Improving Univariate Time Series Anomaly Detection Through Automatic Algorithm Selection and Human-in-the-Loop False-Positive Removement.
FLAIRS Conference(2021)
摘要
The existence of a time series anomaly detection method that performs well for all domains is a myth. Given a massive library of available methods, how can one select the best method for their application? An extensive evaluation of every anomaly detection method is not feasible. Many existing anomaly detection systems do not include an avenue for human feedback, essential given the subjective nature of what even is anomalous. We present a technique for improving univariate time series anomaly detection through automatic algorithm selection and human-in-the-loop false-positive removement. These determinations were made by extensively experimenting with over 30 pre-annotated time series from the open-source Numenta Anomaly Benchmark repository. Once the highest performing anomaly detection methods are selected via these characteristics, humans can annotate the predicted outliers which are used to tune anomaly scores via subsequence similarity search and improve the selected methods for their data, increasing evaluation scores and reducing the need for annotation by 70% on predicted anomalies where annotation is used to improve F-scores.
更多查看译文
AI 理解论文
溯源树
样例
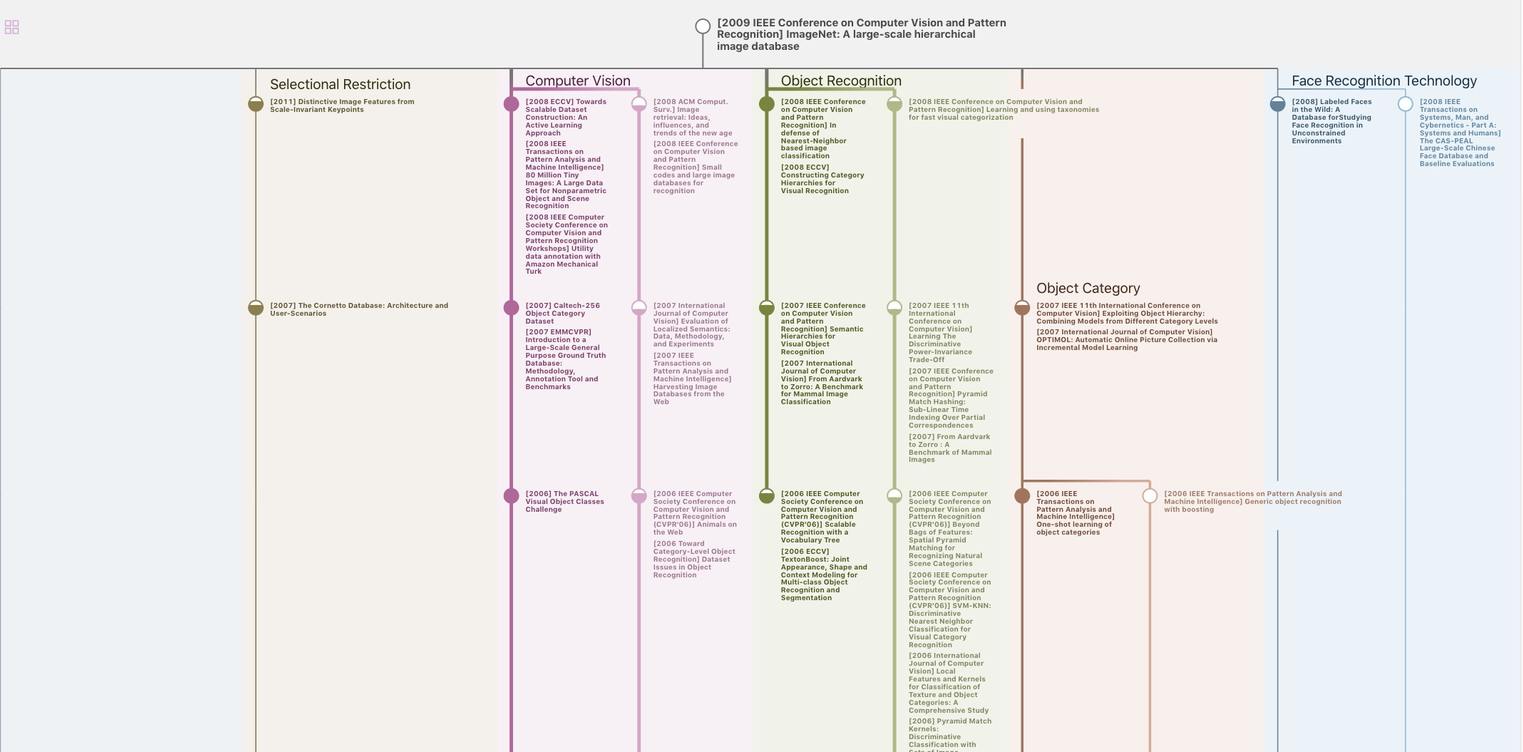
生成溯源树,研究论文发展脉络
Chat Paper
正在生成论文摘要