Propensity-scored Probabilistic Label Trees
Research and Development in Information Retrieval(2021)
摘要
ABSTRACTExtreme multi-label classification (XMLC) refers to the task of tagging instances with small subsets of relevant labels coming from an extremely large set of all possible labels. Recently, XMLC has been widely applied to diverse web applications such as automatic content labeling, online advertising, or recommendation systems. In such environments, label distribution is often highly imbalanced, consisting mostly of very rare tail labels, and relevant labels can be missing. As a remedy to these problems, the propensity model has been introduced and applied within several XMLC algorithms. In this work, we focus on the problem of optimal predictions under this model for probabilistic label trees, a popular approach for XMLC problems. We introduce an inference procedure, based on the A*-search algorithm, that efficiently finds the optimal solution, assuming that all probabilities and propensities are known. We demonstrate the attractiveness of this approach in a wide empirical study on popular XMLC benchmark datasets.
更多查看译文
关键词
extreme classification, multi-label classification, propensity model, missing labels, probabilistic label trees, supervised learning, recommendation, tagging, ranking
AI 理解论文
溯源树
样例
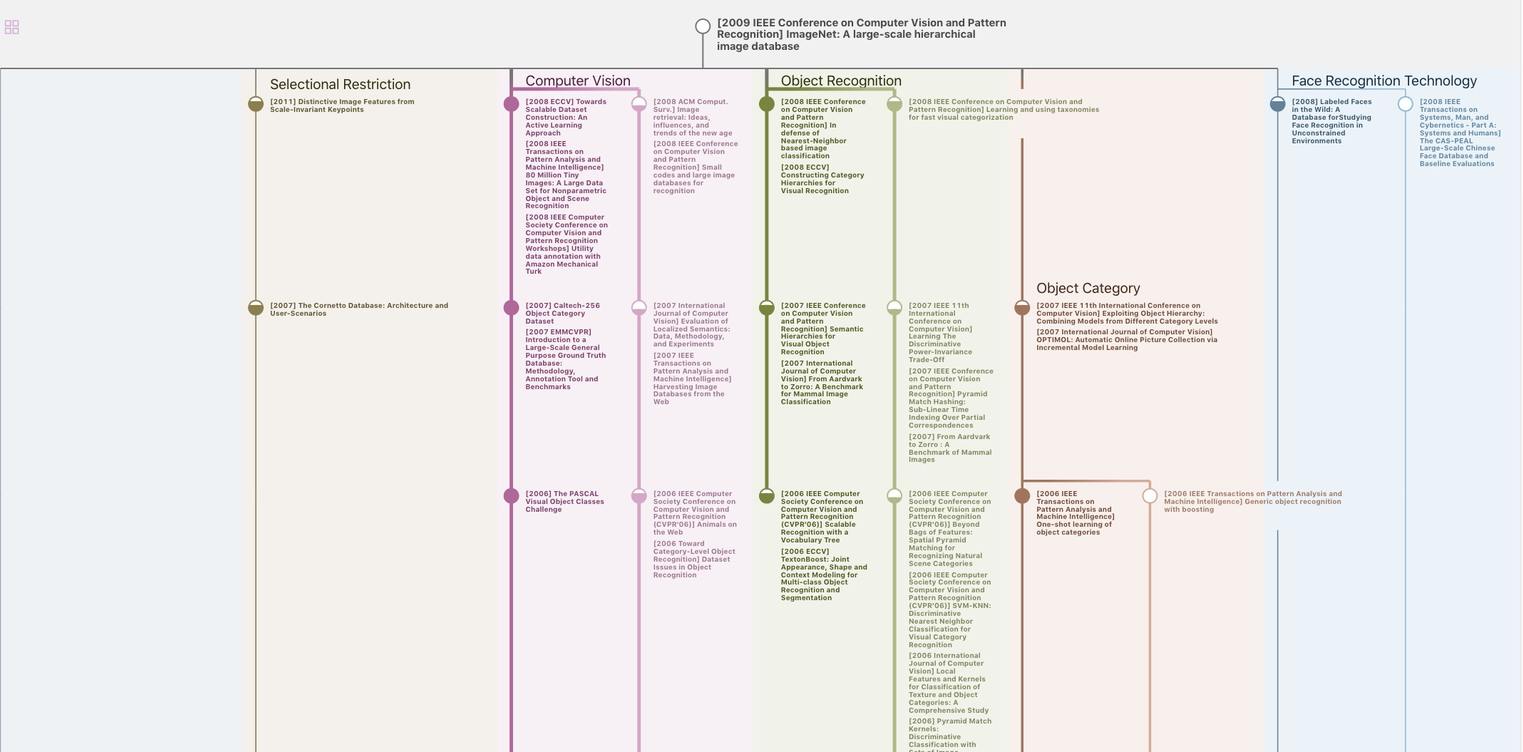
生成溯源树,研究论文发展脉络
Chat Paper
正在生成论文摘要