Defending against Reconstruction Attacks with R\'enyi Differential Privacy
arxiv(2022)
摘要
Reconstruction attacks allow an adversary to regenerate data samples of the training set using access to only a trained model. It has been recently shown that simple heuristics can reconstruct data samples from language models, making this threat scenario an important aspect of model release. Differential privacy is a known solution to such attacks, but is often used with a relatively large privacy budget (epsilon > 8) which does not translate to meaningful guarantees. In this paper we show that, for a same mechanism, we can derive privacy guarantees for reconstruction attacks that are better than the traditional ones from the literature. In particular, we show that larger privacy budgets do not protect against membership inference, but can still protect extraction of rare secrets. We show experimentally that our guarantees hold against various language models, including GPT-2 finetuned on Wikitext-103.
更多查看译文
关键词
Rényi Differential Privacy,Reconstruction Attacks,Information Theory
AI 理解论文
溯源树
样例
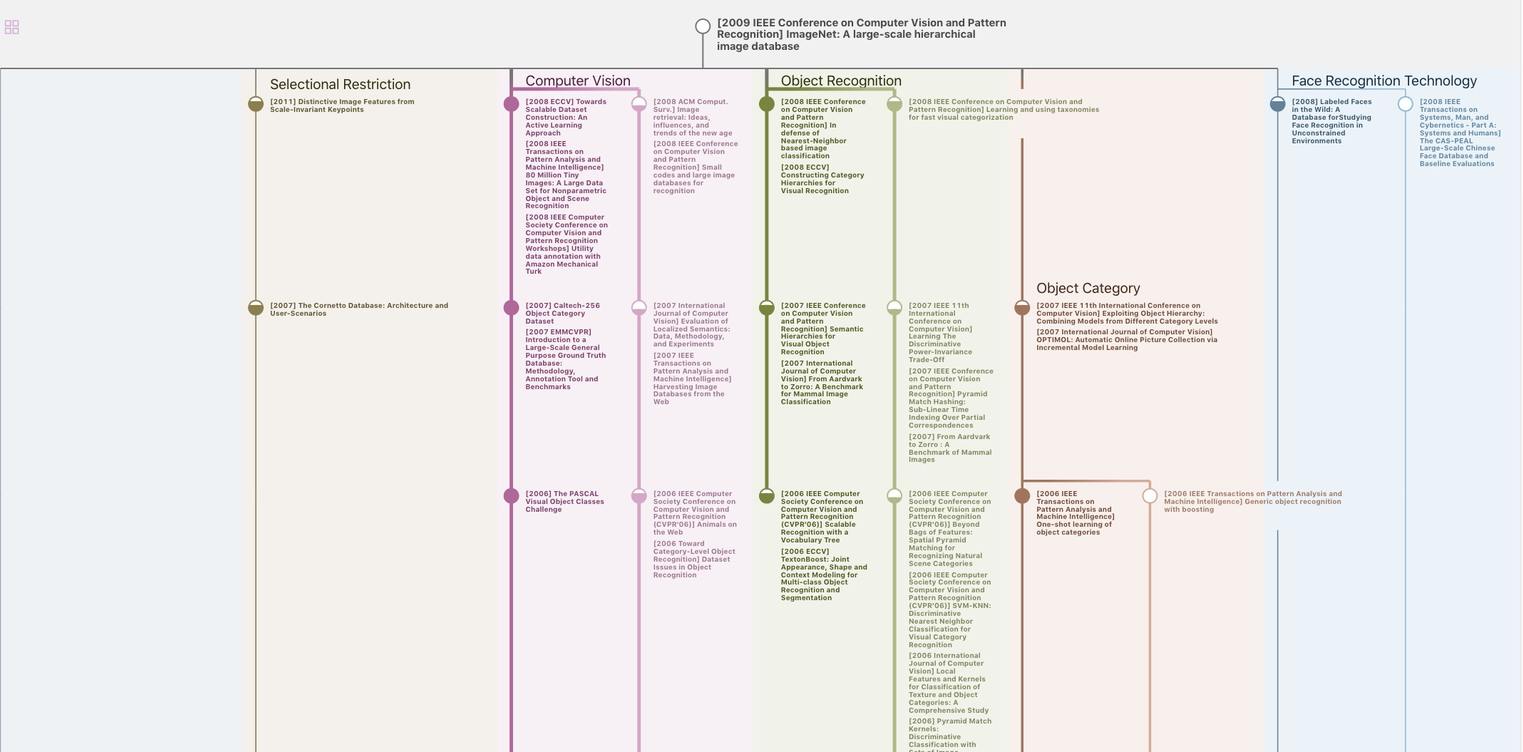
生成溯源树,研究论文发展脉络
Chat Paper
正在生成论文摘要