Factorized Fourier Neural Operators
ICLR 2023(2022)
摘要
We propose the Factorized Fourier Neural Operator (F-FNO), a learning-based approach for simulating partial differential equations (PDEs). Starting from a recently proposed Fourier representation of flow fields, the F-FNO bridges the performance gap between pure machine learning approaches to that of the best numerical or hybrid solvers. This is achieved with new representations - separable spectral layers and improved residual connections - and a combination of training strategies such as the Markov assumption, Gaussian noise, and cosine learning rate decay. On several challenging benchmark PDEs on regular grids, structured meshes, and point clouds, the F-FNO can scale to deeper networks and outperform both the FNO and the geo-FNO, reducing the error by 83% on the Navier-Stokes problem, 31% on the elasticity problem, 57% on the airfoil flow problem, and 60% on the plastic forging problem. Compared to the state-of-the-art pseudo-spectral method, the F-FNO can take a step size that is an order of magnitude larger in time and achieve an order of magnitude speedup to produce the same solution quality.
更多查看译文
关键词
fourier transform,fourier operators,pde,navier stokes
AI 理解论文
溯源树
样例
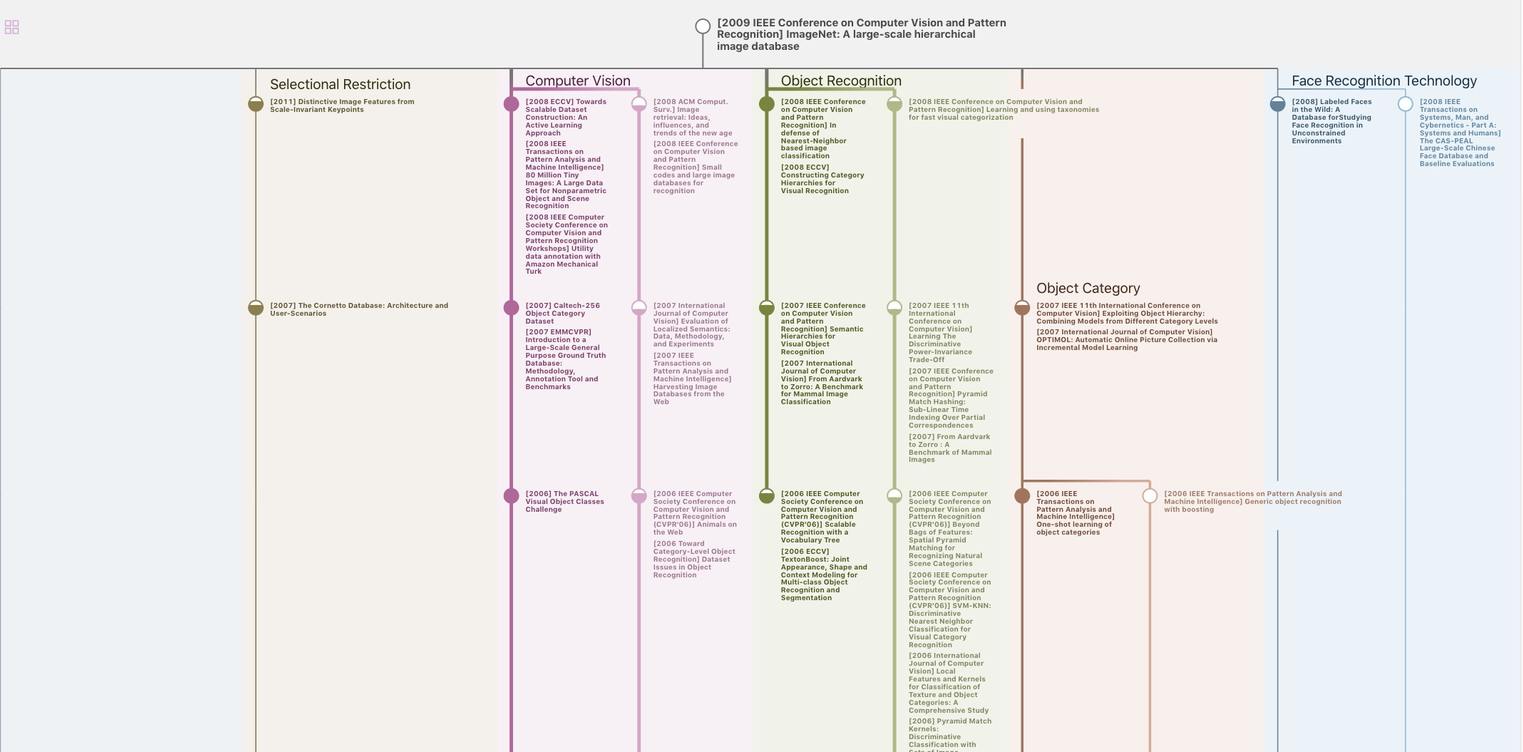
生成溯源树,研究论文发展脉络
Chat Paper
正在生成论文摘要