State space partitioning based on constrained spectral clustering for block particle filtering
SIGNAL PROCESSING(2022)
摘要
To overcome the curse of dimensionality of particle filter (PF), the block PF (BPF) partitions the state space into several blocks of smaller dimension so that the correction and resampling steps can be performed independently on each block. Despite its potential performance, this approach has a practical limitation. BPF requires an additional input compared to classical PF: the partition from which blocks are defined. A poor choice will not offer the expected performance gain. In this paper, we formulate the partitioning problem as a clustering problem and propose a data-driven partitioning method based on constrained spectral clustering (CSC) to automatically provide an appropriate partition. We design a generalized BPF that contains two new steps: (i) estimation of the state vector correlation matrix from particles, (ii) CSC using this estimate to determine blocks. The proposed method succeeds in providing an online partition into blocks restricted in size and grouping the most correlated state variables. This partition allows the BPF to escape the curse of dimensionality by reducing the variance of the filtering distribution estimate while limiting the level of bias. Since our approach relies on particles that are already necessary to generate as part of BPF, the computation overhead is limited. (C) 2022 Elsevier B.V. All rights reserved.
更多查看译文
关键词
Block particle filter,High dimensional models,State space partitioning,Constrained spectral clustering
AI 理解论文
溯源树
样例
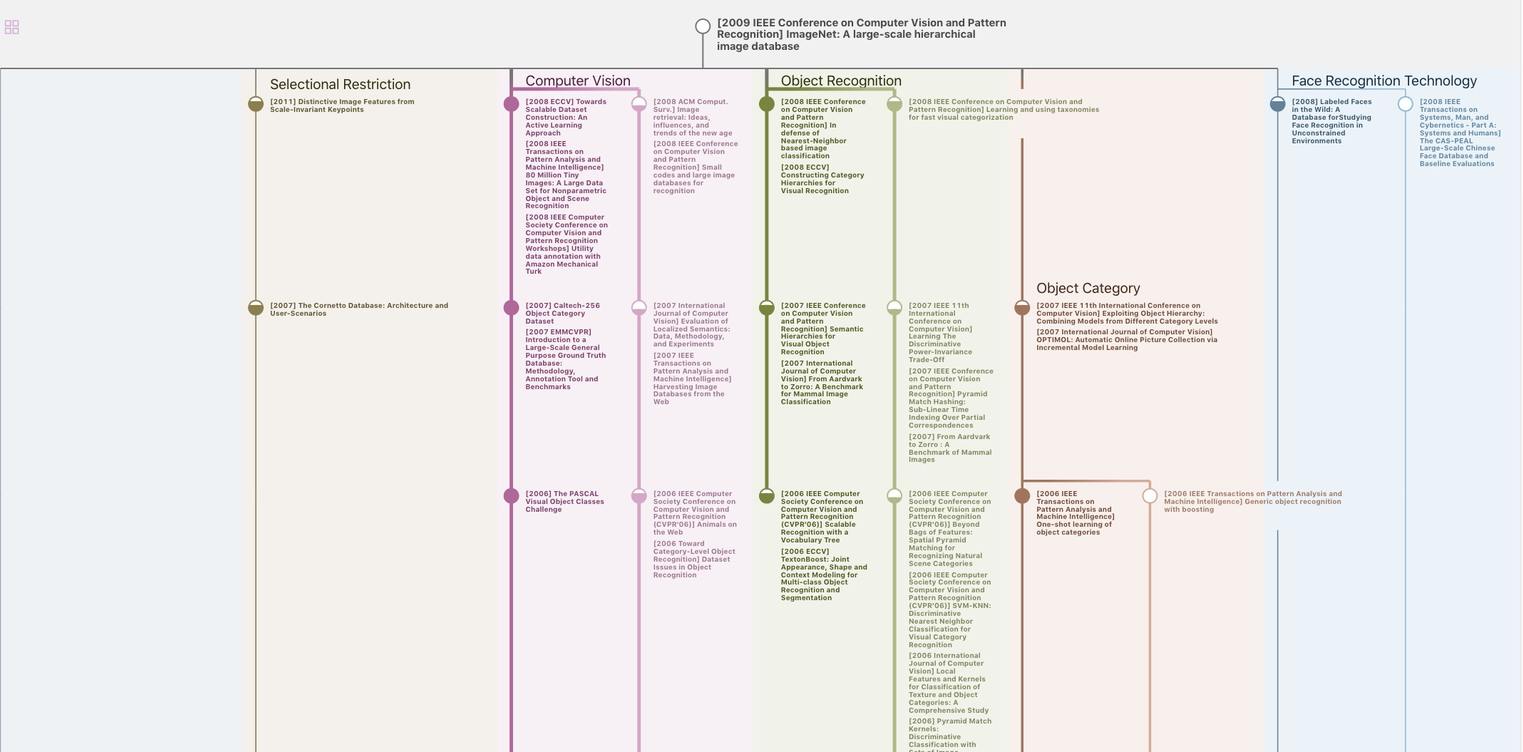
生成溯源树,研究论文发展脉络
Chat Paper
正在生成论文摘要