Learning from Deep Stereoscopic Attention for Simulator Sickness Prediction
IEEE Transactions on Visualization and Computer Graphics(2023)
摘要
Simulator sickness induced by 360° stereoscopic video contents is a prolonged challenging issue in Virtual Reality (VR) system. Current machine learning models for simulator sickness prediction ignore the underlying interdependencies and correlations across multiple visual features which may lead to simulator sickness. We propose a model for sickness prediction by automatic learning and adaptive integrating multi-level mappings from stereoscopic video features to simulator sickness scores. Firstly, saliency, optical flow and disparity features are extracted from videos to reflect the factors causing simulator sickness, including human attention area, motion velocity and depth information. Then, these features are embedded and fed into a 3-dimensional convolutional neural network (3D CNN) to extract the underlying multi-level knowledge which includes low-level and higher-order visual concepts, and global image descriptor. Finally, an attentional mechanism is exploited to adaptively fuse multi-level information with attentional weights for sickness score estimation. The proposed model is trained by an end-to-end approach and validated over a public dataset. Comparison results with state-of-the-art models and ablation studies demonstrated improved performance in terms of Root Mean Square Error (RMSE) and Pearson Linear Correlation Coefficient.
更多查看译文
关键词
Stereoscopic video,simulator sickness,virtual reality,attention mechanism,3D CNN,I.4.9 [image processing and computer Vision]: applications,H.5.1 [information interfaces and presentation]: multimedia information systems
AI 理解论文
溯源树
样例
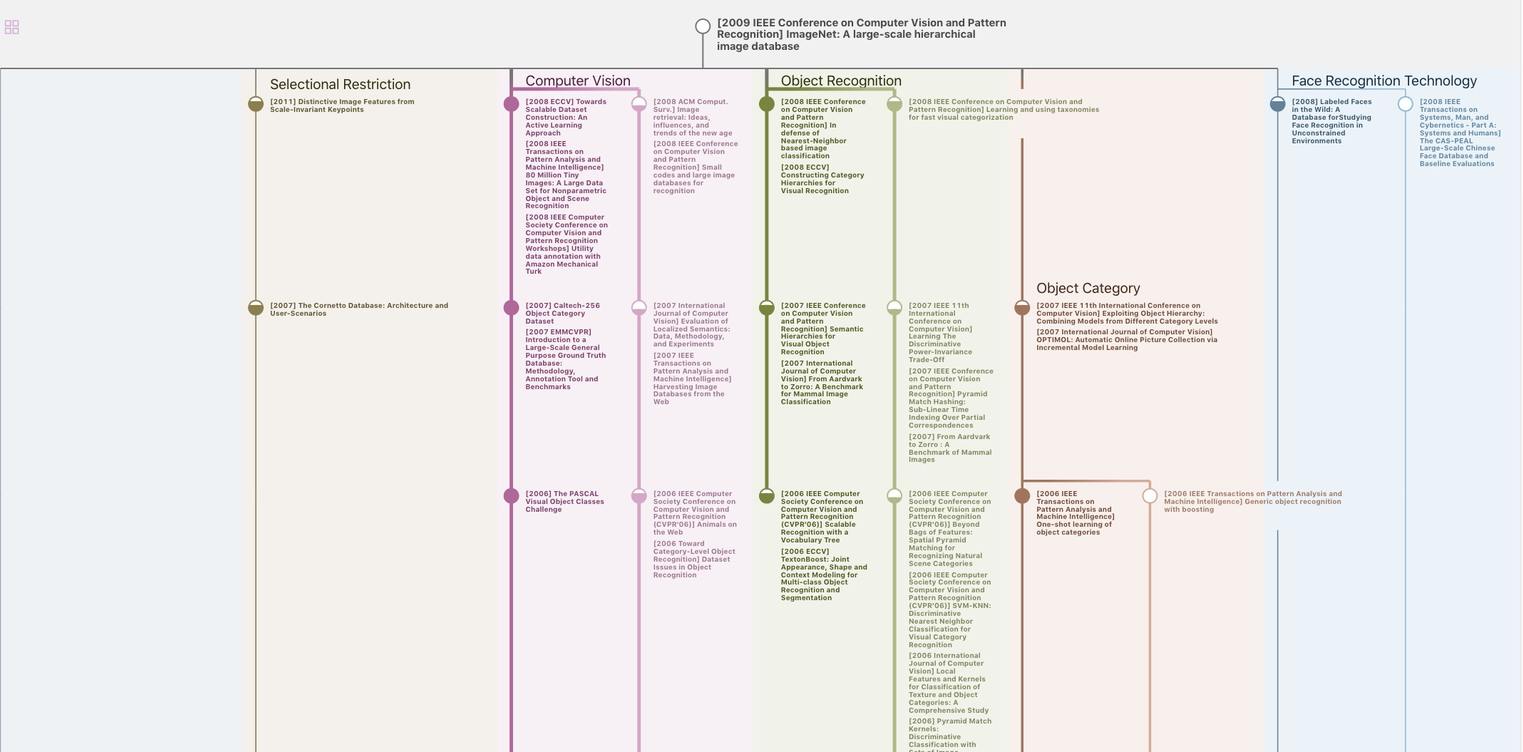
生成溯源树,研究论文发展脉络
Chat Paper
正在生成论文摘要