Quantum Algorithms and Lower Bounds for Linear Regression with Norm Constraints
arxiv(2022)
摘要
Lasso and Ridge are important minimization problems in machine learning and statistics. They are versions of linear regression with squared loss where the vector $\theta\in\mathbb{R}^d$ of coefficients is constrained in either $\ell_1$-norm (for Lasso) or in $\ell_2$-norm (for Ridge). We study the complexity of quantum algorithms for finding $\varepsilon$-minimizers for these minimization problems. We show that for Lasso we can get a quadratic quantum speedup in terms of $d$ by speeding up the cost-per-iteration of the Frank-Wolfe algorithm, while for Ridge the best quantum algorithms are linear in $d$, as are the best classical algorithms. As a byproduct of our quantum lower bound for Lasso, we also prove the first classical lower bound for Lasso that is tight up to polylog-factors.
更多查看译文
关键词
quantum,lower bounds,constraints,algorithms,norm
AI 理解论文
溯源树
样例
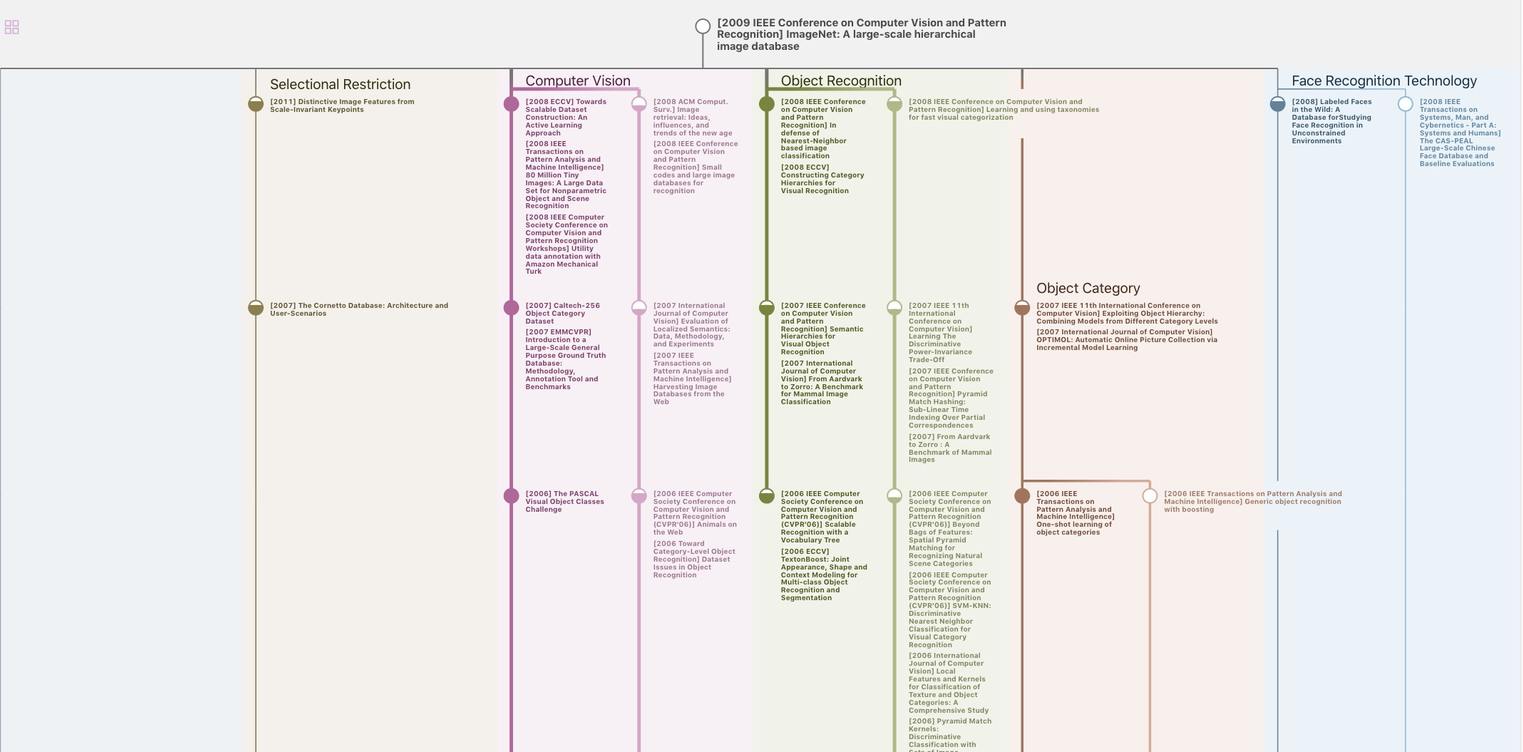
生成溯源树,研究论文发展脉络
Chat Paper
正在生成论文摘要