MPCSL - A Modular Pipeline for Causal Structure Learning
Knowledge Discovery and Data Mining(2021)
摘要
ABSTRACTThe examination of causal structures is crucial for data scientists in a variety of machine learning application scenarios. In recent years, the corresponding interest in methods of causal structure learning has led to a wide spectrum of independent implementations, each having specific accuracy characteristics and introducing implementation-specific overhead in the runtime. Hence, considering a selection of algorithms or different implementations in different programming languages utilizing different hardware setups becomes a tedious manual task with high setup costs. Consequently, a tool that enables to plug in existing methods from different libraries into a single system to compare and evaluate the results is substantial support for data scientists in their research efforts. In this work, we propose an architectural blueprint of a pipeline for causal structure learning and outline our reference implementation MPCSL that addresses the requirements towards platform independence and modularity while ensuring the comparability and reproducibility of experiments. Moreover, we demonstrate the capabilities of MPCSL within a case study, where we evaluate existing implementations of the well-known PC-Algorithm concerning their runtime performance characteristics.
更多查看译文
关键词
Causal Structure Learning, Modular Pipeline, Evaluation Framework, Benchmarking
AI 理解论文
溯源树
样例
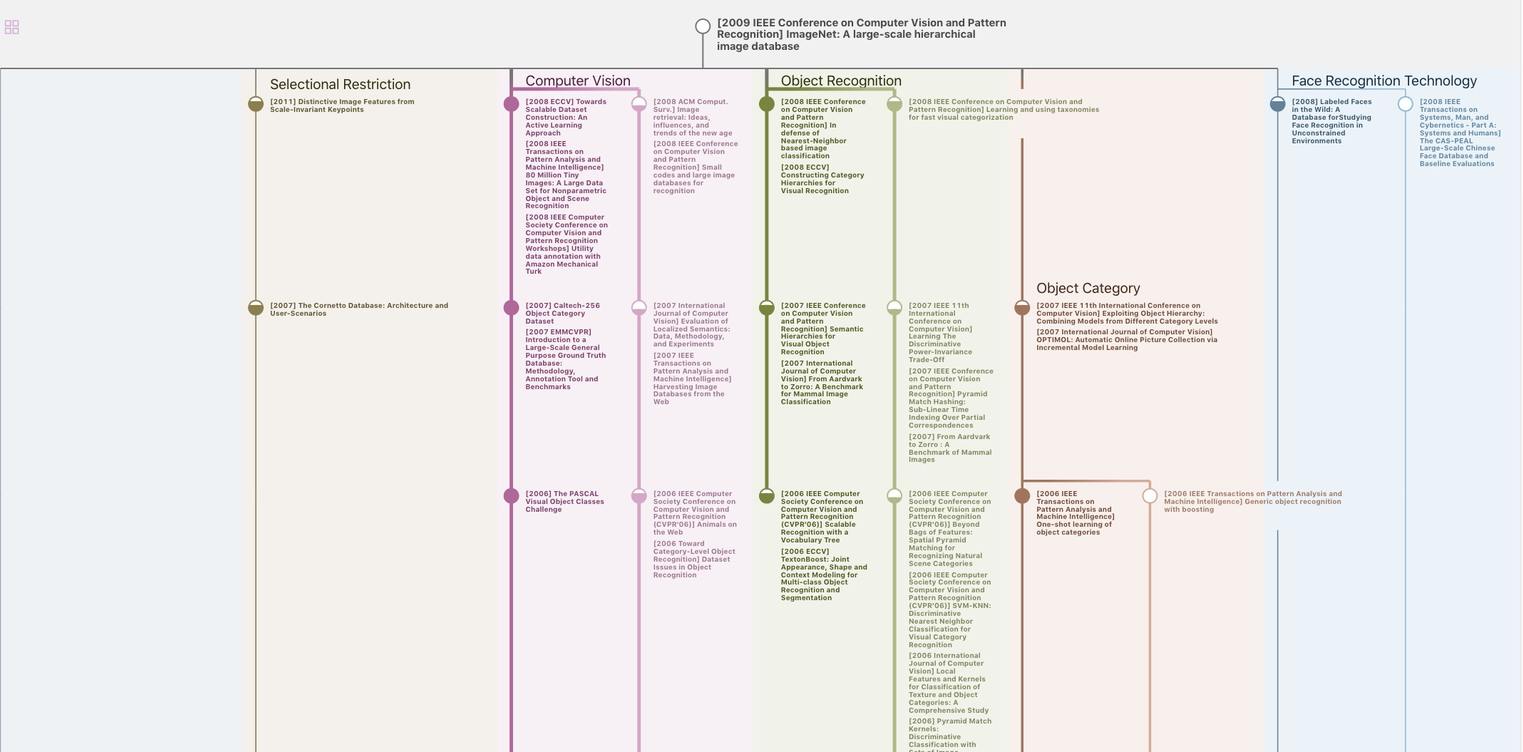
生成溯源树,研究论文发展脉络
Chat Paper
正在生成论文摘要