Learning to Approximate: Auto Direction Vector Set Generation for Hypervolume Contribution Approximation
IEEE TRANSACTIONS ON EVOLUTIONARY COMPUTATION(2024)
摘要
Hypervolume contribution is an important concept in evolutionary multiobjective optimization (EMO). It involves hypervolume-based EMO algorithms and hypervolume subset selection algorithms. Its main drawback is that it is computationally expensive in high-dimensional spaces, which limits its applicability to many-objective optimization. Recently, an R2 indicator variant (i.e., R-2(HVC) indicator) is proposed to approximate the hypervolume contribution. The R-2(HVC) indicator uses line segments along a number of direction vectors for hypervolume contribution approximation. It has been shown that different direction vector sets lead to different approximation qualities. In this article, we propose learning to approximate (LtA), a direction vector set generation method for the R-2(HVC) indicator. The direction vector set is automatically learned from training data. The learned direction vector set can then be used in the R-2(HVC) indicator to improve its approximation quality. The usefulness of the proposed LtA method is examined by comparing it with other commonly used direction vector set generation methods for the R-2(HVC) indicator. Experimental results suggest the superiority of LtA over the other methods for generating high-quality direction vector sets.
更多查看译文
关键词
Approximation,evolutionary multiobjective optimization (EMO),hypervolume contribution,hypervolume indicator
AI 理解论文
溯源树
样例
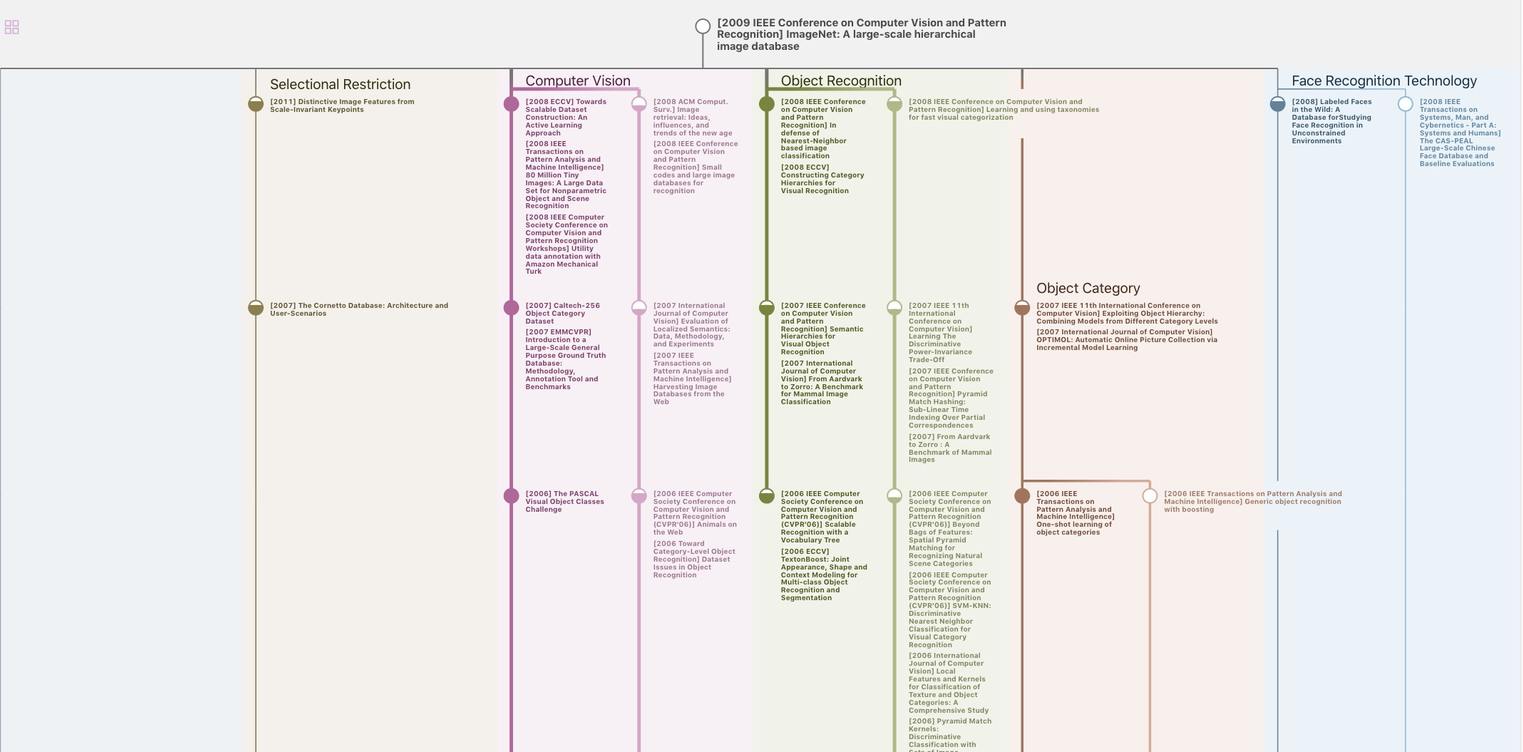
生成溯源树,研究论文发展脉络
Chat Paper
正在生成论文摘要