NowCasting-Nets: Representation Learning to Mitigate Latency Gap of Satellite Precipitation Products Using Convolutional and Recurrent Neural Networks
IEEE TRANSACTIONS ON GEOSCIENCE AND REMOTE SENSING(2022)
摘要
Accurate and timely estimation of precipitation is critical for issuing hazard warnings (e.g., for flash floods or landslides). Current remotely sensed precipitation products have a few hours of latency, associated with the acquisition and processing of satellite data. By applying a robust nowcasting system to these products, it is (in principle) possible to mitigate this latency and improve their applicability, value, and impact. However, the development of such a system is complicated by the chaotic nature of the atmosphere, lack of sufficient knowledge about the evolution of precipitation systems based on previous observations, and the consequent rapid changes that can occur in the structures of precipitation systems. In this work, we develop two approaches (hereafter referred to as NowCasting-nets) that use recurrent and convolutional deep neural network (DNN) structures to address the challenge of precipitation nowcasting. A total of five models are trained using global precipitation measurement (GPM) Integrated MultisatellitE Retrievals for GPM (IMERG) precipitation data over the Eastern contiguous United States (CONUS) and then tested against independent data for the Eastern and Western CONUS. The models were designed to provide forecasts with a lead time of up to 1.5 h, and by using a feedback loop approach, the ability of the models to extend the forecast time to 4.5 h was also investigated. The performance of the models was compared against the random forest (RF) and linear regression (LR) machine learning (ML) methods, a persistence benchmark (BM) that uses the most recent observation as the forecast, and optical flow (OF). Independent IMERG observations were used as a reference, and experiments were conducted to examine both overall statistics and case studies involving specific precipitation events. Overall, the forecasts provided by the NowCasting-net models are superior, with the convolutional NowCasting-net (CNC) achieving 42%, 24%, 18%, and 16% improvement on the test set mean squared error (MSE) over the BM, LR, RF, and OF models, respectively, for the Eastern CONUS. Results of further testing over the Western CONUS (which was not part of the training data) are encouraging and indicate the ability of the proposed models to learn the dynamics of precipitation systems without having explicit access to motion vectors and other auxiliary features and then to generalize to different hydro-geo-climatic conditions.
更多查看译文
关键词
Convolutional long short-term memory (ConvL-STM), deep learning (DL), deep neural networks (DNNs), global precipitation measurement (GPM), Integrated Multi-satellitE Retrievals for GPM (IMERG), precipitation nowcasting, UNET
AI 理解论文
溯源树
样例
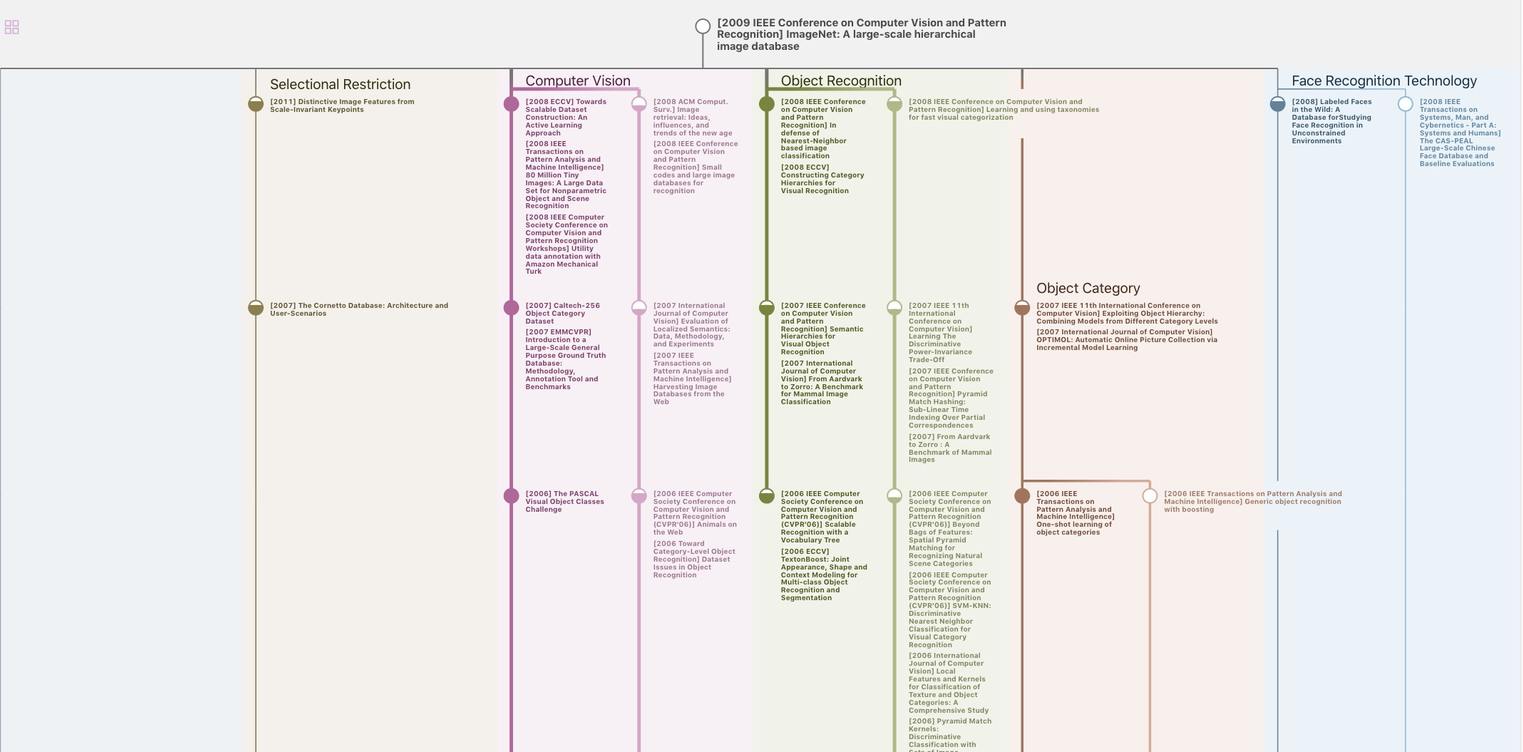
生成溯源树,研究论文发展脉络
Chat Paper
正在生成论文摘要