Simple Questions Generate Named Entity Recognition Datasets
emnlp 2022(2022)
摘要
Recent named entity recognition (NER) models often rely on human-annotated datasets, requiring the significant engagement of professional knowledge on the target domain and entities. This research introduces an ask-to-generate approach that automatically generates NER datasets by asking questions in simple natural language to an open-domain question answering system (e.g., "Which disease?"). Despite using fewer in-domain resources, our models, solely trained on the generated datasets, largely outperform strong low-resource models by an average F1 score of 19.4 for six popular NER benchmarks. Furthermore, our models provide competitive performance with rich-resource models that additionally leverage in-domain dictionaries provided by domain experts. In few-shot NER, we outperform the previous best model by an F1 score of 5.2 on three benchmarks and achieve new state-of-the-art performance.
更多查看译文
关键词
questions
AI 理解论文
溯源树
样例
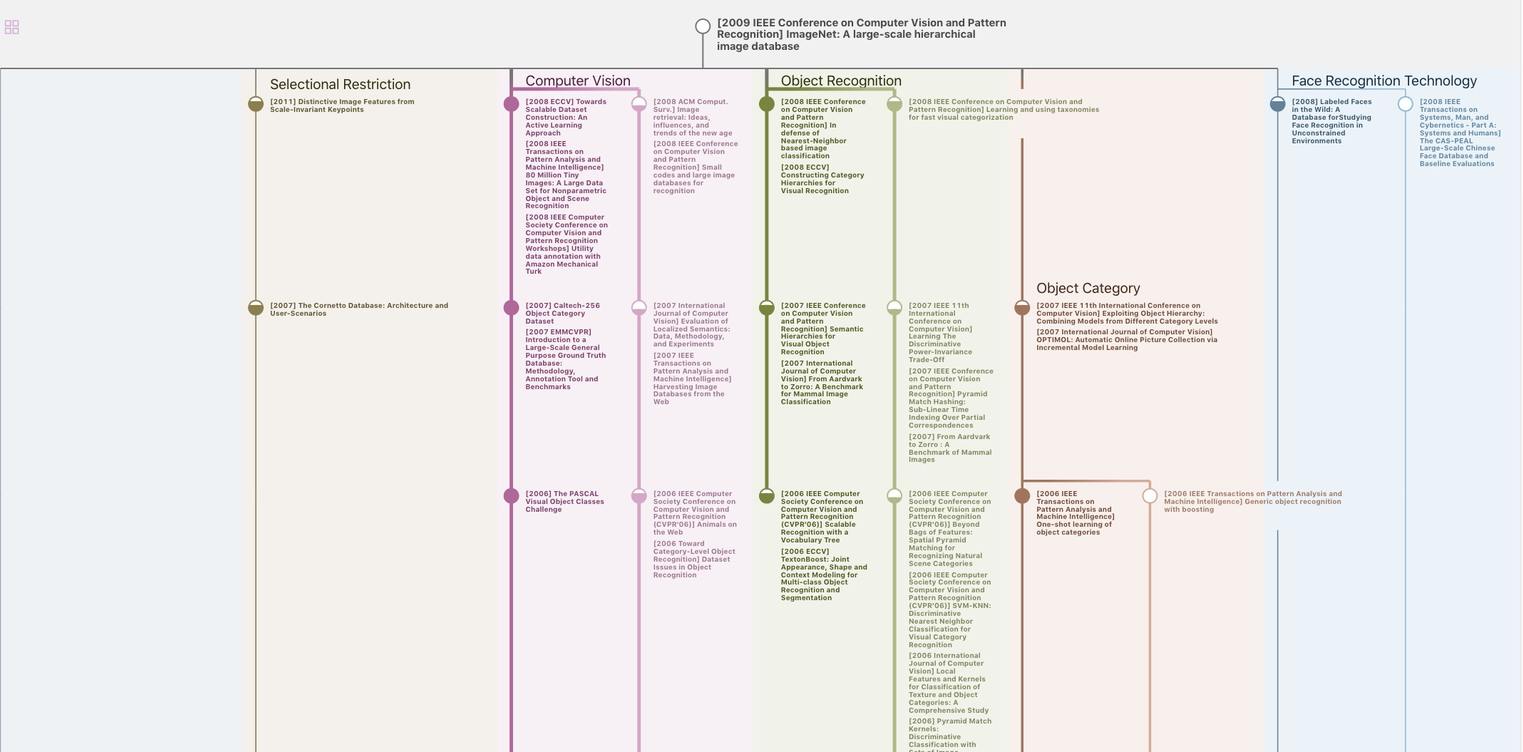
生成溯源树,研究论文发展脉络
Chat Paper
正在生成论文摘要