Online and Offline Machine Learning for Industrial Design Flow Tuning
2021 IEEE/ACM INTERNATIONAL CONFERENCE ON COMPUTER AIDED DESIGN (ICCAD)(2021)
摘要
Modern logic and physical synthesis tools provide numerous options and parameters that can drastically affect design quality; however, the large number of options leads to a complex design space difficult kir human designers to navigate. Fortunately, machine learning approaches and cloud computing environments are well suited for tackling complex parameter tuning problems like those seen in VLSI design flows. This paper proposes a holistic approach where online and offline machine learning approaches work together for tuning industrial design flows. We describe a system called SynTunSys (STS) that has been used to optimize multiple industrial high-performance processors. STS consists of an online system that optimizes designs and generates data for a recommender system that performs offline training and recommendation. Experimental results show the collaboration between STS online and offline machine learning systems as well as insight from human designers provide best-of-breed results. Finally, we discuss potential new directions for research on design flow tuning.
更多查看译文
关键词
industrial design flow tuning,offline machine learning,machine learning
AI 理解论文
溯源树
样例
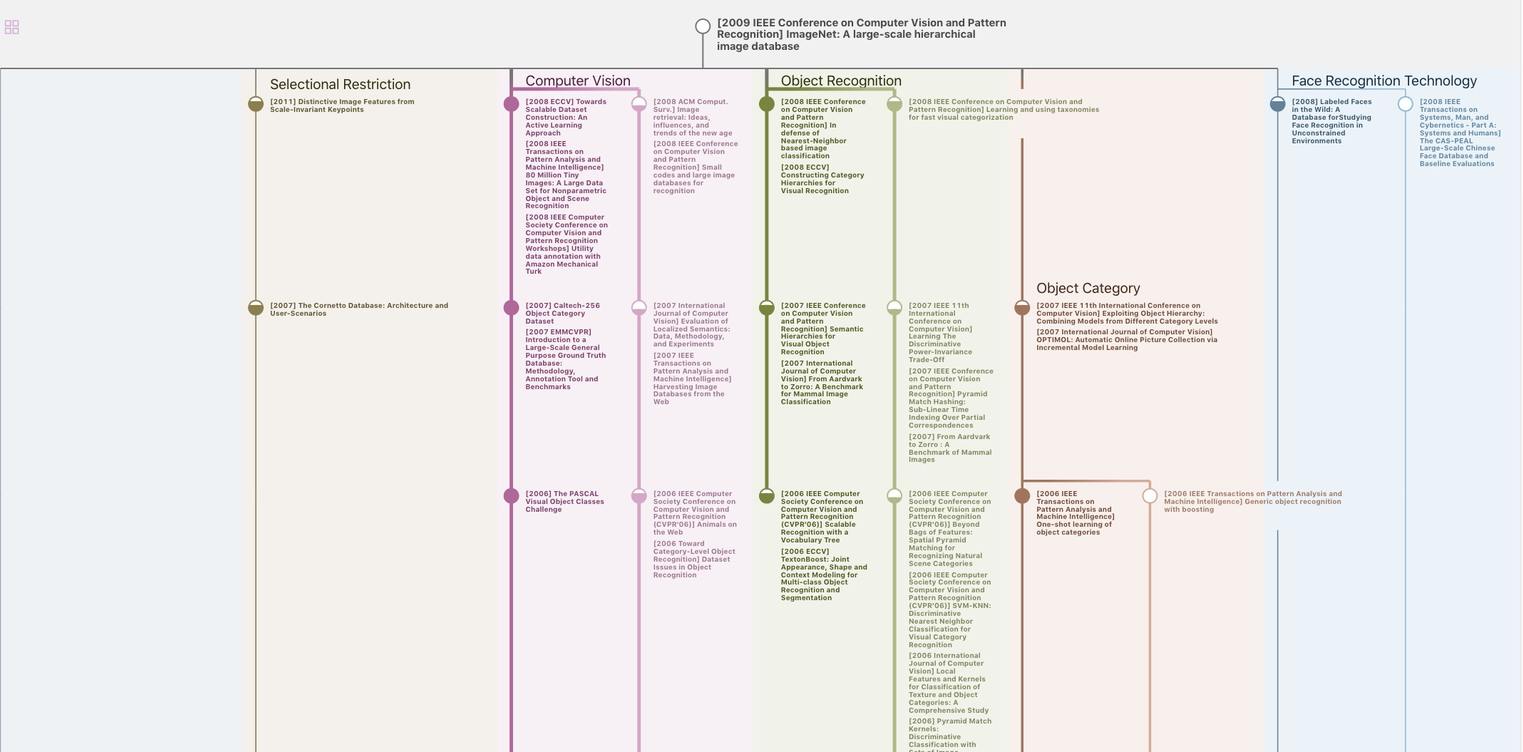
生成溯源树,研究论文发展脉络
Chat Paper
正在生成论文摘要