Towards Adversarial Robustness via Transductive Learning
arxiv(2021)
摘要
There has been emerging interest to use transductive learning for adversarial robustness (Goldwasser et al., NeurIPS 2020; Wu et al., ICML 2020). Compared to traditional "test-time" defenses, these defense mechanisms "dynamically retrain" the model based on test time input via transductive learning; and theoretically, attacking these defenses boils down to bilevel optimization, which seems to raise the difficulty for adaptive attacks. In this paper, we first formalize and analyze modeling aspects of transductive robustness. Then, we propose the principle of attacking model space for solving bilevel attack objectives, and present an instantiation of the principle which breaks previous transductive defenses. These attacks thus point to significant difficulties in the use of transductive learning to improve adversarial robustness. To this end, we present new theoretical and empirical evidence in support of the utility of transductive learning.
更多查看译文
关键词
adversarial robustness,learning
AI 理解论文
溯源树
样例
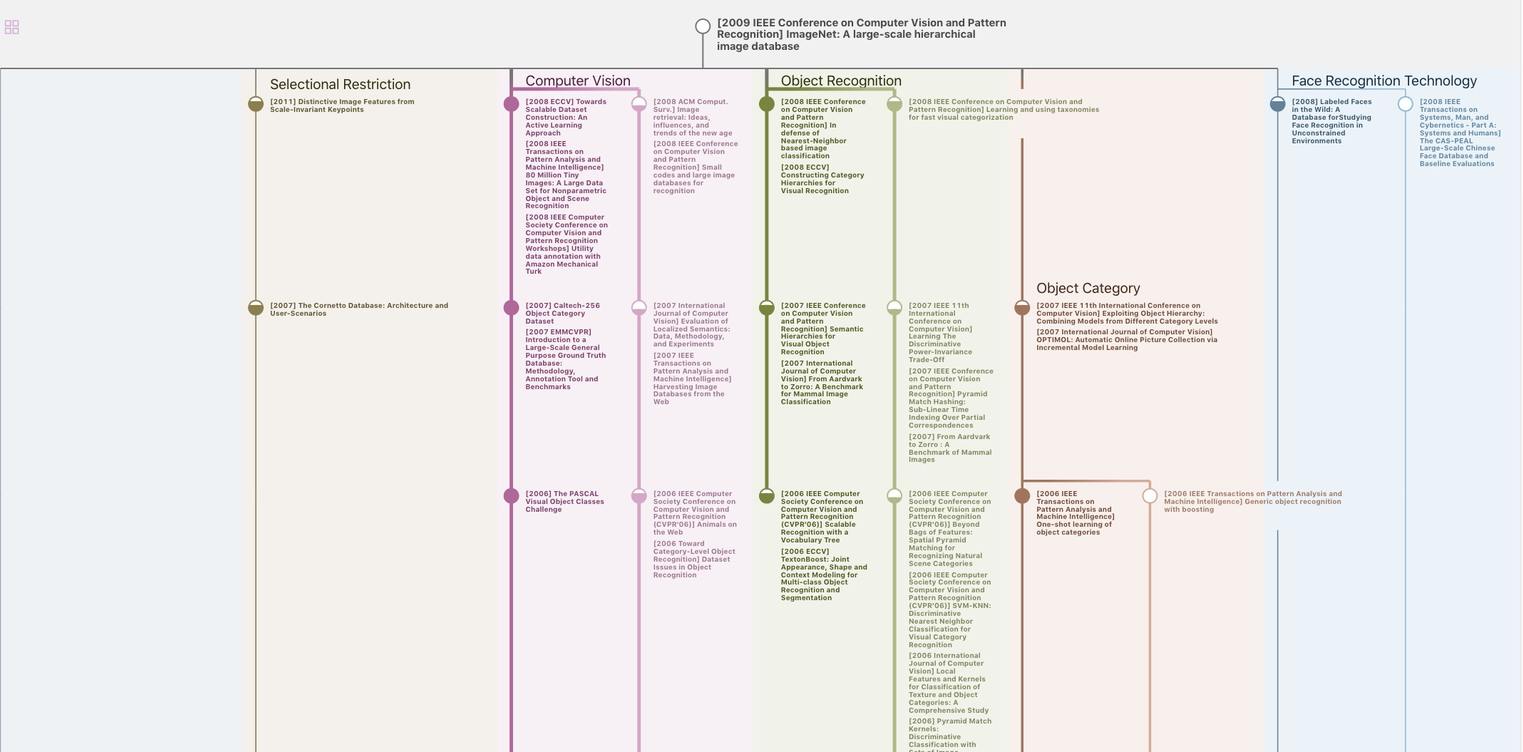
生成溯源树,研究论文发展脉络
Chat Paper
正在生成论文摘要