Debiased Explainable Pairwise Ranking from Implicit Feedback
ACM Conference On Recommender Systems(2021)
摘要
ABSTRACTRecent work in recommender systems has emphasized the importance of fairness, with a particular interest in bias and transparency, in addition to predictive accuracy. In this paper, we focus on the state of the art pairwise ranking model, Bayesian Personalized Ranking (BPR), which has previously been found to outperform pointwise models in predictive accuracy, while also being able to handle implicit feedback. Specifically, we address two limitations of BPR: (1) BPR is a black box model that does not explain its outputs, thus limiting the user’s trust in the recommendations, and the analyst’s ability to scrutinize a model’s outputs; and (2) BPR is vulnerable to exposure bias due to the data being Missing Not At Random (MNAR). This exposure bias usually translates into an unfairness against the least popular items because they risk being under-exposed by the recommender system. In this work, we first propose a novel explainable loss function and a corresponding Matrix Factorization-based model called Explainable Bayesian Personalized Ranking (EBPR) that generates recommendations along with item-based explanations. Then, we theoretically quantify additional exposure bias resulting from the explainability, and use it as a basis to propose an unbiased estimator for the ideal EBPR loss. The result is a ranking model that aptly captures both debiased and explainable user preferences. Finally, we perform an empirical study on three real-world datasets that demonstrate the advantages of our proposed models.
更多查看译文
关键词
Fairness in AI, Debiased Machine Learning, Pairwise Ranking, Explainability, Exposure Bias
AI 理解论文
溯源树
样例
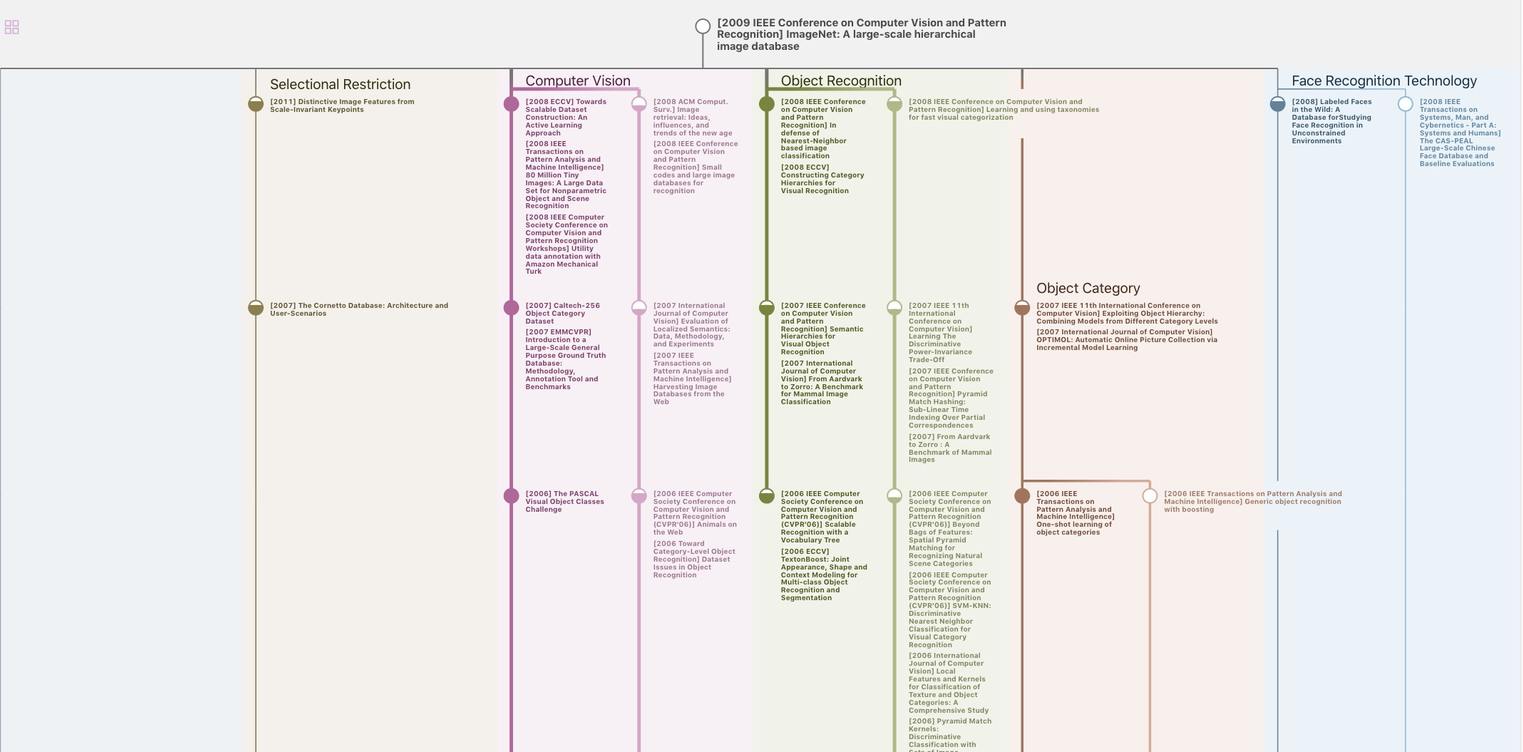
生成溯源树,研究论文发展脉络
Chat Paper
正在生成论文摘要