Online Bipartite Matching with Reusable Resources
MATHEMATICS OF OPERATIONS RESEARCH(2023)
摘要
We study the classic online bipartite matching problem with a twist: off-line vertices, called resources, are reusable. In particular, when a resource is matched to an online vertex, it is unavailable for a deterministic time duration d, after which it becomes available again for a rematch. Thus, a resource can be matched to many different online vertices over a period of time. Whereas recent work on the problem has resolved the asymptotic case in which we have large starting inventory (i.e., many copies) of every resource, we consider the (more general) case of unit inventory and give the first algorithms that are provably better than the naive greedy approach, which has a competitive ratio of (exactly) 0.5. Our first algorithm, which achieves a competitive ratio of 0.589, generalizes the classic RANKING algorithm for online bipartite matching of nonreusable resources (Karp et al. 1990) by reranking resources independently over time. Whereas reranking resources frequently has the same worst case performance as greedy, we show that reranking intermittently on a periodic schedule succeeds in addressing reusability of resources and performs significantly better than greedy in the worst case. Our second algorithm, which achieves a competitive ratio of 0.505, is a primal-dual randomized algorithm that works by suggesting up to two resources as candidate matches for every online vertex and then breaking the tie to make the final matching selection in a randomized correlated fashion over time. As a key component of our algorithm, we suitably adapt and extend the powerful technique of online correlated selection (Fahrbach et al. 2020) to reusable resources in order to induce negative correlation in our tie-breaking step and beat the competitive ratio of 0.5. Both of our results also extend to the case in which off-line vertices have weights.
更多查看译文
关键词
online bipartite matching,reusable resources,primal-dual analysis,online correlated selection,competitive ratio
AI 理解论文
溯源树
样例
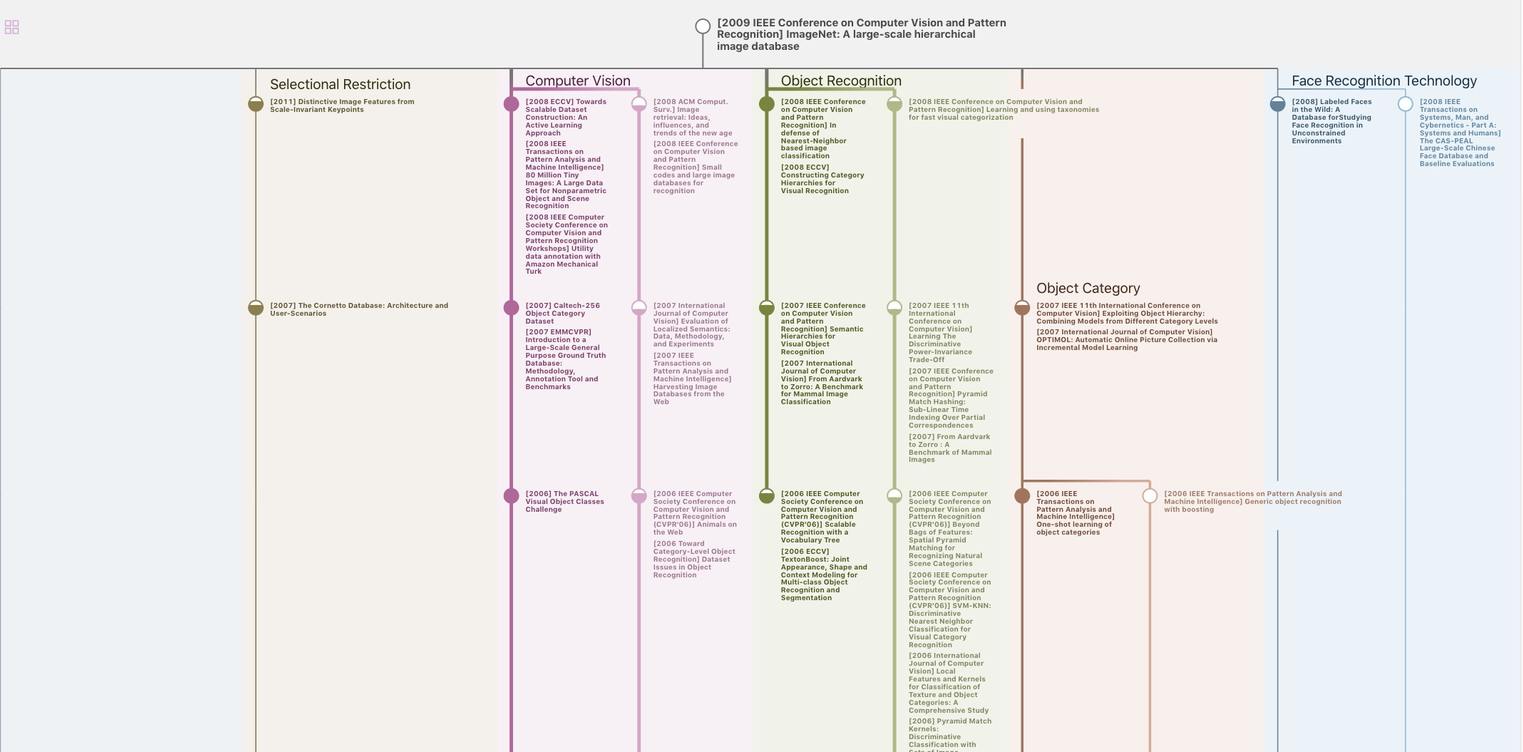
生成溯源树,研究论文发展脉络
Chat Paper
正在生成论文摘要