Learnable Maximum Amplitude Structure for Earthquake Event Classification
IEEE GEOSCIENCE AND REMOTE SENSING LETTERS(2022)
摘要
Recently, most research has been conducted to minimize damage from earthquakes by establishing an early warning system through the analysis of short seismic waves. In particular, deep learning is widely used as it allows to learn complex patterns for earthquake detection from seismic data without complex physical knowledge. In this letter, we propose an improved ConvNetQuake for earthquake event classification by adding learnable features related to the maximum amplitude of the seismic waveform. Since the maximum amplitude is a major factor representing the characteristics of an earthquake, we presented a deep learning structure that can apply this factor in the process of determining whether an earthquake occurs. In the proposed structure, the maximum amplitude is transformed into a feature learned through multi-layer perceptron (MLP) and then concatenates with features extracted through a convolutional neural network (CNN). On the STanford EArthquake Dataset (STEAD) dataset, the proposed method significantly increases the performance for an earthquake event classification than the previous state-of-the-art (SOTA) method by only adding a few parameters.
更多查看译文
关键词
Earthquakes,Feature extraction,Convolution,Biological system modeling,Geoscience and remote sensing,Deep learning,Training,Convolutional neural network (CNN),earthquake event classification,global maximum pooling (GMP),maximum amplitude,multi-layer perceptron (MLP)
AI 理解论文
溯源树
样例
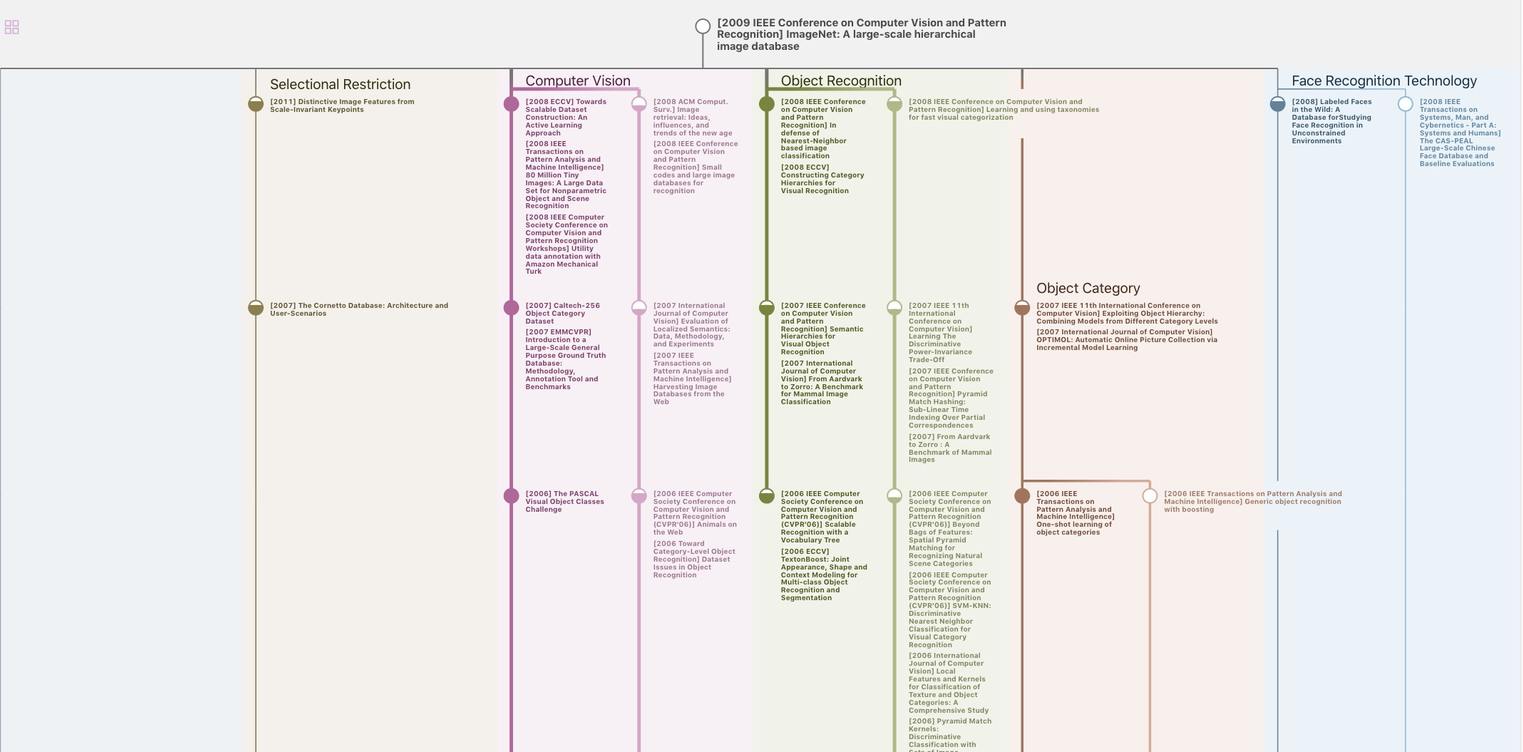
生成溯源树,研究论文发展脉络
Chat Paper
正在生成论文摘要