Trading-off Information Modalities in Zero-shot Classification
2022 IEEE WINTER CONFERENCE ON APPLICATIONS OF COMPUTER VISION (WACV 2022)(2022)
摘要
Zero-shot classification is the task of learning predictors for classes not seen during training. A practical way to deal with the lack of annotations for the target categories is to encode not only the inputs (images) but also the outputs (object classes) into a suitable representation space. We can use these representations to measure the degree at which images and categories agree by fitting a compatibility measure using the information available during training. One way to define such a measure is by a two step process in which we first project the elements of either space (visual or semantic) onto the other and then compute a similarity score in the target space. Although projections onto the visual space has shown better general performance, little attention has been paid to the degree at which the visual and semantic information contribute to the final predictions. In this paper, we build on this observation and propose two different formulations that allow us to explicitly trade-off the relative importance of the visual and semantic spaces for classification in a zero-shot setting. Our formulations are based on redefinition of the similarity scoring and loss function used to learn the projections. Experiments on six different datasets show that our approach lead to improve performance compared to similar methods. Moreover; combined with synthetic features, our approach competes favorably with the state of the art on both the standard and generalized settings.
更多查看译文
关键词
Transfer,Few-shot,Semi- and Un- supervised Learning Object Detection/Recognition/Categorization
AI 理解论文
溯源树
样例
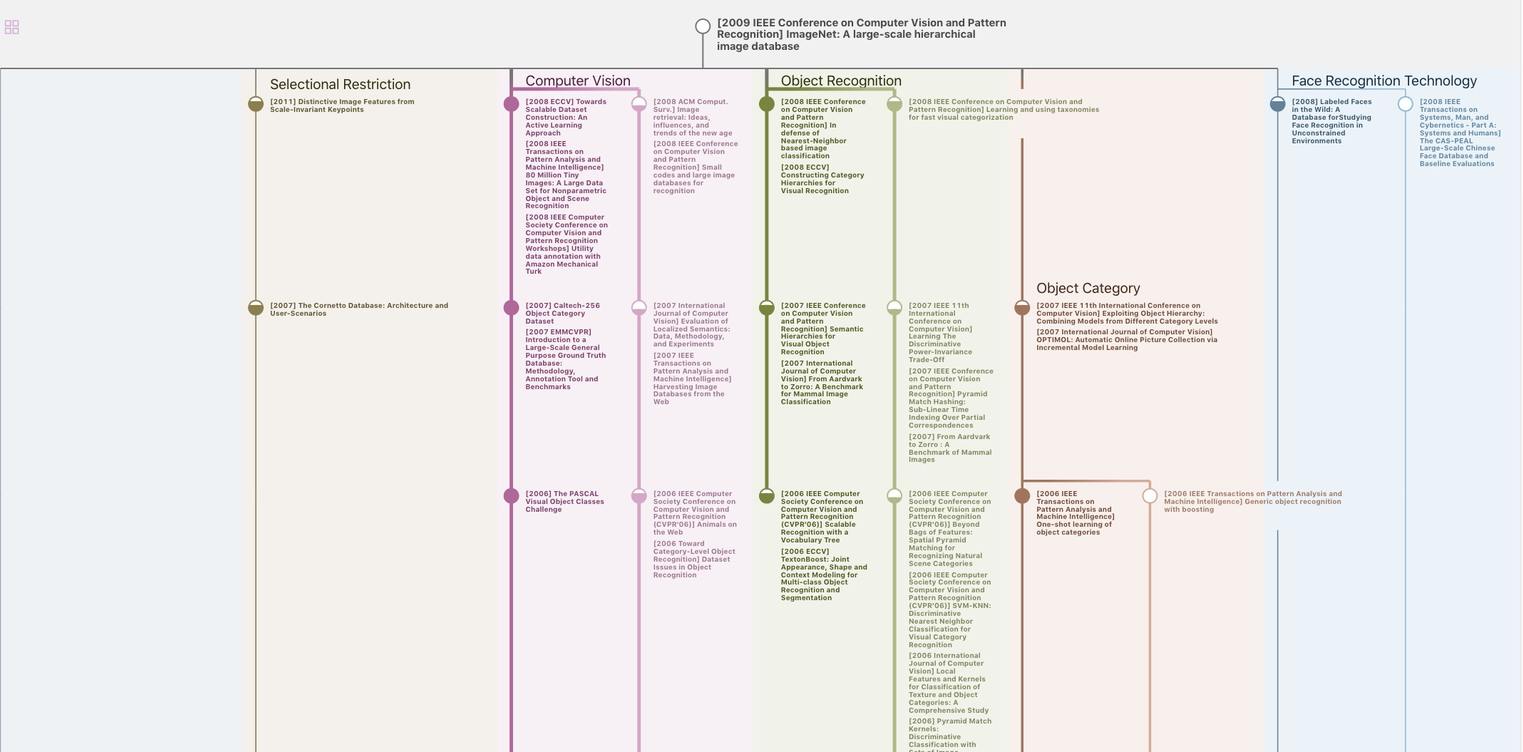
生成溯源树,研究论文发展脉络
Chat Paper
正在生成论文摘要