Continuous Latent Search for Combinatorial Optimization
user-607cde9d4c775e0497f57189(2020)
摘要
Combinatorial optimization problems are notoriously hard because they often require enumeration of the exponentially large solution space. Both classical solving techniques and machine learning-based approaches usually address combinatorial optimization problems by manipulating solutions in their original discrete form. In contrast, we propose a framework that consists of reparametrizing the original discrete solution space into a continuous latent space in which the problem can be (approximately) solved by running continuous optimization methods. We achieve this by learning a surrogate function that is shaped to correlate with the original objective when the latent solution is decoded back to the original solution space. We show that this approach can learn efficient solution strategies and is useful as a primal heuristic inside the widely-used open-source solver SCIP.
更多查看译文
AI 理解论文
溯源树
样例
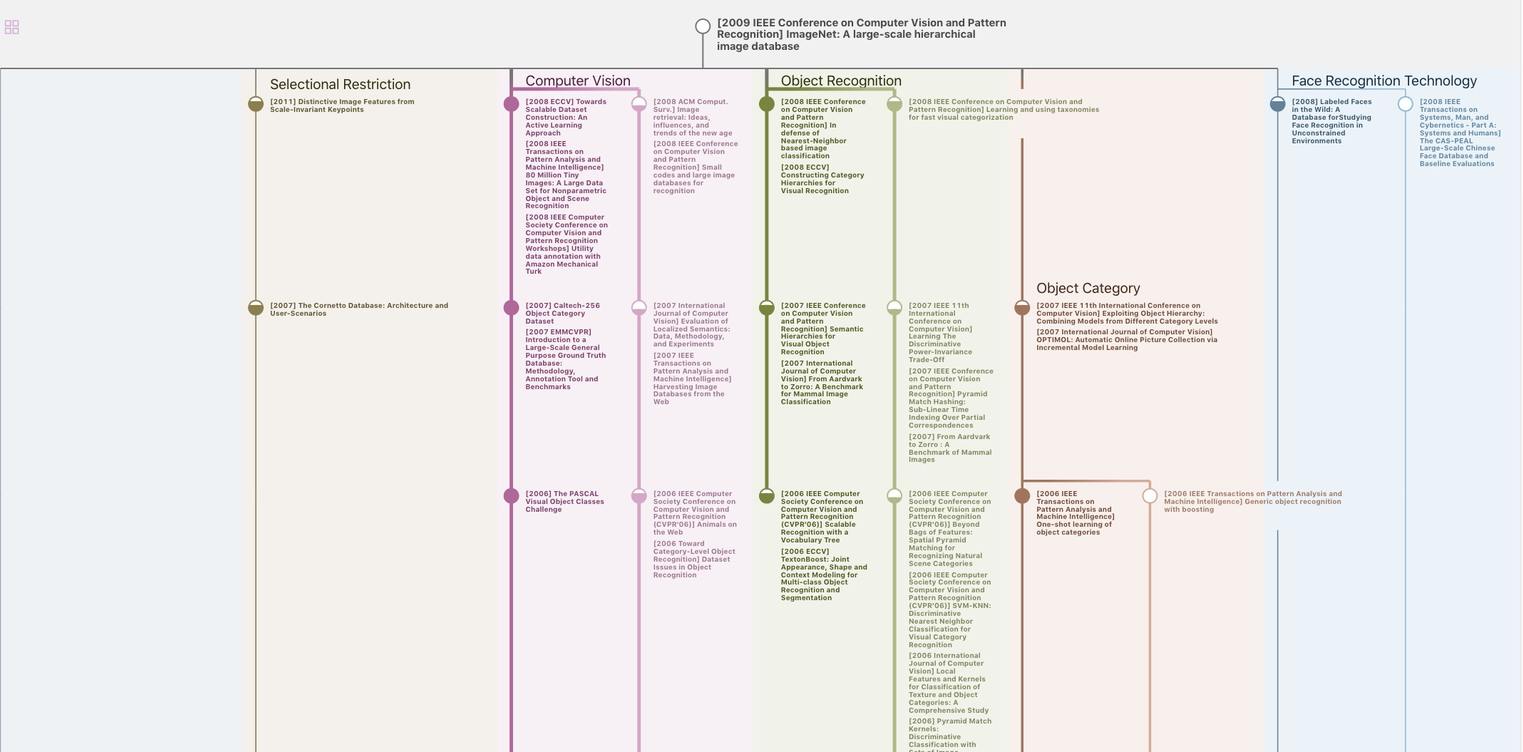
生成溯源树,研究论文发展脉络
Chat Paper
正在生成论文摘要