Leveraging Type Descriptions for Zero-shot Named Entity Recognition and Classification.
59TH ANNUAL MEETING OF THE ASSOCIATION FOR COMPUTATIONAL LINGUISTICS AND THE 11TH INTERNATIONAL JOINT CONFERENCE ON NATURAL LANGUAGE PROCESSING, VOL 1 (ACL-IJCNLP 2021)(2021)
摘要
A common issue in real-world applications of named entity recognition and classification (NERC) is the absence of annotated data for target entity classes during training. Zero-shot learning approaches address this issue by learning models that can transfer information from observed classes in the training data to unseen classes. This paper presents the first approach for zero-shot NERC, introducing a novel architecture that leverage the fact that textual descriptions for many entity classes occur naturally. Our architecture addresses the zero-shot NERC specific challenge that the not-an-entity class is not well defined, since different entity classes are considered in training and testing. For evaluation, we adapt two datasets, OntoNotes and MedMentions, emulating the difficulty of real-world zero-shot learning by testing models on the rarest entity classes. Our proposed approach outperforms baselines adapted from machine reading comprehension and zero-shot text classification. Furthermore, we assess the effect of different class descriptions for this task.
更多查看译文
关键词
Named-entity recognition,Shot (pellet),Zero (linguistics),Pattern recognition,Computer science,Type (model theory),Artificial intelligence
AI 理解论文
溯源树
样例
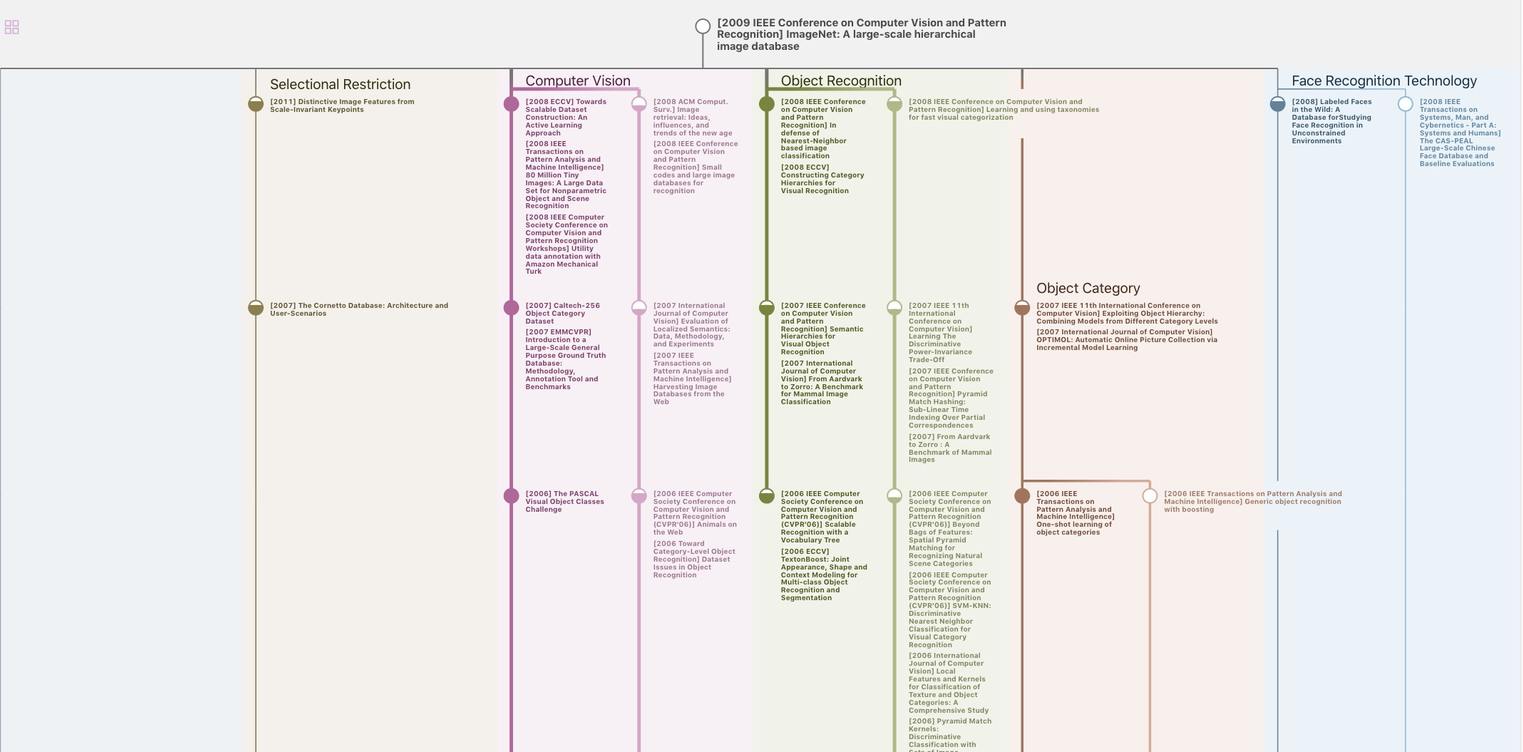
生成溯源树,研究论文发展脉络
Chat Paper
正在生成论文摘要