ChemTab: A Physics Guided Chemistry Modeling Framework
COMPUTATIONAL SCIENCE - ICCS 2022, PT I(2022)
摘要
Modeling of turbulent combustion system requires modeling the underlying chemistry and the turbulent flow. Solving both systems simultaneously is computationally prohibitive. Instead, given the difference in scales at which the two sub-systems evolve, the two sub- systems are typically (re)solved separately. Popular approaches such as the Flamelet Generated Manifolds (FGM) use a two-step strategy where the governing reaction kinetics are pre-computed and mapped to a low-dimensional manifold, characterized by a few reaction progress variables (model reduction) and the manifold is then "looked-up" during the run- time to estimate the high-dimensional system state by the flow system. While existing works have focused on these two steps independently, we show that joint learning of the progress variables and the look-up model, can yield more accurate results. We propose ChemTab an architecture that learns jointly and demonstrate its superiority.
更多查看译文
关键词
Physics guided neural networks, DNN
AI 理解论文
溯源树
样例
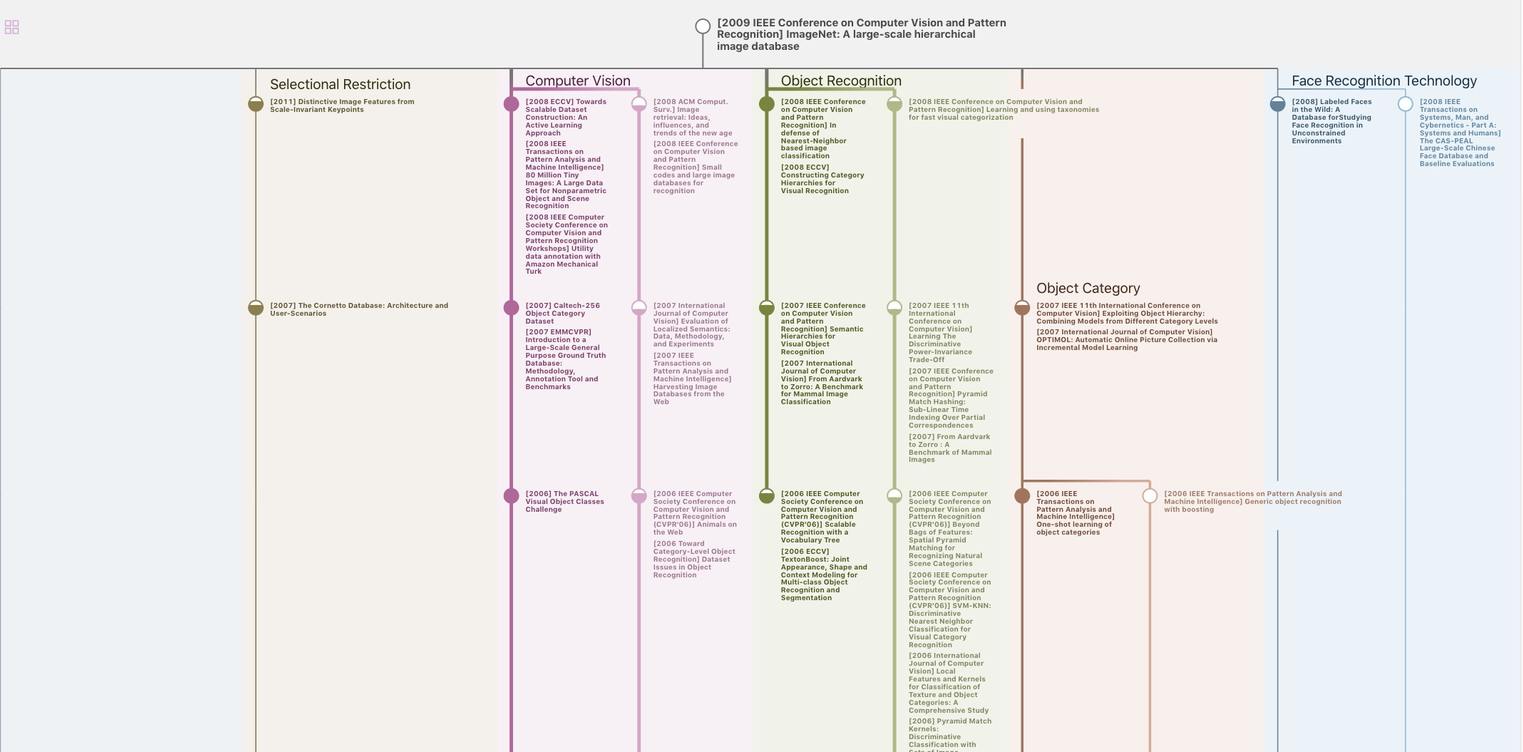
生成溯源树,研究论文发展脉络
Chat Paper
正在生成论文摘要