Universal Graph Compression: Stochastic Block Models
IEEE TRANSACTIONS ON INFORMATION THEORY(2024)
摘要
Motivated by the prevalent data science applications of processing large-scale graph data such as social networks and biological networks, this paper investigates lossless compression of data in the form of a labeled graph. Particularly, we consider a widely used random graph model, stochastic block model (SBM), which captures the clustering effects in social networks. An information-theoretic universal compression framework is applied, in which one aims to design a single compressor that achieves the asymptotically optimal compression rate, for every SBM distribution, without knowing the parameters of the SBM. Such a graph compressor is proposed in this paper, which universally achieves the optimal compression rate with polynomial time complexity for a wide class of SBMs. Existing universal compression techniques are developed mostly for stationary ergodic one-dimensional sequences. However, the adjacency matrix of SBM has complex two-dimensional correlations. The challenge is alleviated through a carefully designed transform that converts two-dimensional correlated data into almost i.i.d. submatrices. The sequence of submatrices is then compressed by a Krichevsky-Trofimov compressor, whose length analysis is generalized to identically distributed but arbitrarily correlated sequences. In four benchmark graph datasets, the compressed files from competing algorithms take 2.4 to 27 times the space needed by the proposed scheme.
更多查看译文
关键词
Compression algorithms,source coding,graph theory
AI 理解论文
溯源树
样例
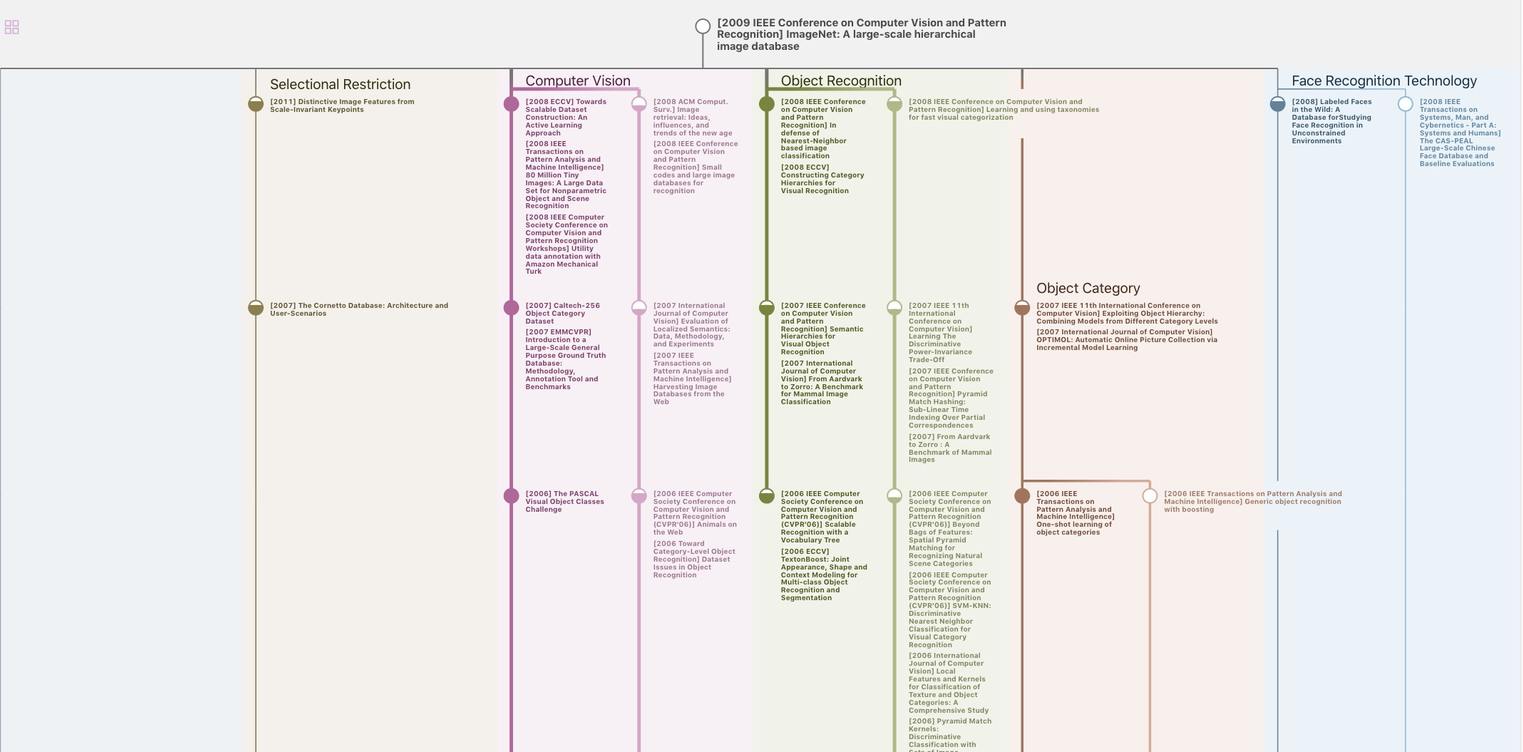
生成溯源树,研究论文发展脉络
Chat Paper
正在生成论文摘要