Optimal Rates for Nonparametric Density Estimation Under Communication Constraints
IEEE TRANSACTIONS ON INFORMATION THEORY(2024)
摘要
We consider density estimation for Besov spaces when each sample is quantized to only a limited number of bits. We provide a noninteractive adaptive estimator that exploits the sparsity of wavelet bases, along with a simulate-and-infer technique from parametric estimation under communication constraints. We show that our estimator is nearly rate-optimal by deriving minimax lower bounds that hold even when interactive protocols are allowed. Interestingly, while our wavelet-based estimator is almost rate-optimal for Sobolev spaces as well, it is unclear whether the standard Fourier basis, which arise naturally for those spaces, can be used to achieve the same performance.
更多查看译文
关键词
Estimation,Protocols,Information theory,Electronic mail,Convergence,Upper bound,Elbow,Density estimation,distributed adaptive estimation,quantization,interactive lower bound
AI 理解论文
溯源树
样例
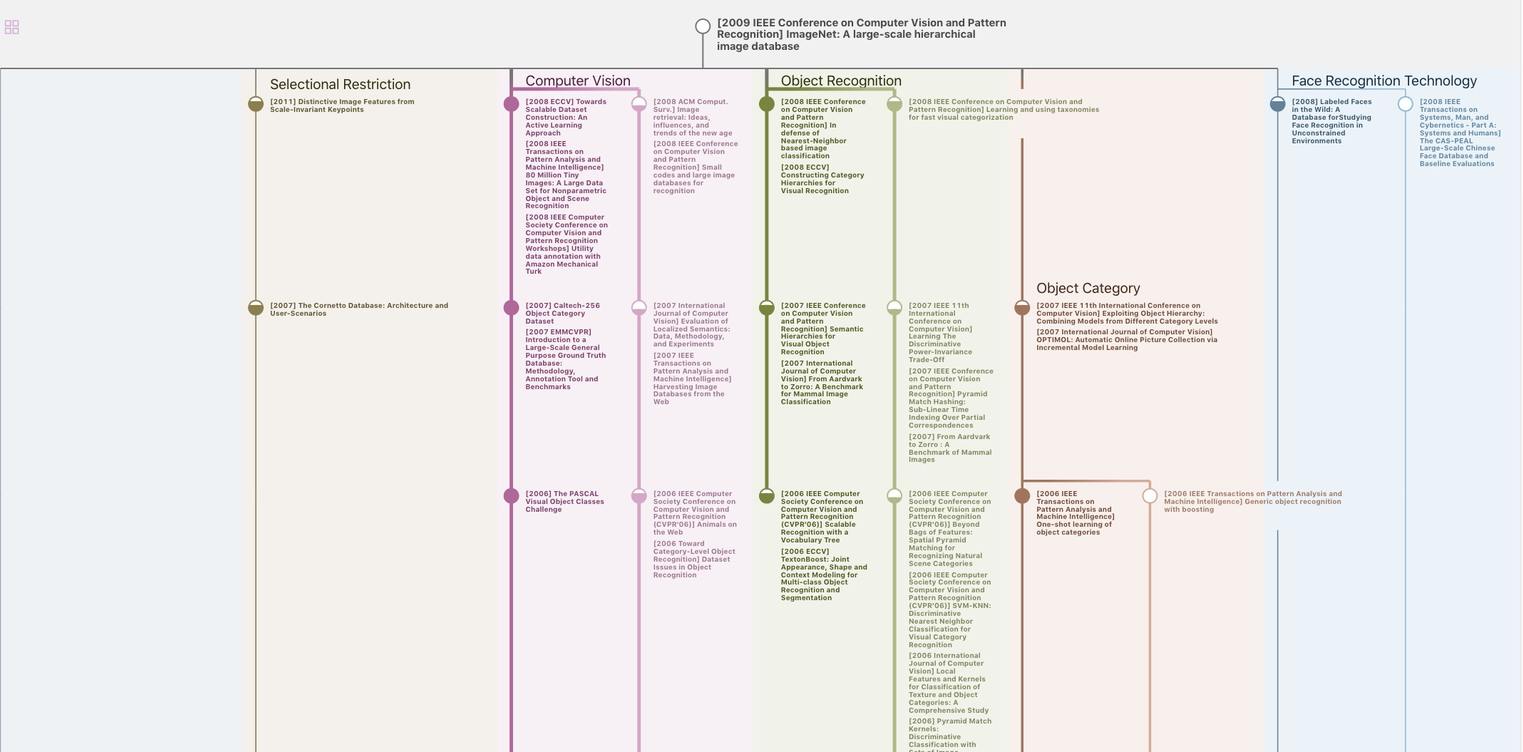
生成溯源树,研究论文发展脉络
Chat Paper
正在生成论文摘要