Design Optimization Of Renewable Energy Systems For Nzebs Based On Deep Residual Learning
RENEWABLE ENERGY(2021)
摘要
The design of renewable energy systems for Nearly Zero Energy Buildings (NZEB) is a complex optimization problem. In recent years, simulation-based optimization has demonstrated to be able to support the search for optimal design, but improvements to the method that are able to reduce the high computation time are needed. This study presents a new approach based on deep residual learning to make the search for optimal design solutions more efficient. It is applied to the problem of system design optimization for an Italian multi-family building case-study equipped with a solar cooling system. Given a design space defined by set of variables related to Heating, Ventilation and Air Conditioning systems (HVAC) and renewable systems, a machine learning method based on residual neural networks to predict and minimize the objective function characterizing non-renewable primary energy consumptions is proposed. Results have shown that the approach is able to successfully identify optimized design solutions (energy performance improved by 47%) with good prediction accuracy (error smaller than 3%) while reducing the overall computation time and maximizing the exploration of the design space, paving the way for further advancements in simulation-based optimization for NZEB design.(c) 2021 Published by Elsevier Ltd.
更多查看译文
关键词
System optimization, Solar cooling, Renewable energy systems, Machine learning, Residual neural network, TRNSYS dynamic Simulation
AI 理解论文
溯源树
样例
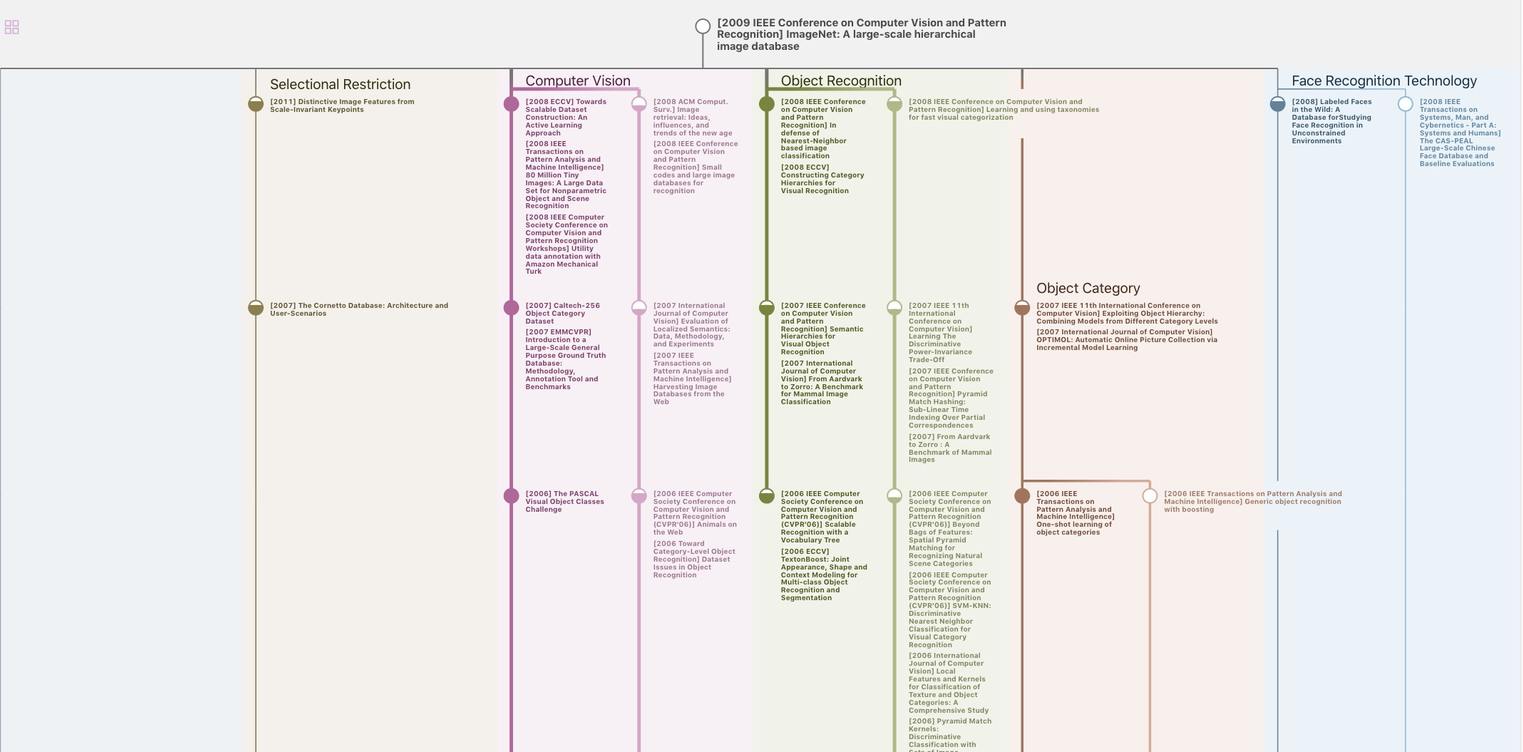
生成溯源树,研究论文发展脉络
Chat Paper
正在生成论文摘要