Algorithmic audits of algorithms, and the law
arxiv(2023)
摘要
Algorithmic decision-making is now widespread, ranging from health care allocation to more common actions such as recommendation or information ranking. The aim to audit these algorithms has grown alongside. In this article, we focus on external audits that are conducted by interacting with the user side of the target algorithm, and hence considered a black box. Yet, the legal framework in which these audits take place is mostly ambiguous to researchers developing them: on the one hand, the legal value of the audit outcome is uncertain; on the other hand, the auditors’ rights and obligations are unclear. The contribution of this article is to articulate two canonical audit forms to law, to shed light on these aspects: 1) the first audit form (we coin the Bobby audit form) checks a predicate against the algorithm, while the second (Sherlock) is looser and opens up to multiple investigations. We find that: Bobby audits are more amenable to prosecution, yet are delicate as operating on real user data. This can lead to rejection by a court (notion of admissibility). Sherlock audits craft data for their operation, most notably to build surrogates of the audited algorithm. It is mostly used for acts for whistleblowing , as even if accepted as proof, the evidential value will be low in practice. 2) these two forms require the prior respect of a proper right to audit, granted by law or by the platform being audited; otherwise, the auditor will be also prone to prosecutions regardless of the audit outcome. This article thus highlights the relation of current audits with law, to structure the growing field of algorithm auditing.
更多查看译文
关键词
Algorithmic decision-making,Machine learning,Audits,Law
AI 理解论文
溯源树
样例
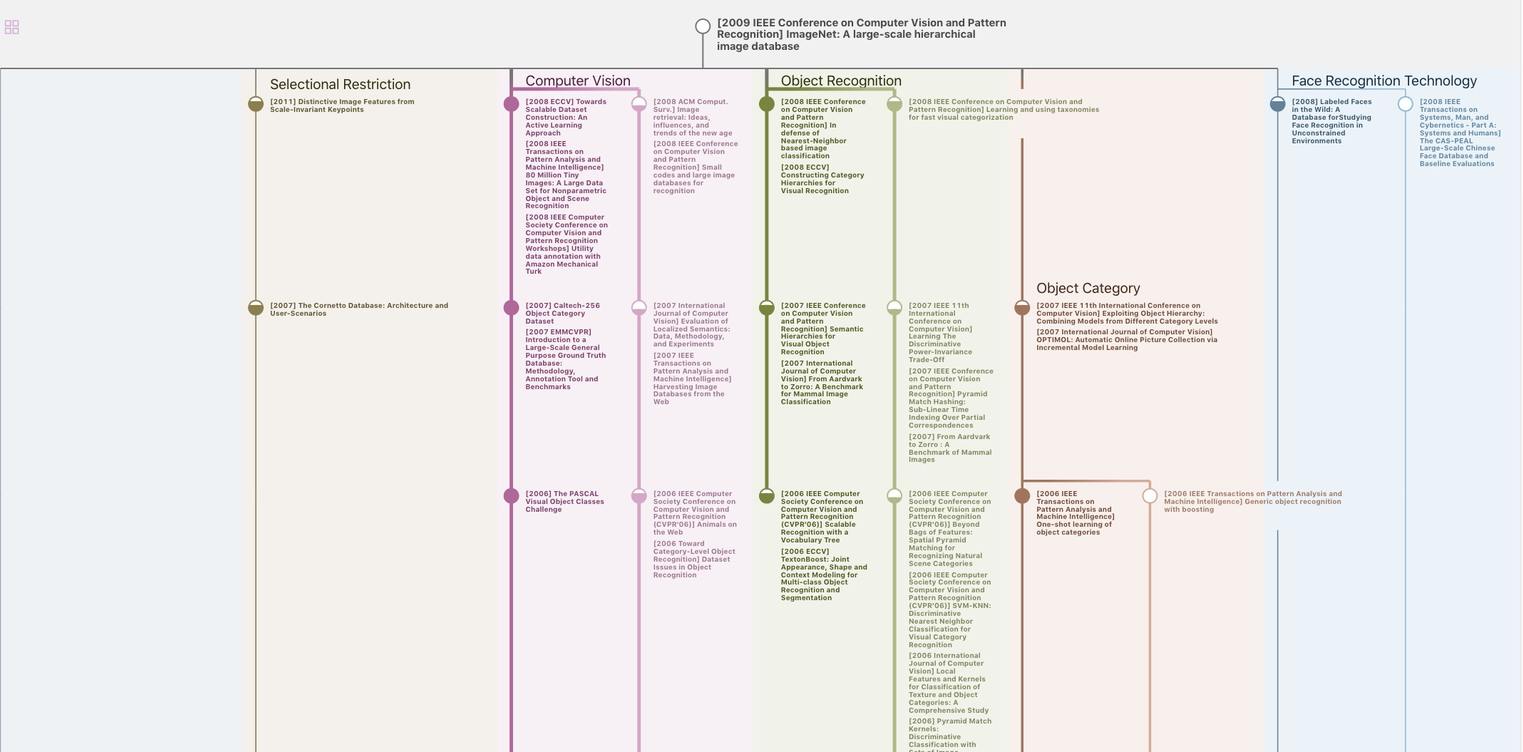
生成溯源树,研究论文发展脉络
Chat Paper
正在生成论文摘要