Compressive Object Tracking And Classification Using Deep Learning For Infrared Videos
PATTERN RECOGNITION AND TRACKING XXX(2019)
摘要
Object tracking and classification in infrared videos are challenging due to large variations in illumination, target sizes, and target orientations. Moreover, if the infrared videos only generate compressive measurements, then it will be even more difficult to perform target tracking and classification directly in the compressive measurement domain, as many conventional trackers and classifiers can only handle reconstructed frames from compressive measurements. This paper summarizes our research effort on target tracking and classification directly in the compressive measurement domain. We focus on one special type of compressive measurement using pixel subsampling. That is, the original pixels in the video frames are randomly subsampled. Even in such special compressive sensing setting, conventional trackers do not work in a satisfactory manner. We propose a deep learning approach that integrates YOLO (You Only Look Once) and ResNet (residual network) for multiple target tracking and classification. YOLO is used for multiple target tracking and ResNet is for target classification. Extensive experiments using short wave infrared (SWIR) videos demonstrated the efficacy of the proposed approach even though the training data are very scarce.
更多查看译文
关键词
Deep learning, compressive measurements, OMP, ALM-L1, YOLO, Res-Net, infrared videos, SWIR
AI 理解论文
溯源树
样例
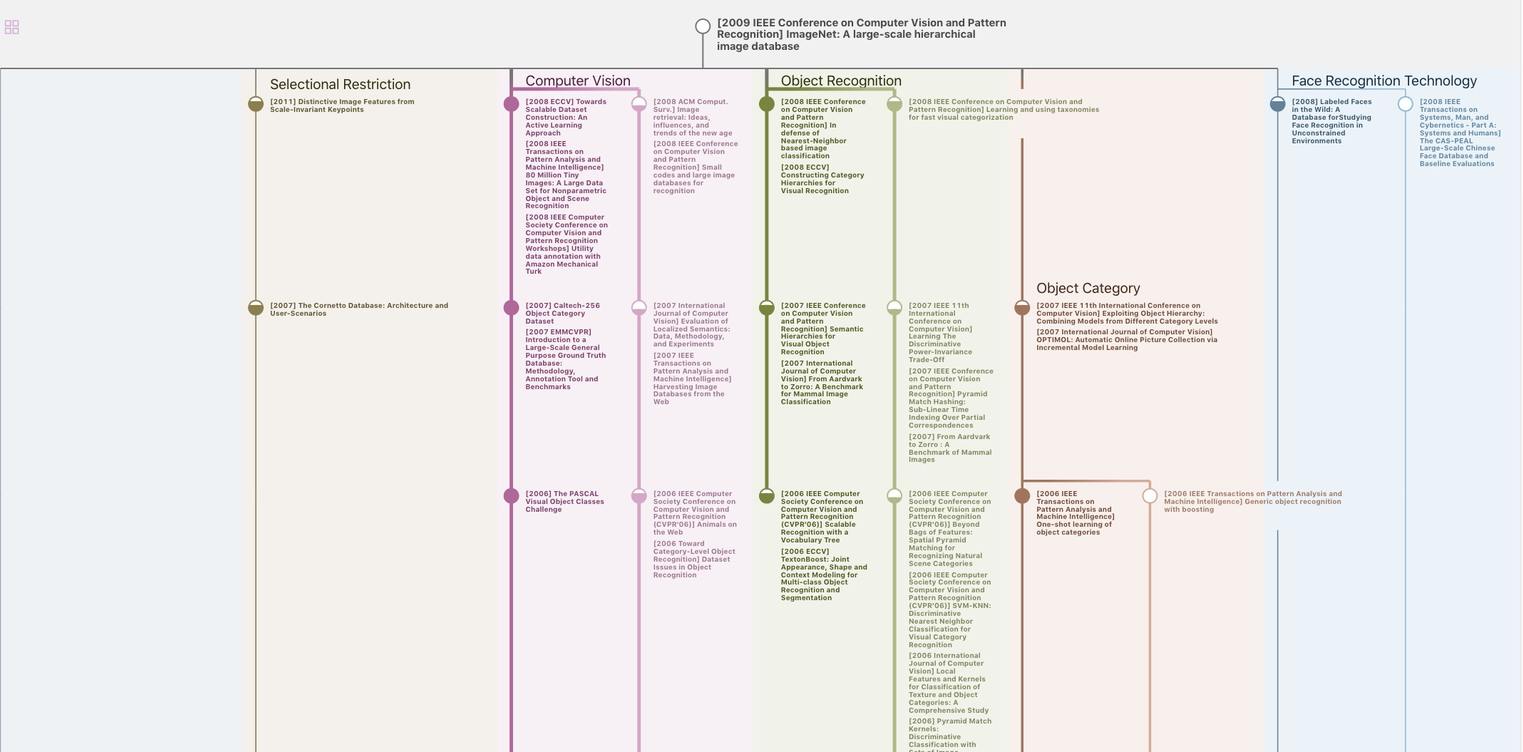
生成溯源树,研究论文发展脉络
Chat Paper
正在生成论文摘要