Barlow constrained optimization for Visual Question Answering
WACV(2023)
摘要
Visual question answering is a vision-and-language multi-modal task, that aims at predicting answers given samples from the question and image modalities. Most recent methods focus on learning a good joint embedding space of images and questions, either by improving the interaction between these two modalities, or by making it a more discriminant space. However, how informative this joint space is, has not been well explored. In this paper, we propose a novel regularization for VQA models, Constrained Optimization using Barlow's theory (COB), that improves the information content of the joint space by minimizing the redundancy. It reduces the correlation between the learned feature components and thereby disentangles semantic concepts. Our model also aligns the joint space with the answer embedding space, where we consider the answer and image+question as two different 'views' of what in essence is the same semantic information. We propose a constrained optimization policy to balance the categorical and redundancy minimization forces. When built on the state-of-the-art GGE model, the resulting model improves VQA accuracy by 1.4% and 4% on the VQA-CP v2 and VQA v2 datasets respectively. The model also exhibits better interpretability. Code is made available: https://github.com/abskjha/Barlow-constrained-VQA
更多查看译文
关键词
Algorithms: Vision + language and/or other modalities,Image recognition and understanding (object detection,categorization,segmentation,scene modeling,visual reasoning)
AI 理解论文
溯源树
样例
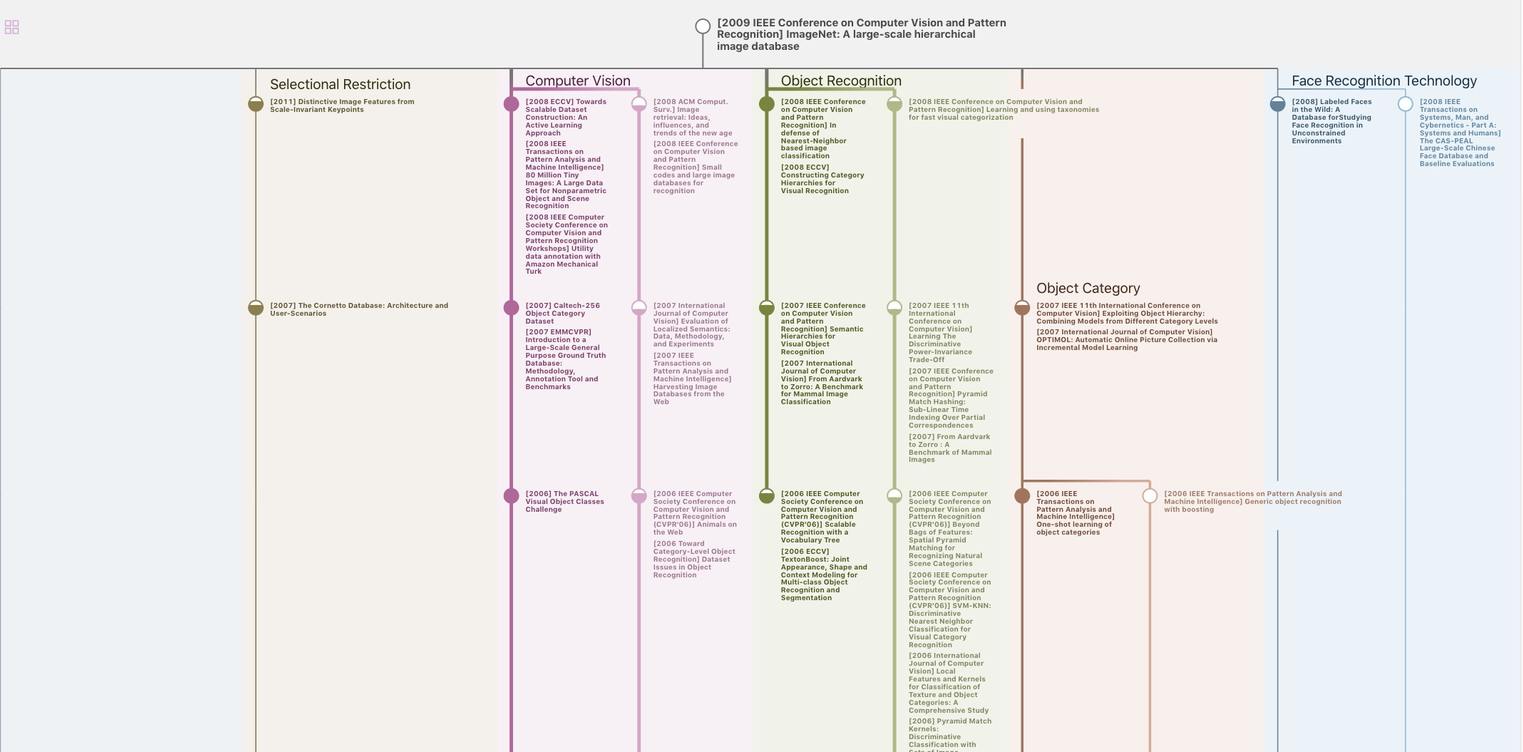
生成溯源树,研究论文发展脉络
Chat Paper
正在生成论文摘要