Sequence-Based Word Embeddings for Effective Text Classification.
NLDB(2021)
摘要
In this work we present DiVe (Distance-based Vector Embedding), a new word embedding technique based on the Logistic Markov Embedding (LME). First, we generalize LME to consider different distance metrics and address existing scalability issues using negative sampling, thus making DiVe scalable for large datasets. In order to evaluate the quality of word embeddings produced by DiVe, we used them to train standard machine learning classifiers, with the goal of performing different Natural Language Processing (NLP) tasks. Our experiments demonstrated that DiVe is able to outperform existing (more complex) machine learning approaches, while preserving simplicity and scalability.
更多查看译文
关键词
Word embeedings,Logistic Markov embedding,NLP
AI 理解论文
溯源树
样例
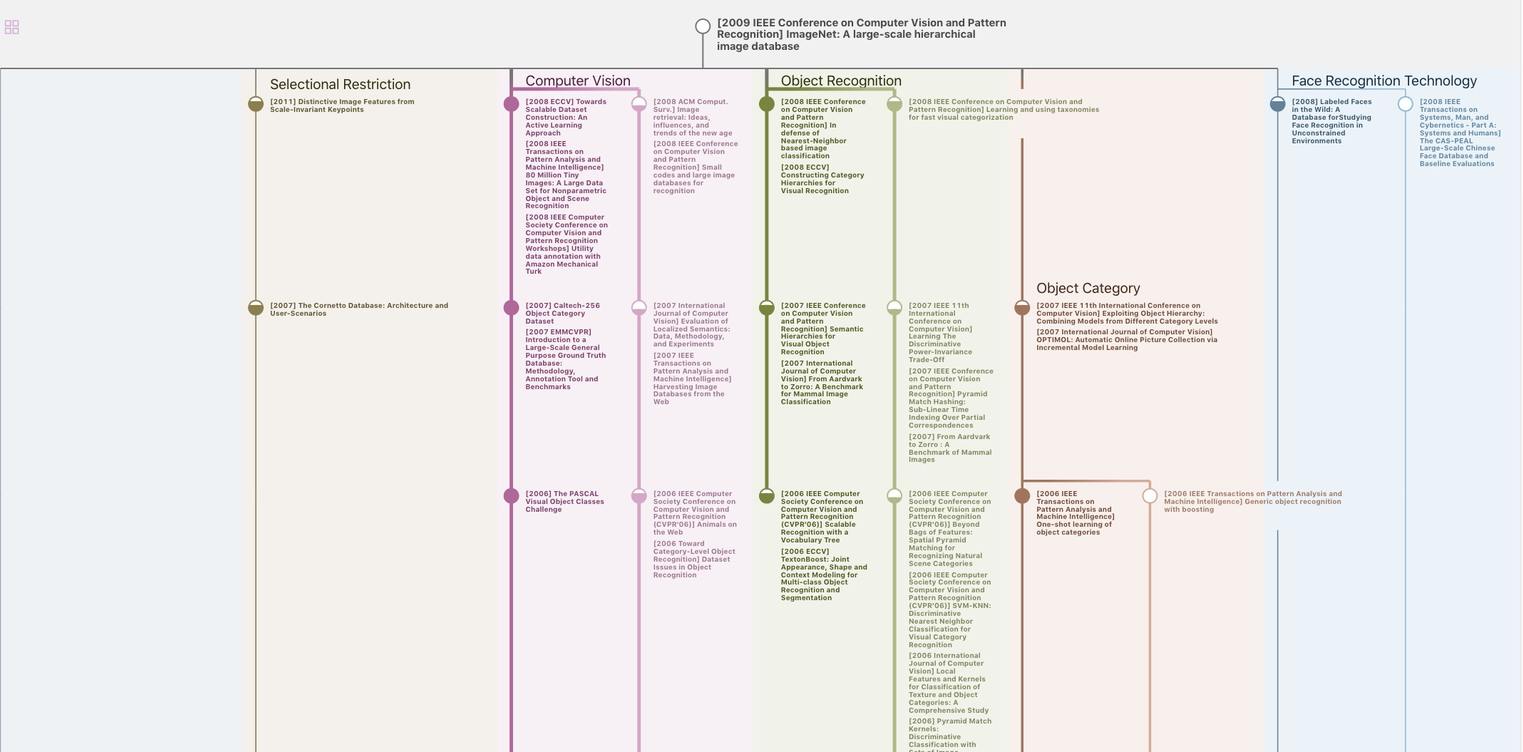
生成溯源树,研究论文发展脉络
Chat Paper
正在生成论文摘要