Reinforcement based Communication Topology Construction for Decentralized Learning with Non-IID Data
2021 IEEE GLOBAL COMMUNICATIONS CONFERENCE (GLOBECOM)(2021)
摘要
Federated Learning (FL) allows Internet-of-Things (IoT) devices to train a global model collaboratively and circumvent the security issue. However, the current FL framework has three main drawbacks, the huge network overhead, single point of failure, and accuracy degradation in non-independent-and-identically-distributed (non-IID) data distribution. We propose a novel Deep Reinforcement Learning (DRL) based Decentralized Learning (DL) framework, Deep Select, to 1) reduce the network overhead of conventional FL, 2) construct a good communication topology adaptively to mitigate the effect of non-IID data, and 3) accelerate the DL training by balancing the effects of hitting time (HT) and data bias. Moreover, DeepSelect with a subtly-designed DRL agent is reusable with different levels of non-IID data distributions. To the best of our knowledge, this paper is the first one to indicate that proper neighbor selection for exchanging parameters (not raw data) can counterbalance the data bias's effect and improve the DL convergence with non-IID data. The experiment results show that DeepSelect can reduce 18%-51% training rounds than the other heuristics on FashionMNIST and CIFAR-10 with non-IID data distributions.
更多查看译文
关键词
Deep Reinforcement Learning, Decentralized Learning, Communication Topology, Federated Learning
AI 理解论文
溯源树
样例
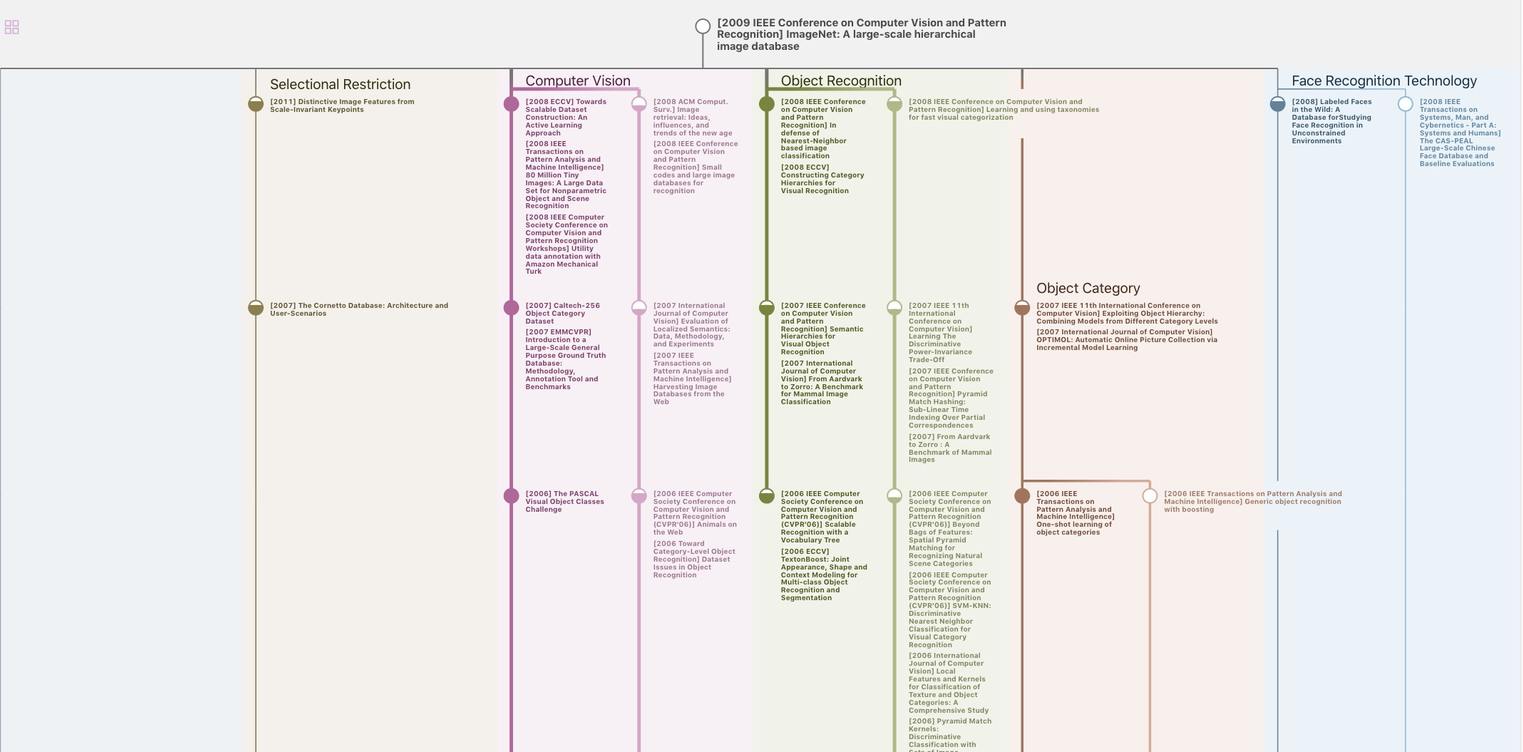
生成溯源树,研究论文发展脉络
Chat Paper
正在生成论文摘要