether the automatically-generated content would pass a spam/spearphishing filter [6]. The filter performs poorly in distinguishing between human-generated and machine-generated text indicating the robustness of existing models.
更多查看译文
AI 理解论文
溯源树
样例
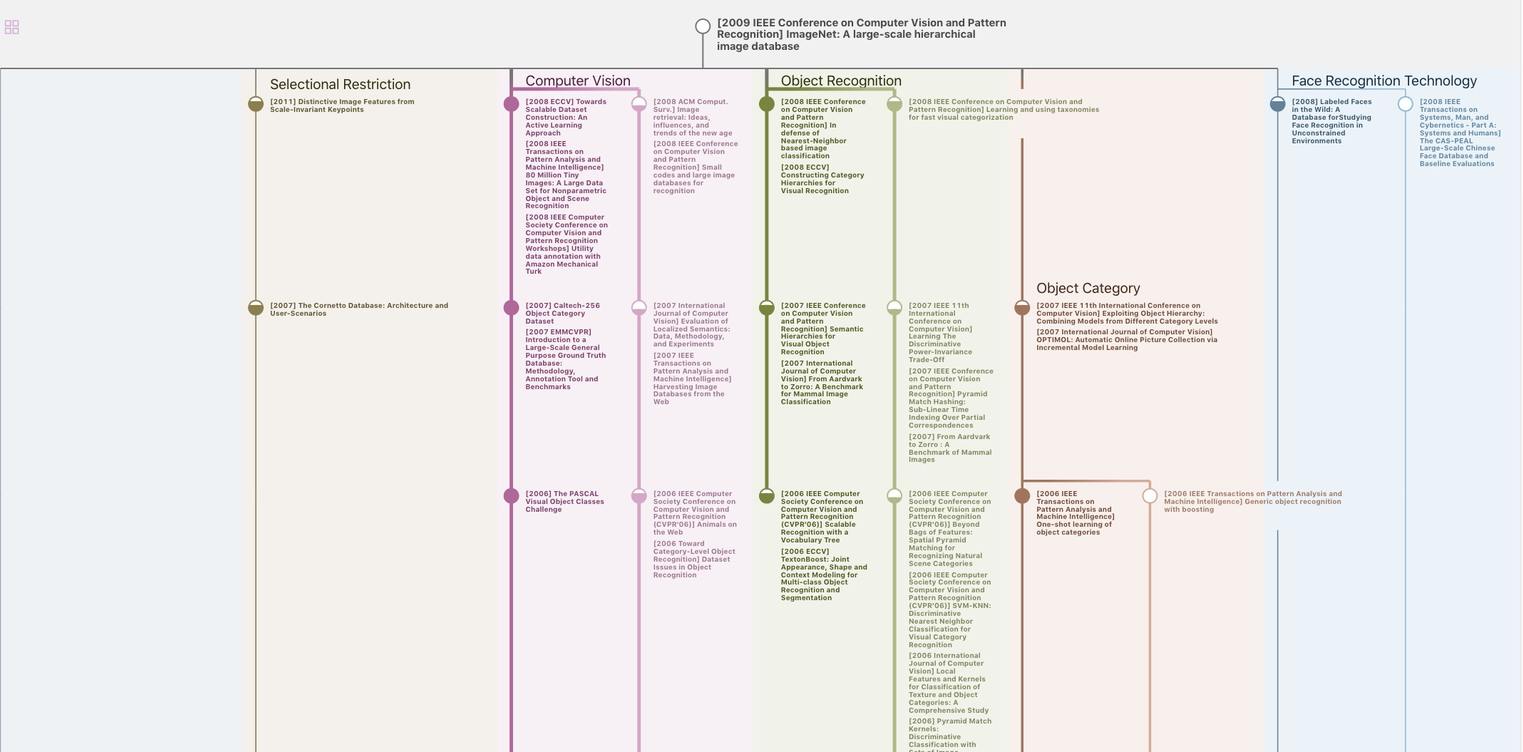
生成溯源树,研究论文发展脉络
Chat Paper
正在生成论文摘要