Finite-time Analysis of Globally Nonstationary Multi-Armed Bandits
arxiv(2021)
摘要
We consider nonstationary multi-armed bandit problems where the model parameters of the arms change over time. We introduce the adaptive resetting bandit (ADR-bandit), which is a class of bandit algorithms that leverages adaptive windowing techniques from the data stream community. We first provide new guarantees on the quality of estimators resulting from adaptive windowing techniques, which are of independent interest in the data mining community. Furthermore, we conduct a finite-time analysis of ADR-bandit in two typical environments: an abrupt environment where changes occur instantaneously and a gradual environment where changes occur progressively. We demonstrate that ADR-bandit has nearly optimal performance when the abrupt or global changes occur in a coordinated manner that we call global changes. We demonstrate that forced exploration is unnecessary when we restrict the interest to the global changes. Unlike the existing nonstationary bandit algorithms, ADR-bandit has optimal performance in stationary environments as well as nonstationary environments with global changes. Our experiments show that the proposed algorithms outperform the existing approaches in synthetic and real-world environments.
更多查看译文
关键词
finite-time,multi-armed
AI 理解论文
溯源树
样例
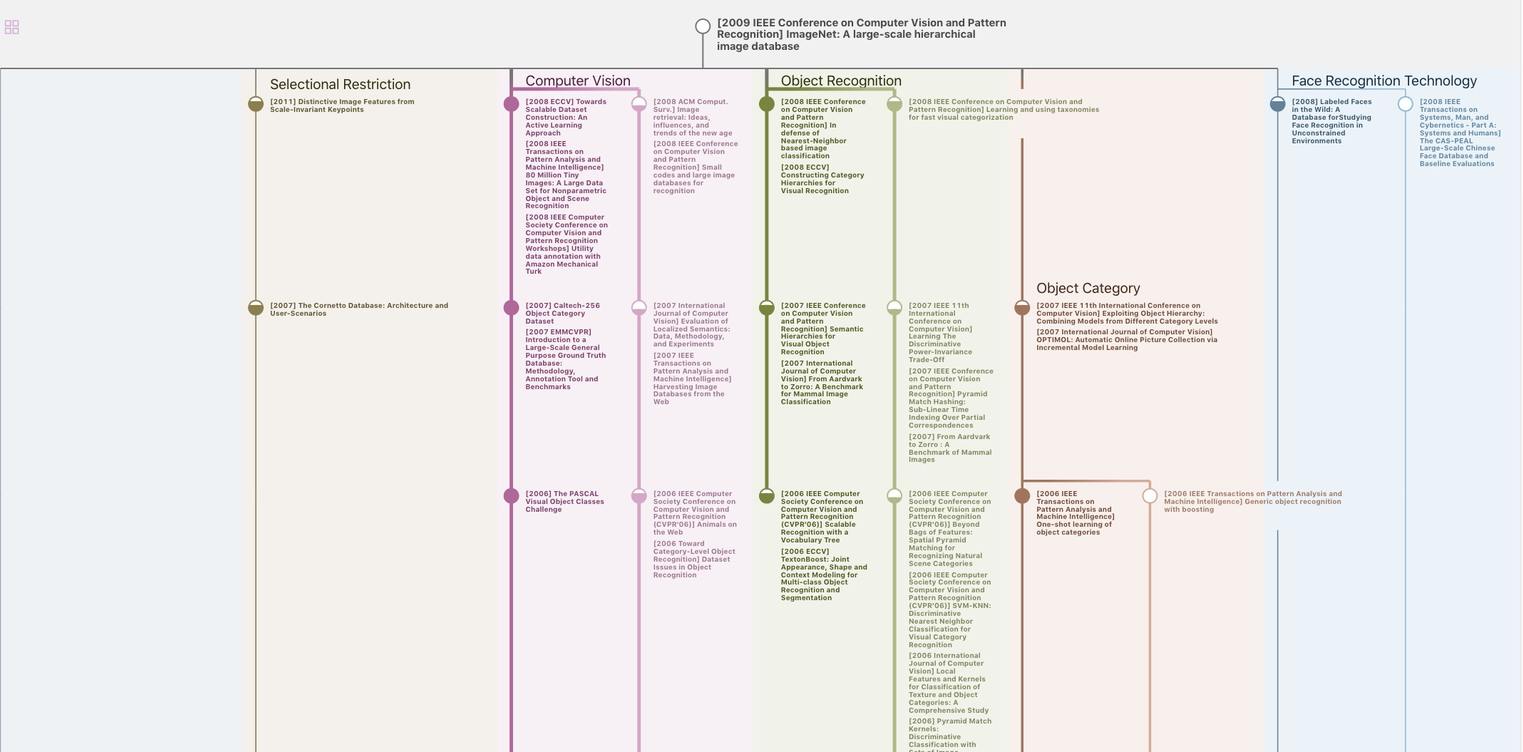
生成溯源树,研究论文发展脉络
Chat Paper
正在生成论文摘要