Distributed DRL-based Resource Allocation for Multicast D2D Communications
2021 IEEE GLOBAL COMMUNICATIONS CONFERENCE (GLOBECOM)(2021)
摘要
Device-to-device (D2D) communication is one of the promising solutions to improve spectrum efficiency and alleviate the mobile traffic explosion. However, interference mitigation and resource allocation in the underlying cellular network is a challenging task. In this paper, we propose a distributed deep reinforcement learning (DRL) based scheme to solve the interference mitigation and resource allocation problem. According to the channel status, each cellular user (CU) and D2D transmitter (D2D TX) will determine the appropriate reused channel and transmit power to maximize the system throughput. We propose a distributed DRL scheme and integrate two hotbooting algorithms into the scheme to improve the system throughput at the early stage of training. Simulation results show that the proposed distributed DRL with hotbooting outperforms the baselines regarding running time, message overhead, and throughput.
更多查看译文
关键词
hotbooting algorithms,D2D transmitter,CU,distributed DRL-based resource allocation scheme,cellular network,D2D TX,cellular user,resource allocation problem,distributed deep reinforcement learning based scheme,interference mitigation,mobile traffic explosion,spectrum efficiency,device-to-device communication,multicast D2D communications
AI 理解论文
溯源树
样例
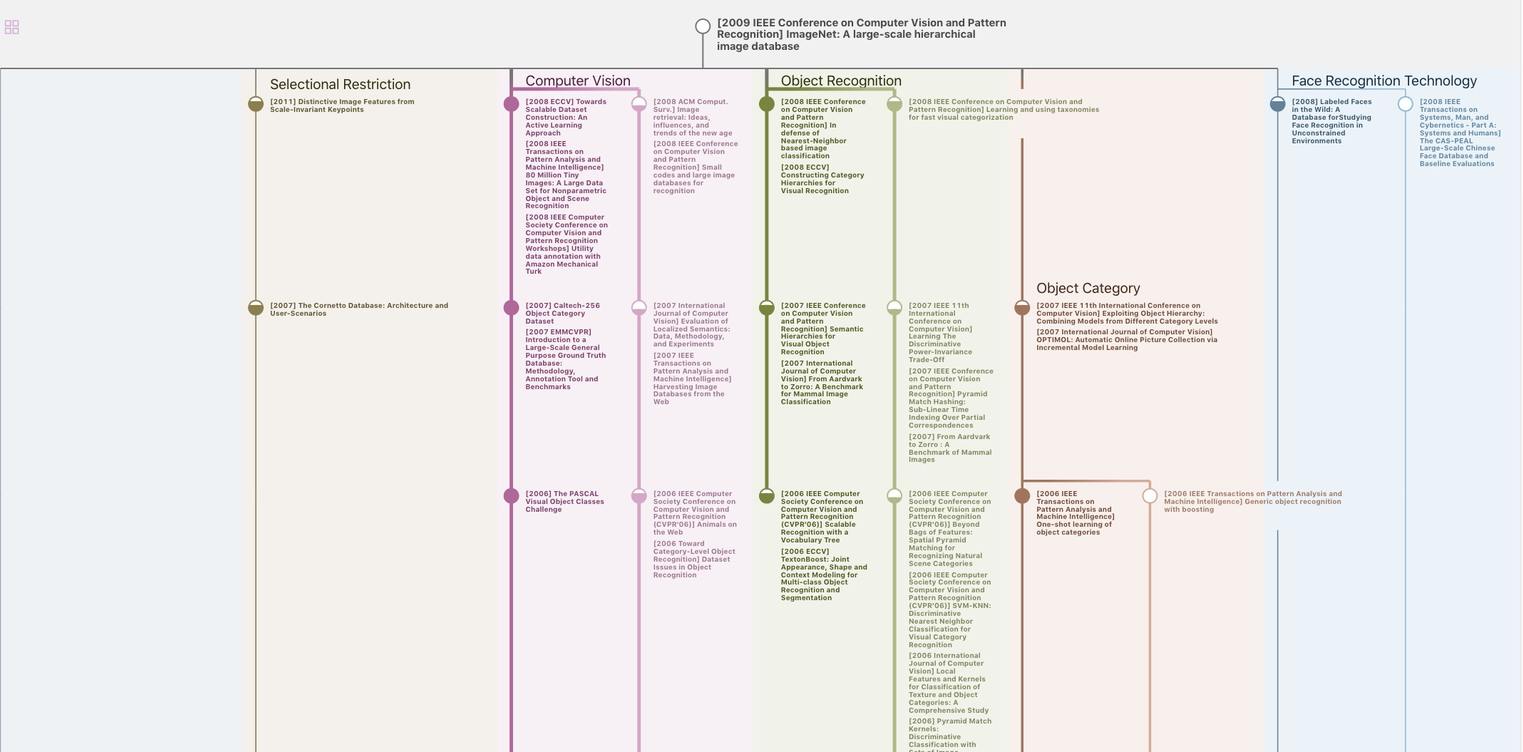
生成溯源树,研究论文发展脉络
Chat Paper
正在生成论文摘要