Faster first-order primal-dual methods for linear programming using restarts and sharpness
arxiv(2022)
摘要
First-order primal-dual methods are appealing for their low memory overhead, fast iterations, and effective parallelization. However, they are often slow at finding high accuracy solutions, which creates a barrier to their use in traditional linear programming (LP) applications. This paper exploits the sharpness of primal-dual formulations of LP instances to achieve linear convergence using restarts in a general setting that applies to alternating direction method of multipliers (ADMM), primal-dual hybrid gradient method (PDHG) and extragradient method (EGM). In the special case of PDHG, without restarts we show an iteration count lower bound of Ω (κ ^2 log (1/ϵ )) , while with restarts we show an iteration count upper bound of O(κlog (1/ϵ )) , where κ is a condition number and ϵ is the desired accuracy. Moreover, the upper bound is optimal for a wide class of primal-dual methods, and applies to the strictly more general class of sharp primal-dual problems. We develop an adaptive restart scheme and verify that restarts significantly improve the ability of PDHG, EGM, and ADMM to find high accuracy solutions to LP problems.
更多查看译文
关键词
90C05 (linear programming), 90C47 (minimax problems)
AI 理解论文
溯源树
样例
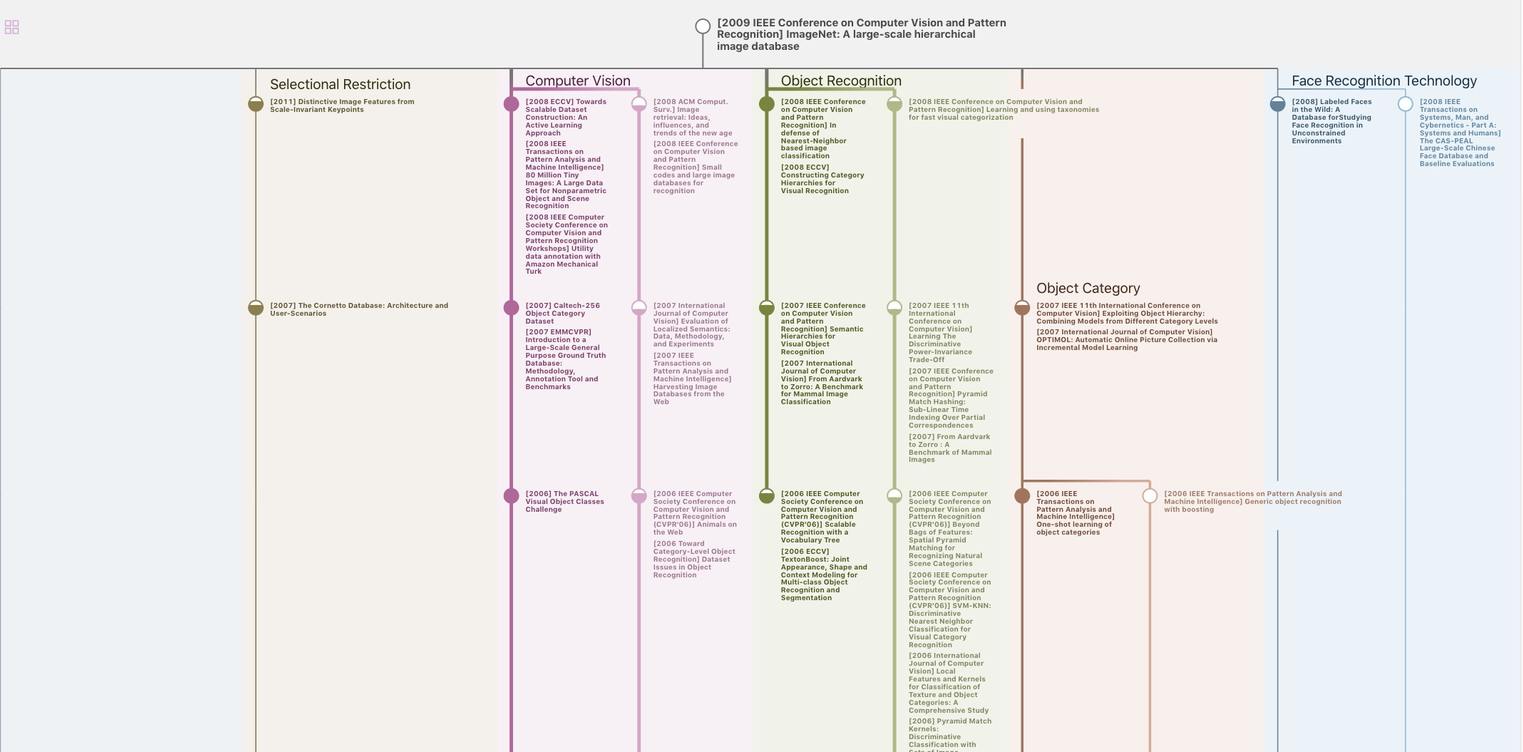
生成溯源树,研究论文发展脉络
Chat Paper
正在生成论文摘要