Graph Convolution Networks for Seismic Events Classification Using Raw Waveform Data From Multiple Stations
IEEE GEOSCIENCE AND REMOTE SENSING LETTERS(2022)
摘要
This letter proposes a multiple station-based seismic event classification model using a deep convolution neural network (CNN) and graph convolution network (GCN). To classify various seismic events, such as natural earthquakes, artificial earthquakes, and noise, the proposed model consists of weight-shared convolution layers, graph convolution layers, and fully connected layers. We employed graph convolution layers in order to aggregate features from multiple stations. Representative experimental results with the Korean peninsula earthquake datasets from 2016 to 2019 showed that the proposed model is superior to the single-station based state-of the-art methods. Moreover, the proposed model significantly reduced false alarms when using continuous waveforms of long duration. The code is available at.(1)
更多查看译文
关键词
Earthquakes,Convolution,Feature extraction,Data models,Convolutional neural networks,Adaptation models,Neural networks,Convolution neural network (CNN),deep learning,graph convolution network (GCN),multiple station,seismic event classification
AI 理解论文
溯源树
样例
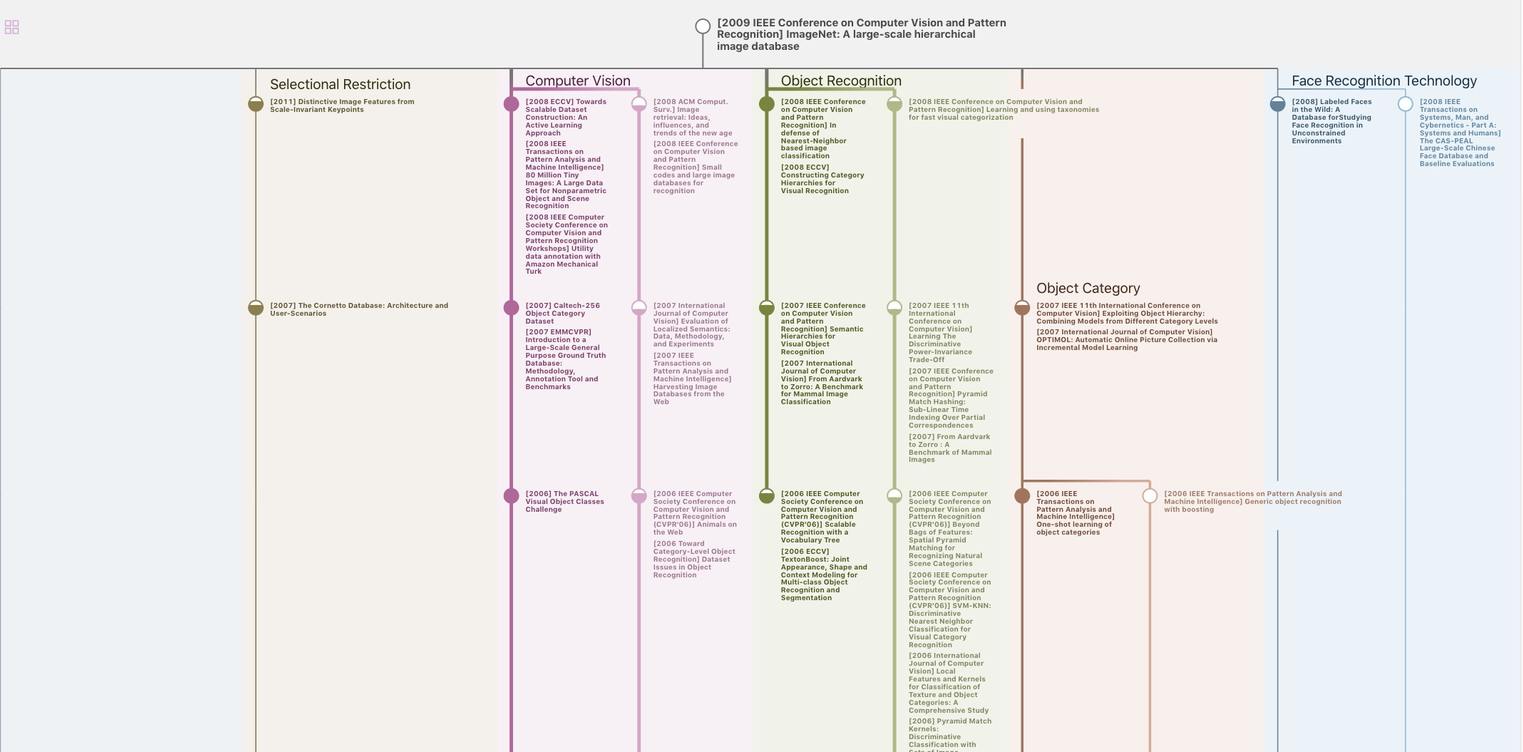
生成溯源树,研究论文发展脉络
Chat Paper
正在生成论文摘要