Learn to Forget: Machine Unlearning via Neuron Masking
user-5e9d449e4c775e765d44d7c9(2023)
摘要
Nowadays, machine learning models, especially neural networks, have became prevalent in many real-world applications. These models are trained based on a one-way trip from user data: as long as users contribute their data, there is no way to withdraw. To this end,
machine unlearning
becomes a popular research topic, which allows the model trainer to unlearn unexpected data from a trained machine learning model. In this article, we propose the first uniform metric called forgetting rate to measure the effectiveness of a machine unlearning method. It is based on the concept of membership inference and describes the transformation rate of the eliminated data from “memorized” to “unknown” after conducting unlearning. We also propose a novel unlearning method called
Forsaken
. It is superior to previous work in either utility or efficiency (when achieving the same forgetting rate). We benchmark
Forsaken
with eight standard datasets to evaluate its performance. The experimental results show that it can achieve more than 90% forgetting rate on average and only causeless than 5% accuracy loss.
更多查看译文
关键词
Machine unlearning, neuron masking, neural network
AI 理解论文
溯源树
样例
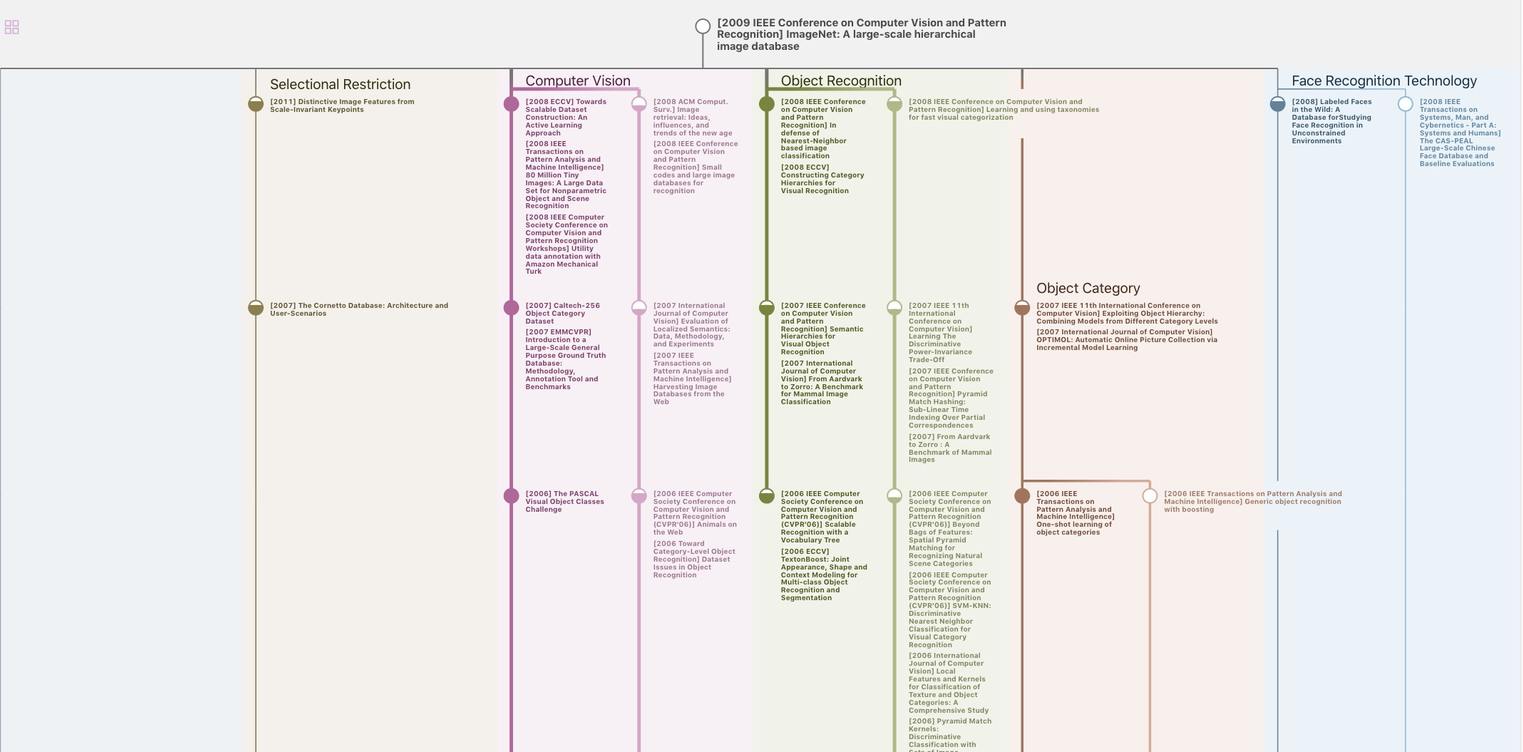
生成溯源树,研究论文发展脉络
Chat Paper
正在生成论文摘要