Machine learning methods applied to genotyping data capture interactions between single nucleotide variants in late onset Alzheimer's disease
medRxiv(2021)
摘要
INTRODUCTION Genome-wide association studies (GWAS) in late onset Alzheimer's disease (LOAD) provide lists of individual genetic determinants. However, GWAS are not good at capturing the synergistic effects among multiple genetic variants and lack good specificity. METHODS We applied tree-based machine learning algorithms (MLs) to discriminate LOAD (> 700 individuals) and age-matched unaffected subjects using single nucleotide variants (SNVs) from AD studies, obtaining specific genomic profiles with the prioritized SNVs. RESULTS The MLs prioritized a set of SNVs located in close proximity genes PVRL2, TOMM40, APOE and APOC1. The captured genomic profiles in this region showed a clear interaction between rs405509 and rs1160985. Additionally, rs405509 located in APOE promoter interacts with rs429358 among others, seemingly neutralizing their predisposing effect. Interactions are characterized by their association with specific comorbidities and the presence of eQTL and sQTLs. DISCUSSION Our approach efficiently discriminates LOAD from controls, capturing genomic profiles defined by interactions among SNVs in a hot-spot region.
更多查看译文
关键词
Apolipoprotein E, genetic determinants, genomic interactions, genomic profiles, late onset Alzheimer's disease, machine learning, single nucleotide variants, variant prioritization
AI 理解论文
溯源树
样例
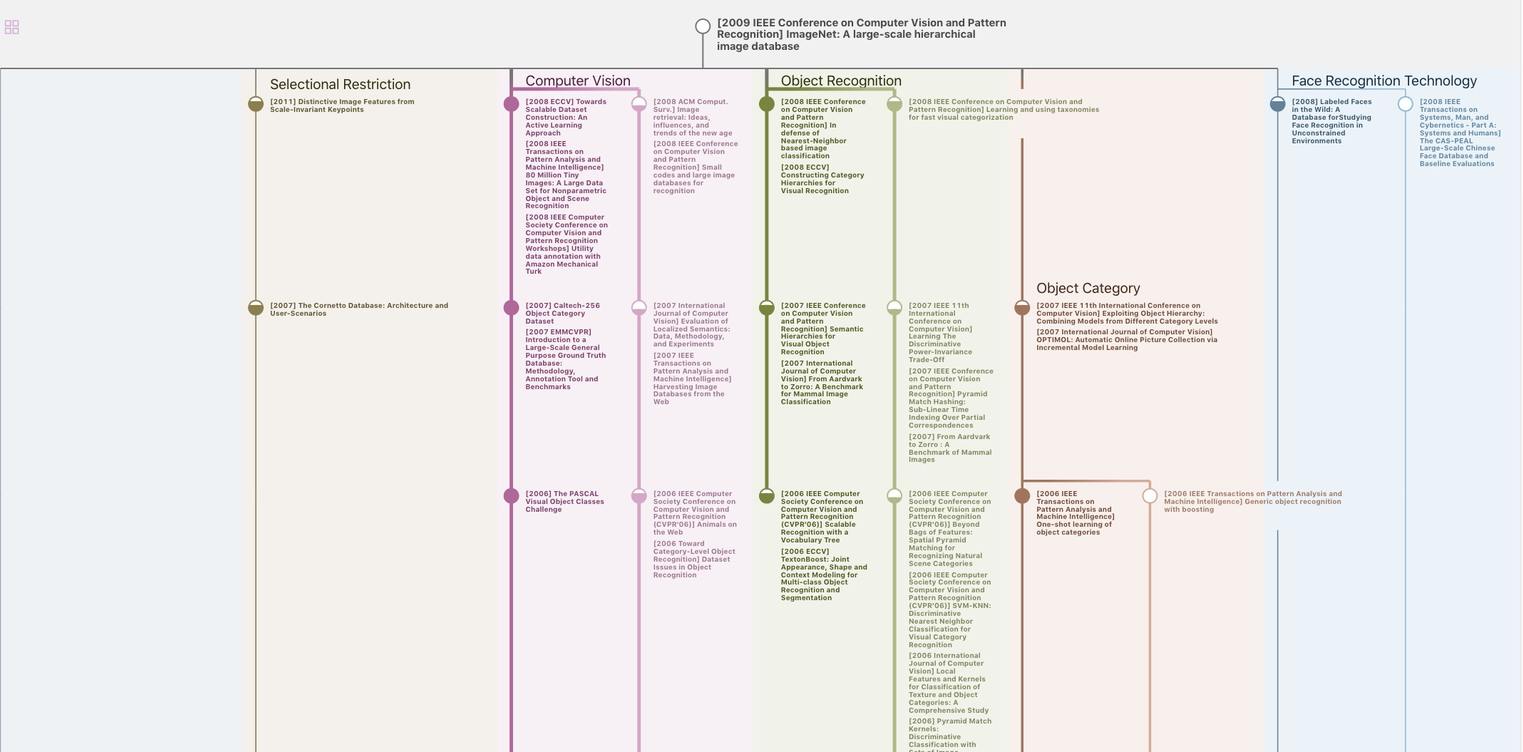
生成溯源树,研究论文发展脉络
Chat Paper
正在生成论文摘要