Precious: Resource-Demand Estimation for Embedded Neural Network Accelerators
semanticscholar(2020)
摘要
The recent advances of hardware-based accelerators for machine learning—in particular neural networks—attracted the attention of embedded-systems designers and engineers. Since embedded systems usually operate with strict resource constraints, knowledge about the resource demand (i.e., time and power) for executing machine-learning workloads is key. This paper presents Precious, an approach, as well as a practical implementation, of a system that estimates execution time and power draw of convolutional and fully-connected neural networks that execute on a commerciallyavailable off-the-shelf embedded accelerator hardware for neural networks (i.e., Google Coral Edge TPU). ACM Reference Format: Stefan Reif, Benedict Herzog, Judith Hemp, Timo Hönig, and Wolfgang Schröder-Preikschat. 2020. Precious: Resource-Demand Estimation for Embedded Neural Network Accelerators. In First International Workshop on Benchmarking Machine Learning Workloads on Emerging Hardware, March 4, 2020, Austin, TX. ACM, New York, NY, USA, 9 pages.
更多查看译文
AI 理解论文
溯源树
样例
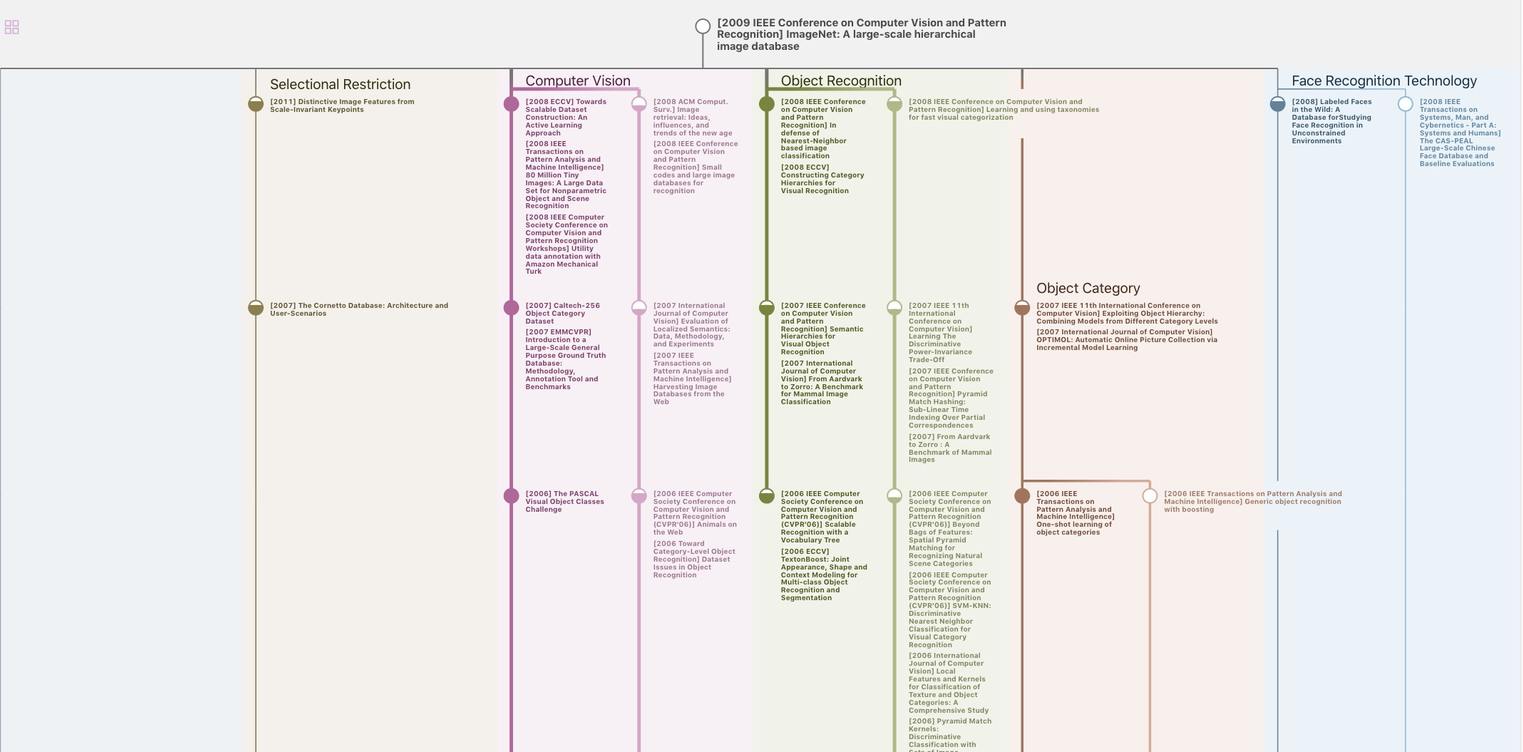
生成溯源树,研究论文发展脉络
Chat Paper
正在生成论文摘要