On minimizing cost in legal document review workflows
DOCENG(2021)
摘要
ABSTRACTTechnology-assisted review (TAR) refers to human-in-the-loop machine learning workflows for document review in legal discovery and other high recall review tasks. Attorneys and legal technologists have debated whether review should be a single iterative process (one-phase TAR workflows) or whether model training and review should be separate (two-phase TAR workflows), with implications for the choice of active learning algorithm. The relative cost of manual labeling for different purposes (training vs. review) and of different documents (positive vs. negative examples) is a key and neglected factor in this debate. Using a novel cost dynamics analysis, we show analytically and empirically that these relative costs strongly impact whether a one-phase or two-phase workflow minimizes cost. We also show how category prevalence, classification task difficulty, and collection size impact the optimal choice not only of workflow type, but of active learning method and stopping point.
更多查看译文
关键词
cost modeling, active learning, total recall, high-recall retrieval
AI 理解论文
溯源树
样例
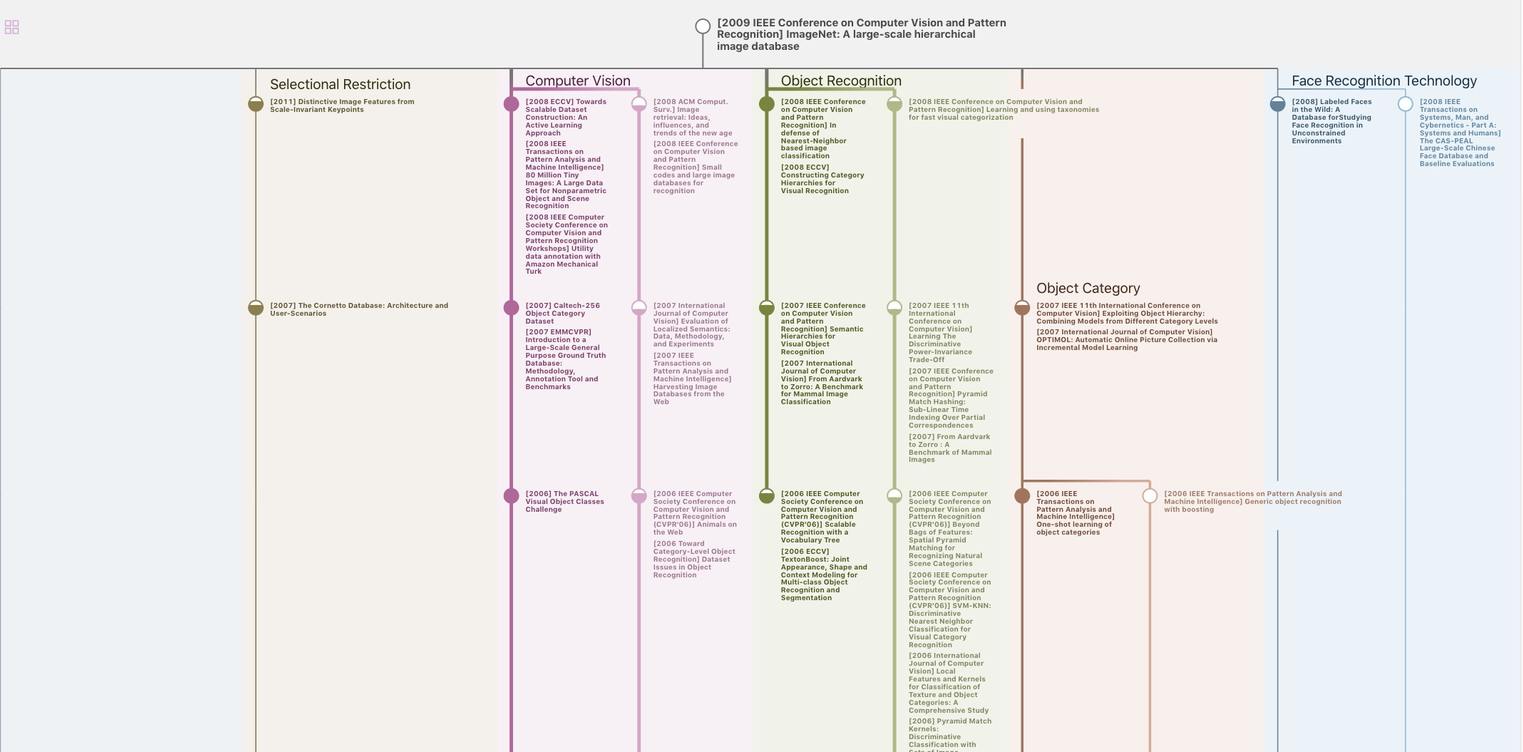
生成溯源树,研究论文发展脉络
Chat Paper
正在生成论文摘要