A Discriminative Technique For Multiple-Source Adaptation
INTERNATIONAL CONFERENCE ON MACHINE LEARNING, VOL 139(2021)
摘要
We present a new discriminative technique for the multiple-source adaptation (MSA) problem. Unlike previous work, which relies on density estimation for each source domain, our solution only requires conditional probabilities that can be straightforwardly accurately estimated from unlabeled data from the source domains. We give a detailed analysis of our new technique, including general guarantees based on Renyi divergences, and learning bounds when conditional Maxent is used for estimating conditional probabilities for a point to belong to a source domain. We show that these guarantees compare favorably to those that can be derived for the generative solution, using kernel density estimation. Our experiments with real-world applications further demonstrate that our new discriminative MSA algorithm outperforms the previous generative solution as well as other domain adaptation baselines.
更多查看译文
关键词
discriminative technique,multiple-source
AI 理解论文
溯源树
样例
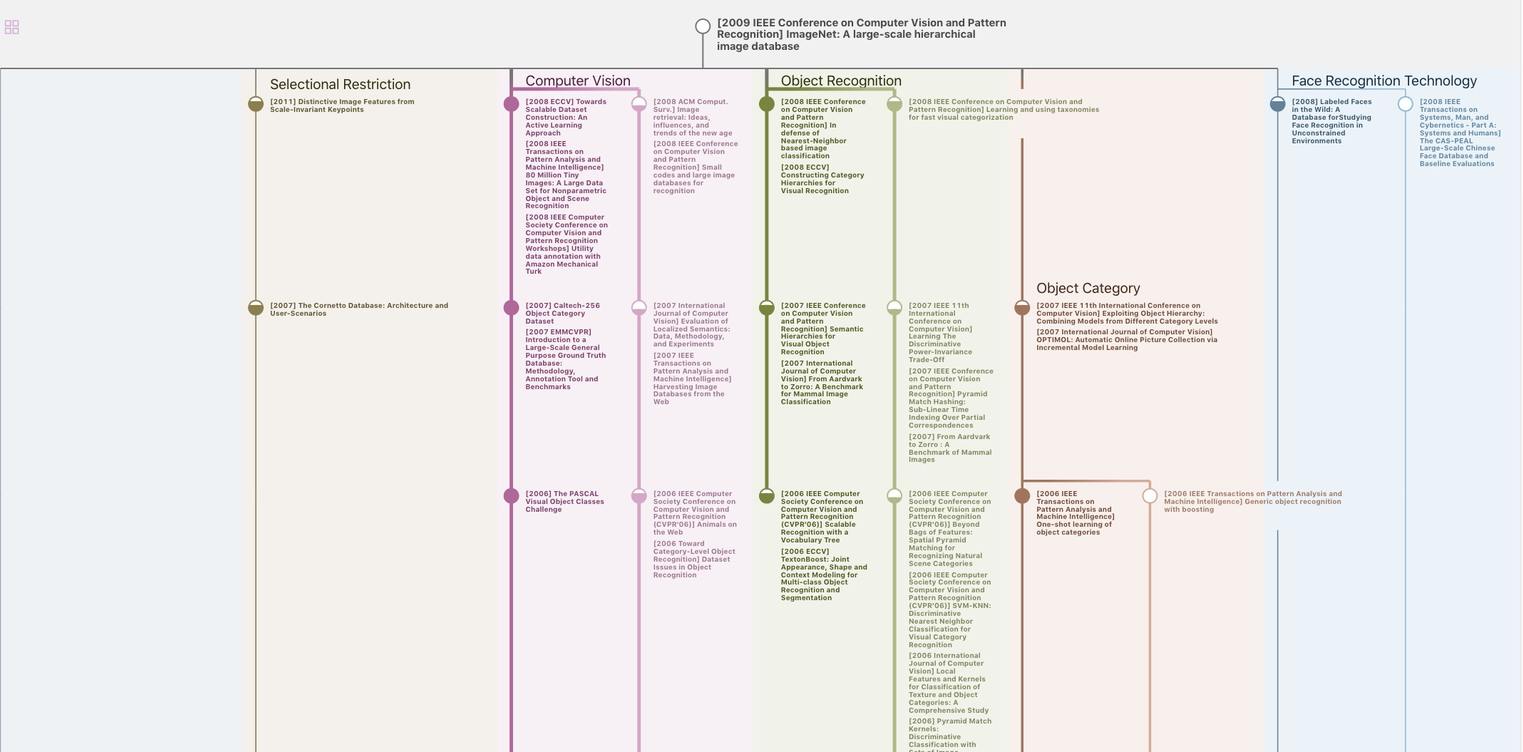
生成溯源树,研究论文发展脉络
Chat Paper
正在生成论文摘要