Bilateral Filtering Graph Convolutional Network for Multi-relational Social Recommendation in the Power-law Networks
ACM Transactions on Information Systems(2022)
摘要
AbstractIn recent years, advances in Graph Convolutional Networks (GCNs) have given new insights into the development of social recommendation. However, many existing GCN-based social recommendation methods often directly apply GCN to capture user-item and user-user interactions, which probably have two main limitations: (a) Due to the power-law property of the degree distribution, the vanilla GCN with static normalized adjacency matrix has limitations in learning node representations, especially for the long-tail nodes; (b) multi-typed social relationships between users that are ubiquitous in the real world are rarely considered. In this article, we propose a novel Bilateral Filtering Heterogeneous Attention Network (BFHAN), which improves long-tail node representations and leverages multi-typed social relationships between user nodes. First, we propose a novel graph convolutional filter for the user-item bipartite network and extend it to the user-user homogeneous network. Further, we theoretically analyze the correlation between the convergence values of different graph convolutional filters and node degrees after stacking multiple layers. Second, we model multi-relational social interactions between users as the multiplex network and further propose a multiplex attention network to capture distinctive inter-layer influences for user representations. Last but not least, the experimental results demonstrate that our proposed method outperforms several state-of-the-art GCN-based methods for social recommendation tasks.
更多查看译文
关键词
Social recommendation, power-law networks, multi-typed social relationships, heterogeneous graph neural network
AI 理解论文
溯源树
样例
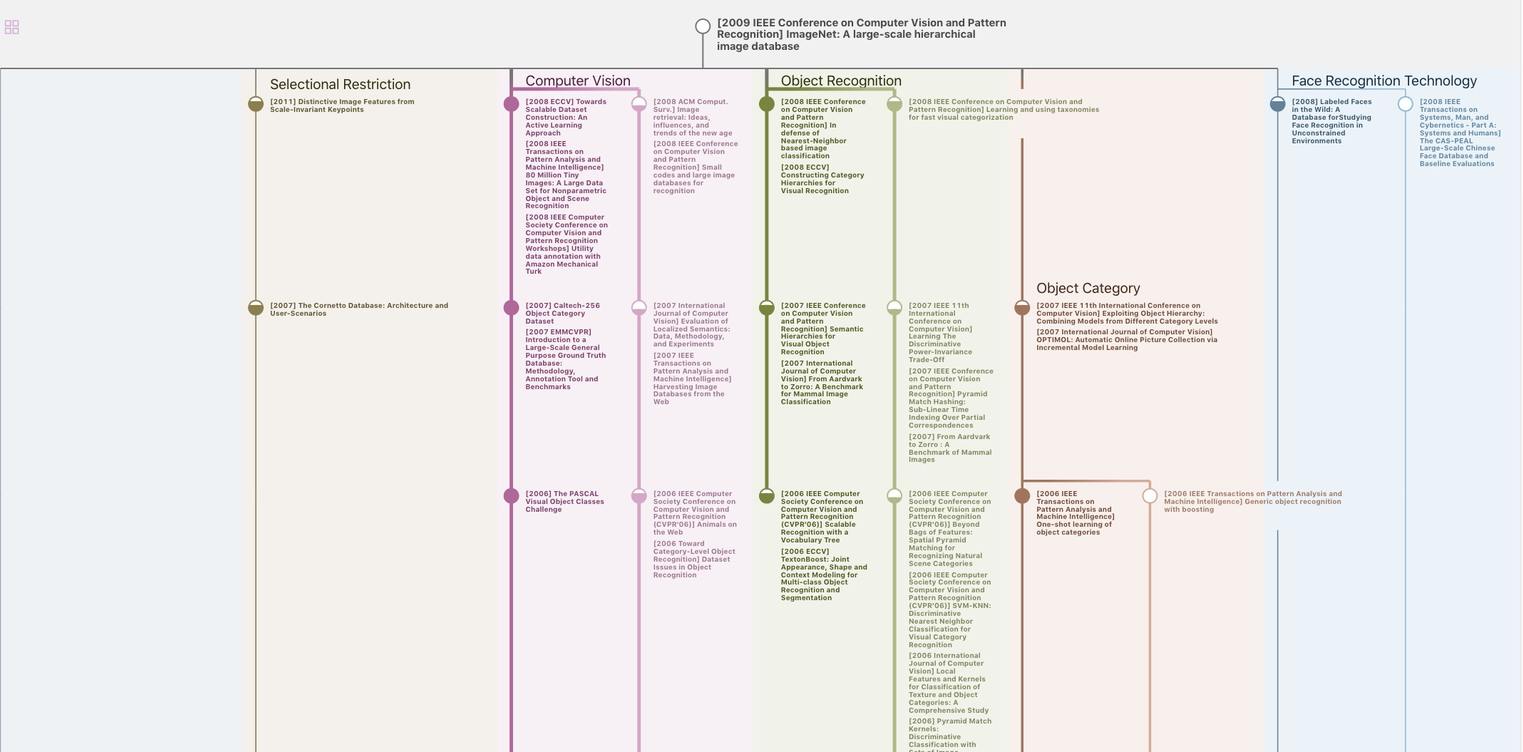
生成溯源树,研究论文发展脉络
Chat Paper
正在生成论文摘要