Joint Learning on the Hierarchy Representation for Fine-Grained Human Action Recognition.
ICIP(2021)
摘要
Fine-grained human action recognition is a core research topic in computer vision. Inspired by the recently proposed hierarchy representation of fine-grained actions in FineGym and SlowFast network for action recognition, we propose a novel multi-task network which exploits the FineGym hierarchy representation to achieve effective joint learning and prediction for fine-grained human action recognition. The multi-task network consists of three pathways of SlowOnly networks with gradually increased frame rates for events, sets and elements of fine-grained actions, followed by our proposed integration layers for joint learning and prediction. It is a two-stage approach, where it first learns deep feature representation at each hierarchical level, and is followed by feature encoding and fusion for multi-task learning. Our empirical results on the FineGym dataset achieve a new state-of-the-art performance, with 91.80% Top-1 accuracy and 88.46% mean accuracy for element actions, which are 3.40% and 7.26% higher than the previous best results.
更多查看译文
关键词
Action recognition,fine-grained action recognition,multi-task learning,joint representation
AI 理解论文
溯源树
样例
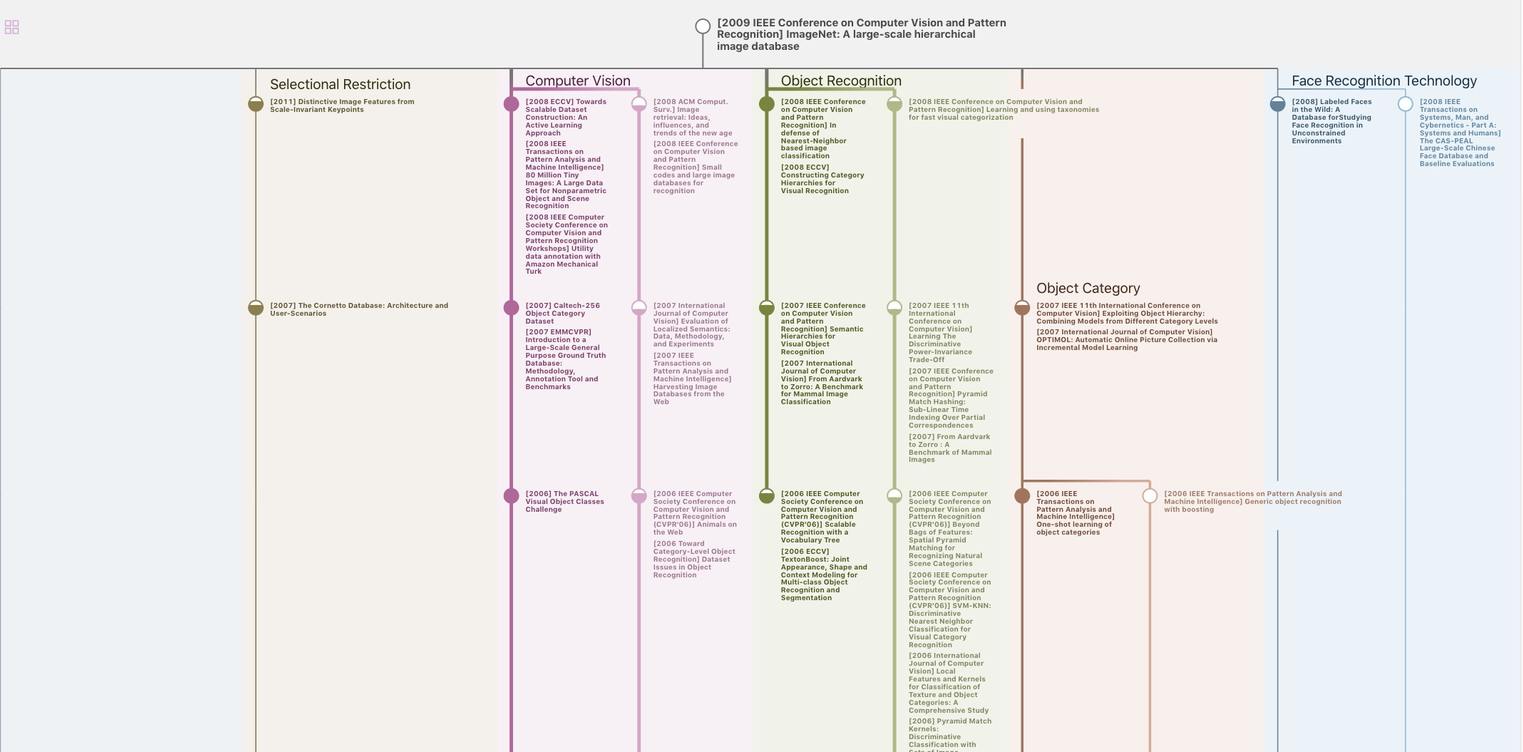
生成溯源树,研究论文发展脉络
Chat Paper
正在生成论文摘要