Statistical Estimation and Inference via Local SGD in Federated Learning
arxiv(2021)
摘要
Federated Learning (FL) makes a large amount of edge computing devices (e.g., mobile phones) jointly learn a global model without data sharing. In FL, data are generated in a decentralized manner with high heterogeneity. This paper studies how to perform statistical estimation and inference in the federated setting. We analyze the so-called Local SGD, a multi-round estimation procedure that uses intermittent communication to improve communication efficiency. We first establish a {\it functional central limit theorem} that shows the averaged iterates of Local SGD weakly converge to a rescaled Brownian motion. We next provide two iterative inference methods: the {\it plug-in} and the {\it random scaling}. Random scaling constructs an asymptotically pivotal statistic for inference by using the information along the whole Local SGD path. Both the methods are communication efficient and applicable to online data. Our theoretical and empirical results show that Local SGD simultaneously achieves both statistical efficiency and communication efficiency.
更多查看译文
关键词
local sgd,statistical estimation,learning,inference
AI 理解论文
溯源树
样例
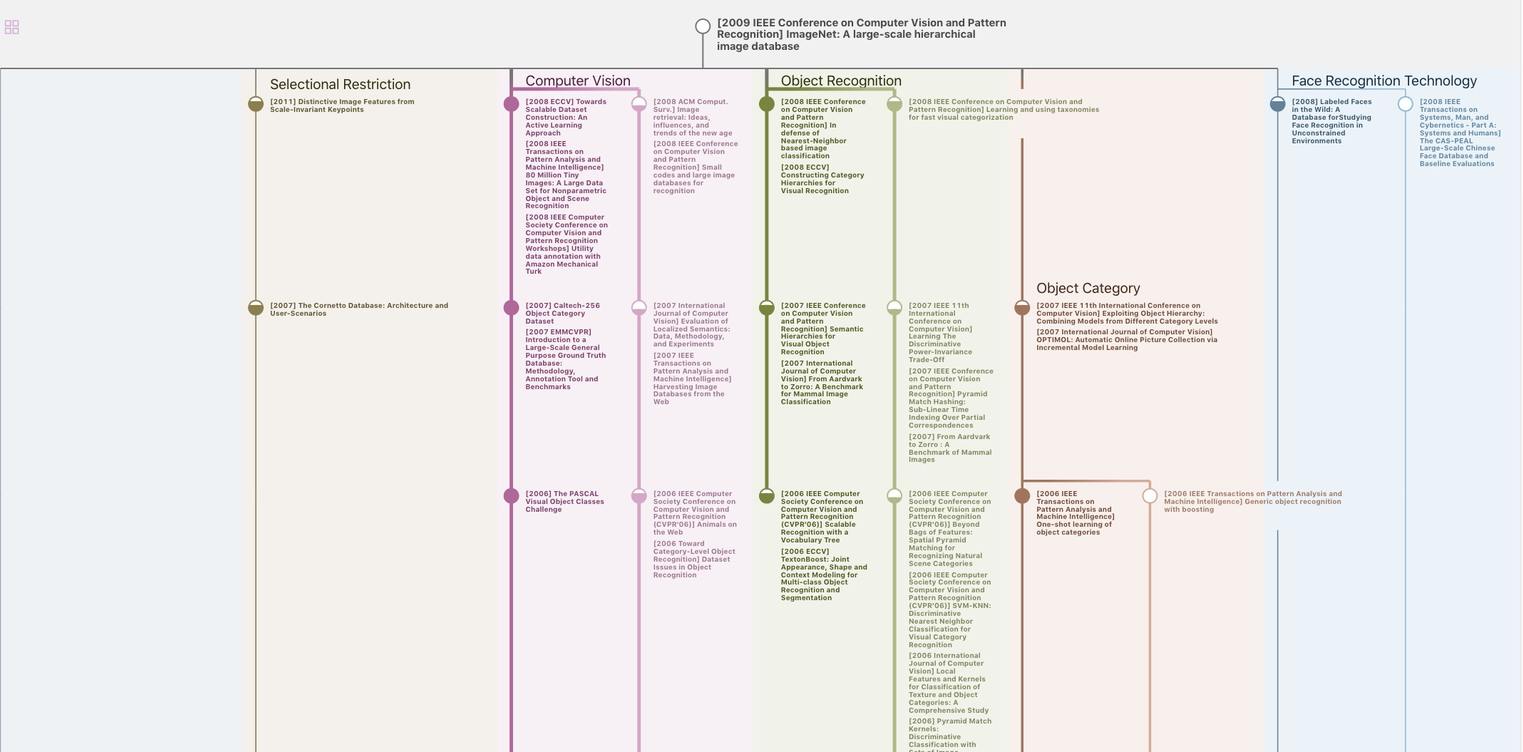
生成溯源树,研究论文发展脉络
Chat Paper
正在生成论文摘要